Multi-Agent Training beyond Zero-Sum with Correlated Equilibrium Meta-Solvers
INTERNATIONAL CONFERENCE ON MACHINE LEARNING, VOL 139(2021)
摘要
Two-player, constant-sum games are well studied in the literature, but there has been limited progress outside of this setting. We propose Joint Policy-Space Response Oracles (JPSRO), an algorithm for training agents in n-player, general-sum extensive form games, which provably converges to an equilibrium. We further suggest correlated equilibria (CE) as promising meta-solvers, and propose a novel solution concept Maximum Gini Correlated Equilibrium (MGCE), a principled and computationally efficient family of solutions for solving the correlated equilibrium selection problem. We conduct several experiments using CE meta-solvers for JPSRO and demonstrate convergence on n-player, general-sum games.
更多查看译文
关键词
correlated equilibrium
AI 理解论文
溯源树
样例
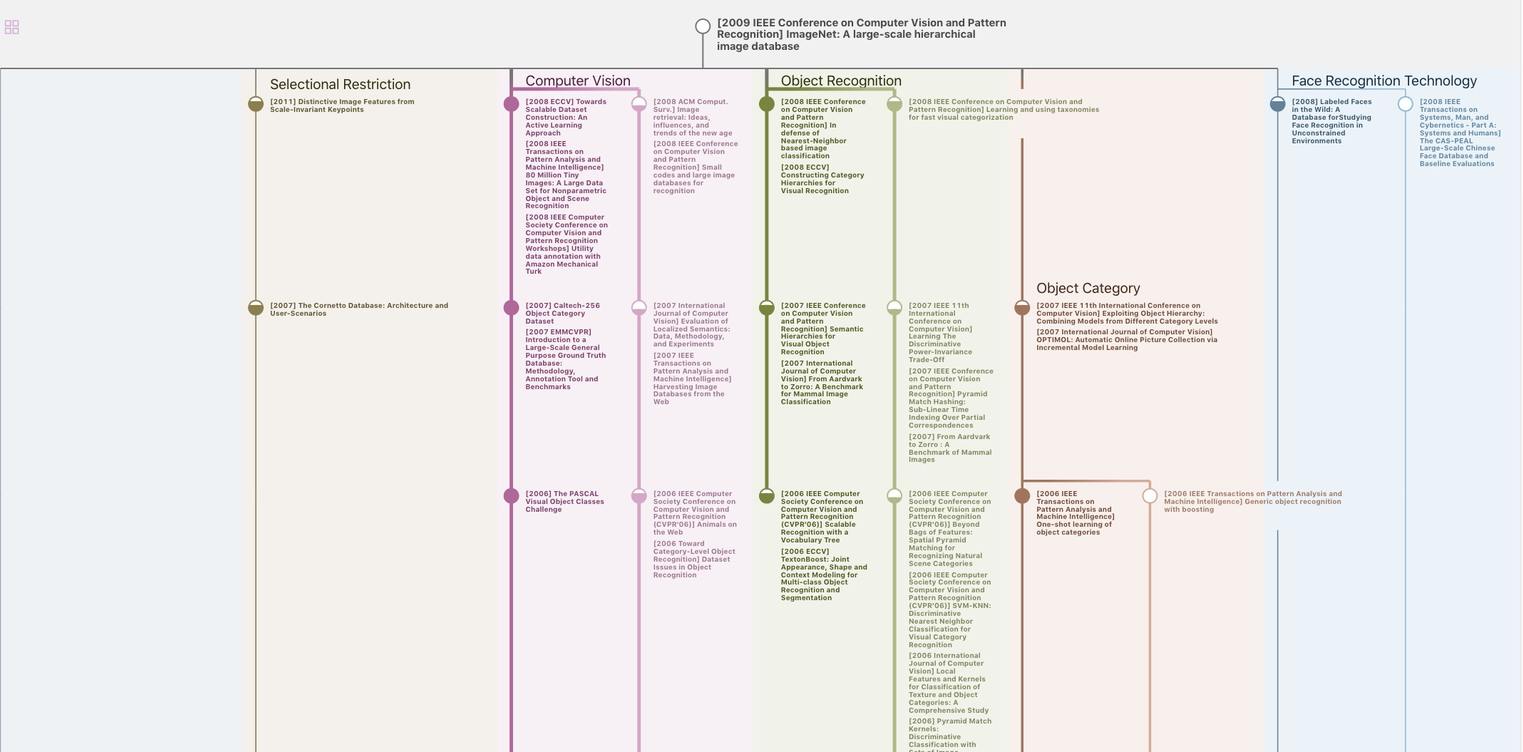
生成溯源树,研究论文发展脉络
Chat Paper
正在生成论文摘要