Reward Identification In Inverse Reinforcement Learning
INTERNATIONAL CONFERENCE ON MACHINE LEARNING, VOL 139(2021)
摘要
We study the problem of reward identifiability in the context of Inverse Reinforcement Learning (IRL). The reward identifiability question is critical to answer when reasoning about the effectiveness of using Markov Decision Processes (MDPs) as computational models of real world decision makers in order to understand complex decision making behavior and perform counterfactual reasoning. While identifiability has been acknowledged as a fundamental theoretical question in IRL, little is known about the types of MDPs for which rewards are identifiable, or even if there exist such MDPs. In this work, we formalize the reward identification problem in IRL and study how identifiability relates to properties of the MDP model. For deterministic MDP models with the MaxEntRL objective, we prove necessary and sufficient conditions for identifiability. Building on these results, we present efficient algorithms for testing whether or not an MDP model is identifiable.
更多查看译文
关键词
identification,learning
AI 理解论文
溯源树
样例
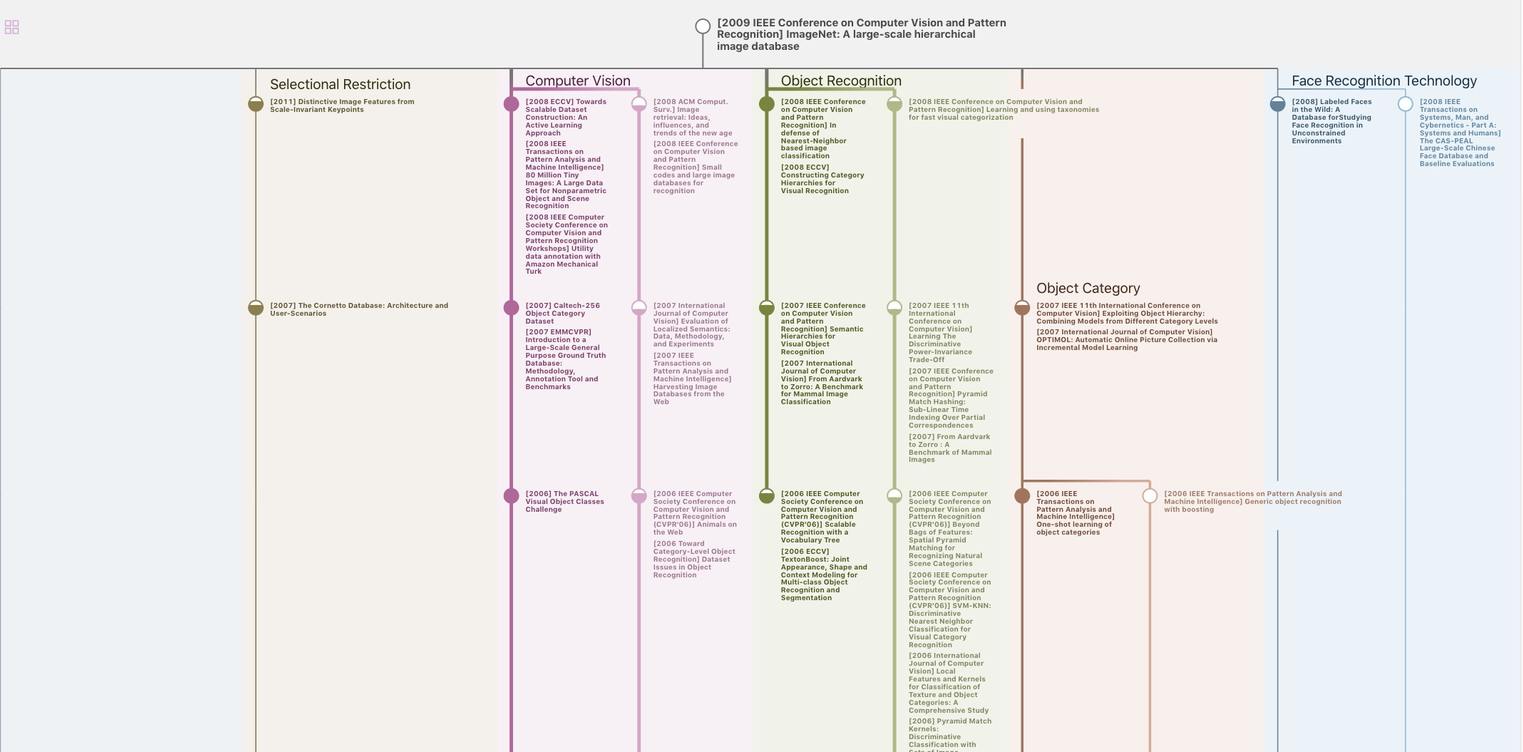
生成溯源树,研究论文发展脉络
Chat Paper
正在生成论文摘要