Defending against Backdoor Attacks in Natural Language Generation
AAAI 2023(2023)
Abstract
The frustratingly fragile nature of neural network models make current natural language generation (NLG) systems prone to backdoor attacks and generate malicious sequences that could be sexist or offensive. Unfortunately, little effort has been invested to how backdoor attacks can affect current NLG models and how to defend against these attacks. In this work, by giving a formal definition of backdoor attack and defense, we investigate this problem on two important NLG tasks, machine translation and dialog generation. Tailored to the inherent nature of NLG models (e.g., producing a sequence of coherent words given contexts), we design defending strategies against attacks.
We find that testing the backward probability of generating sources given targets yields effective defense performance against all different types of attacks, and is able to handle the one-to-many issue in many NLG tasks such as dialog generation. We hope that this work can raise the awareness of backdoor risks concealed in deep NLG systems and inspire more future work (both attack and defense) towards this direction.
MoreTranslated text
Key words
APP: Security,SNLP: Bias, Fairness, Transparency & Privacy,SNLP: Adversarial Attacks & Robustness,SNLP: Generation
AI Read Science
Must-Reading Tree
Example
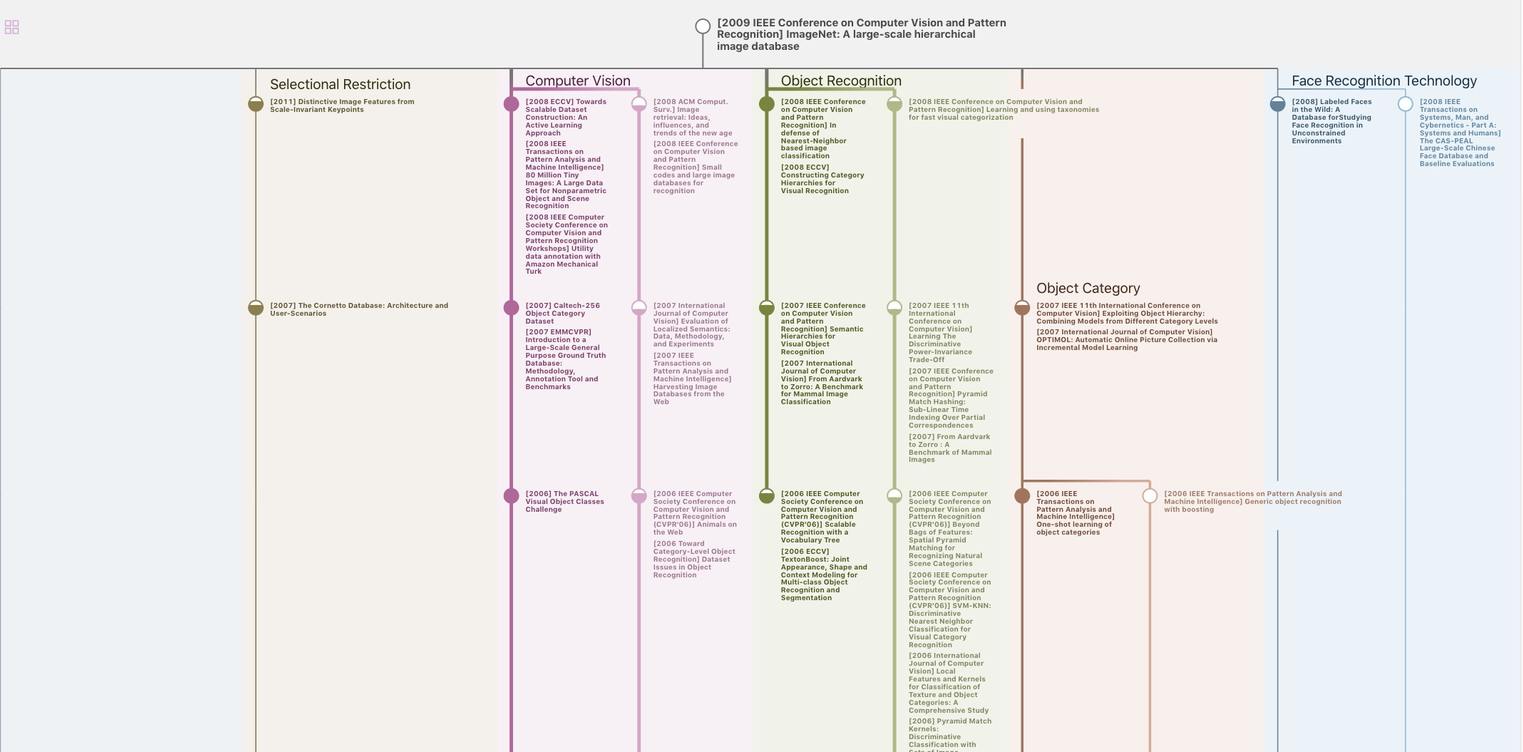
Generate MRT to find the research sequence of this paper
Chat Paper
Summary is being generated by the instructions you defined