A Statistical Perspective on Distillation
INTERNATIONAL CONFERENCE ON MACHINE LEARNING, VOL 139(2021)
摘要
Knowledge distillation is a technique for improving a "student" model by replacing its one-hot training labels with a label distribution obtained from a "teacher" model. Despite its broad success, several basic questions - e.g., Why does distillation help? Why do more accurate teachers not necessarily distill better? - have received limited formal study. In this paper, we present a statistical perspective on distillation which sheds light on these questions. Our core observation is that a "Bayes teacher" providing the true class-probabilities can lower the variance of the student objective, and thus improve performance. We then establish a bias-variance tradeoff that quantifies the utility of teachers that approximate the Bayes class-probabilities. This provides a formal criterion as to what constitutes a "good" teacher, namely, the quality of its probability estimates. Finally, we illustrate how our statistical perspective facilitates novel applications of distillation to bipartite ranking and multiclass retrieval.
更多查看译文
AI 理解论文
溯源树
样例
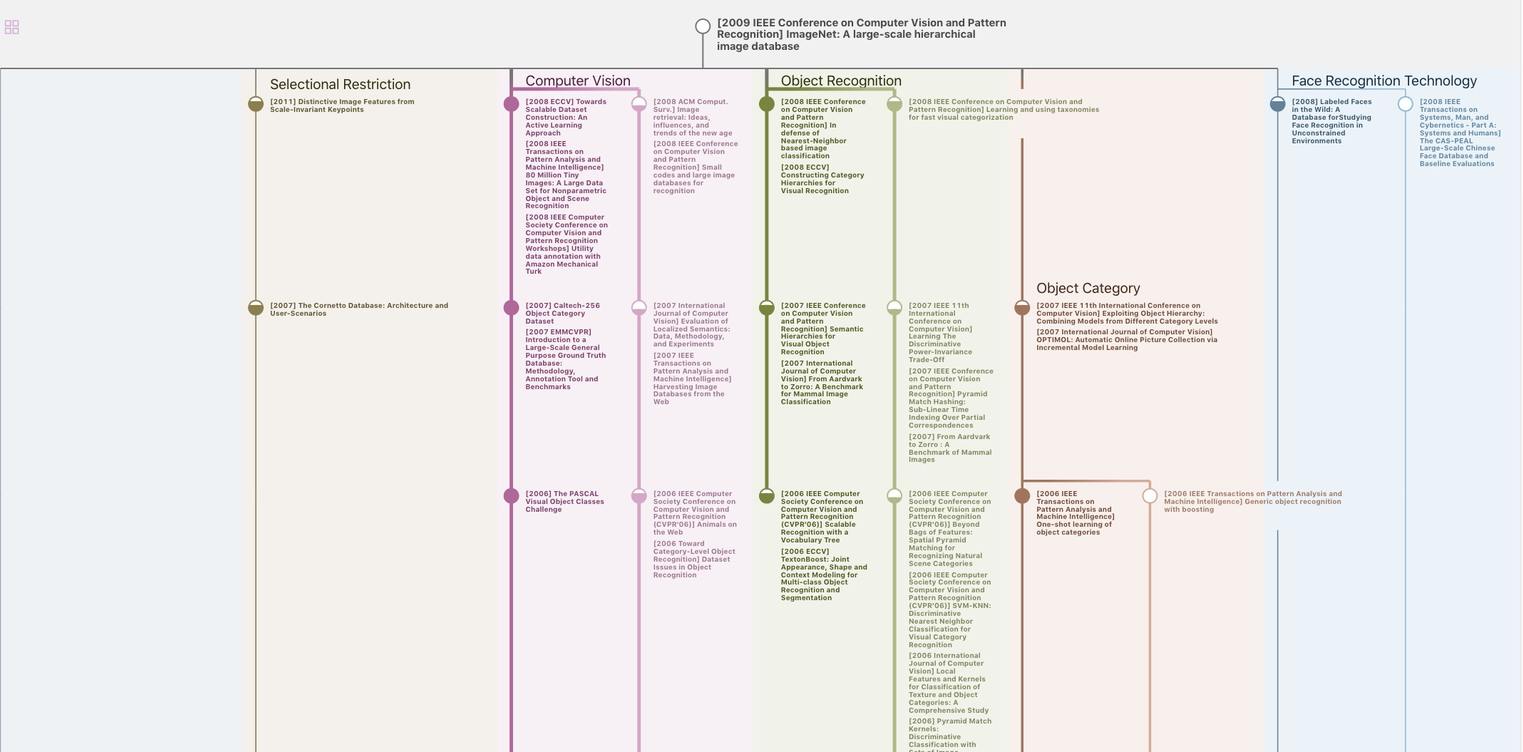
生成溯源树,研究论文发展脉络
Chat Paper
正在生成论文摘要