Development And Validation Of A Nomogram For Predicting Stroke Risk In Rheumatoid Arthritis Patients
AGING-US(2021)
Abstract
We developed and validated a nomogram to predict the risk of stroke in patients with rheumatoid arthritis (RA) in northern China. Out of six machine learning algorithms studied to improve diagnostic and prognostic accuracy of the prediction model, the logistic regression algorithm showed high performance in terms of calibration and decision curve analysis. The nomogram included stratifications of sex, age, systolic blood pressure, C-reactive protein, erythrocyte sedimentation rate, total cholesterol, and low-density lipoprotein cholesterol along with the history of traditional risk factors such as hypertensive, diabetes, atrial fibrillation, and coronary heart disease. The nomogram exhibited a high Hosmer-Lemeshow goodness-for-fit and good calibration (P > 0.05). The analysis, including the area under the receiver operating characteristic curve, the net reclassification index, the integrated discrimination improvement, and clinical use, showed that our prediction model was more accurate than the Framingham risk model in predicting stroke risk in RA patients. In conclusion, the nomogram can be used for individualized preoperative prediction of stroke risk in RA patients.
MoreTranslated text
Key words
rheumatoid arthritis, stroke, lipids, inflammatory markers, development and validation nomogram
AI Read Science
Must-Reading Tree
Example
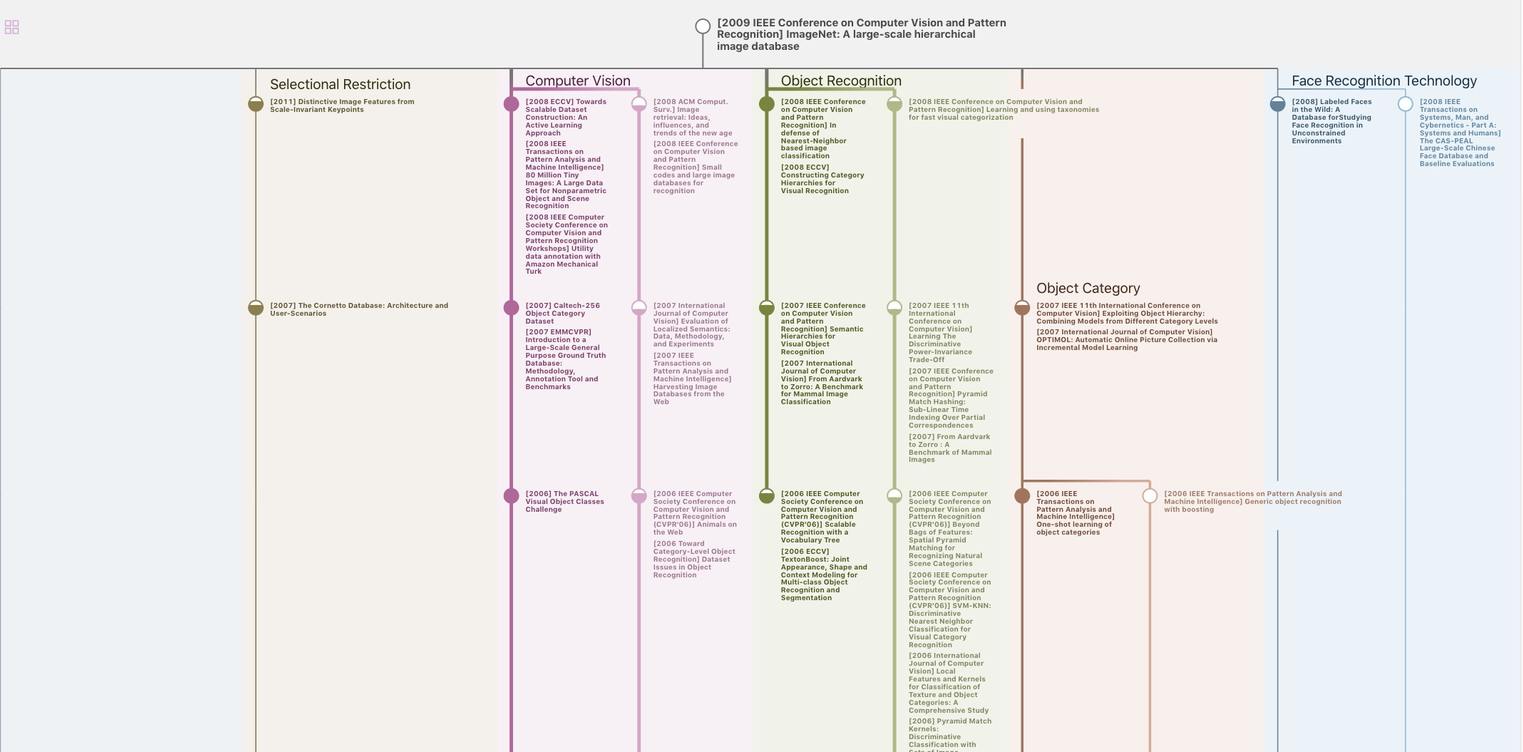
Generate MRT to find the research sequence of this paper
Chat Paper
Summary is being generated by the instructions you defined