Grounding Language To Entities And Dynamics For Generalization In Reinforcement Learning
INTERNATIONAL CONFERENCE ON MACHINE LEARNING, VOL 139(2021)
摘要
We investigate the use of natural language to drive the generalization of control policies and introduce the new multi-task environment MESSENGER with free-form text manuals describing the environment dynamics. Unlike previous work, MESSENGER does not assume prior knowledge connecting text and state observations - the control policy must simultaneously ground the game manual to entity symbols and dynamics in the environment. We develop a new model, EMMA (Entity Mapper with Multi-modal Attention) which uses an entity-conditioned attention module that allows for selective focus over relevant descriptions in the manual for each entity in the environment. EMMA is end-to-end differentiable and learns a latent grounding of entities and dynamics from text to observations using only environment rewards. EMMA achieves successful zero-shot generalization to unseen games with new dynamics, obtaining a 40% higher win rate compared to multiple baselines. However, win rate on the hardest stage of MESSENGER remains low (10%), demonstrating the need for additional work in this direction.
更多查看译文
关键词
generalization,reinforcement,grounding,entities,learning
AI 理解论文
溯源树
样例
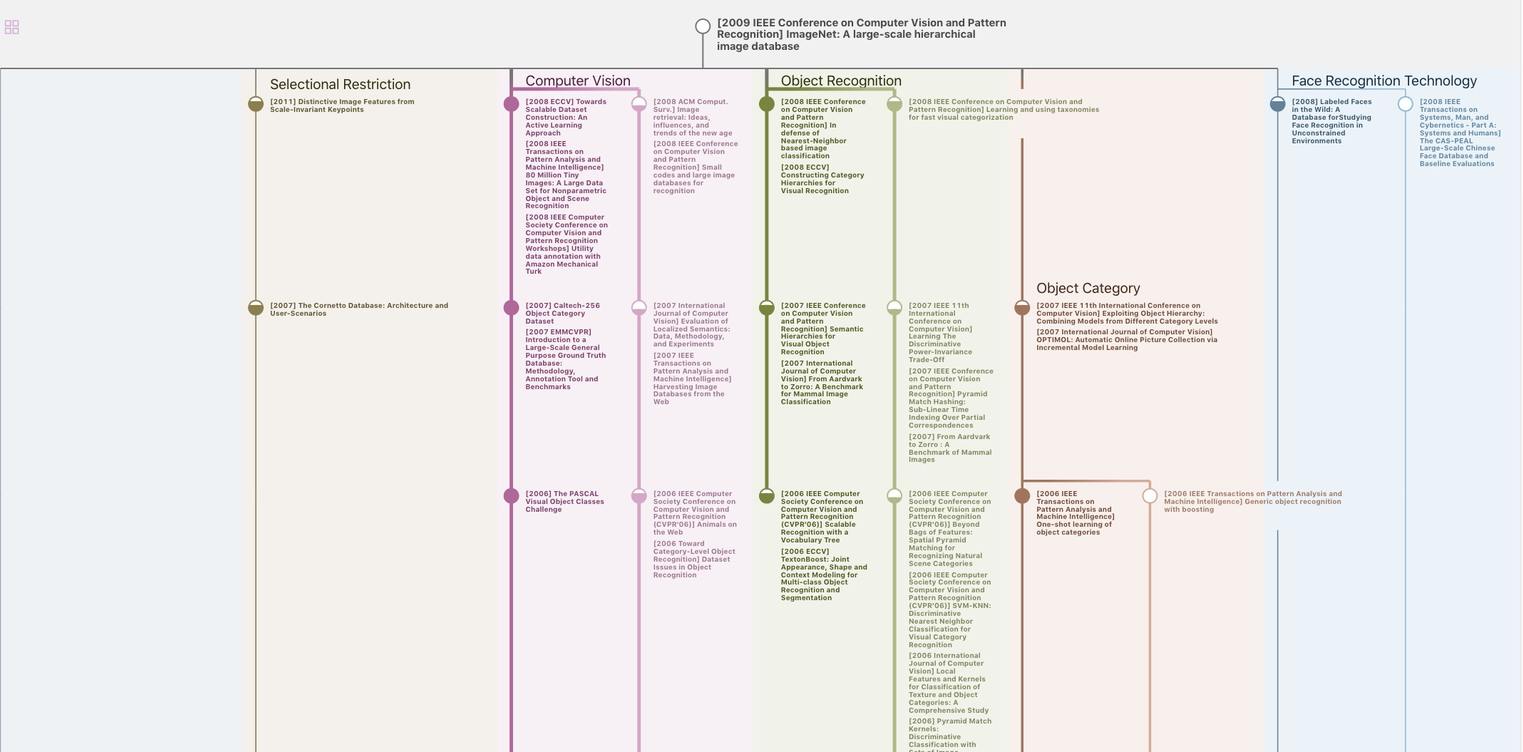
生成溯源树,研究论文发展脉络
Chat Paper
正在生成论文摘要