Local Anomaly Detection for Multivariate Time Series by Temporal Dependency Based on Poisson Model
IEEE Transactions on Neural Networks and Learning Systems(2022)
摘要
Multivariate time series data are invasive in different domains, ranging from data center supervision and e-commerce data to financial transactions. This kind of data presents an important challenge for anomaly detection due to the temporal dependency aspect of its observations. In this article, we investigate the problem of unsupervised local anomaly detection in multivariate time series data from temporal modeling and residual analysis perspectives. The residual analysis has been shown to be effective in classical anomaly detection problems. However, it is a nontrivial task in multivariate time series as the temporal dependency between the time series observations complicates the residual modeling process. Methodologically, we propose a unified learning framework to characterize the residuals and their coherence with the temporal aspect of the whole multivariate time series. Experiments on real-world datasets are provided showing the effectiveness of the proposed algorithm.
更多查看译文
关键词
Knowledge and data engineering tools and techniques,machine learning,optimization,time series analysis
AI 理解论文
溯源树
样例
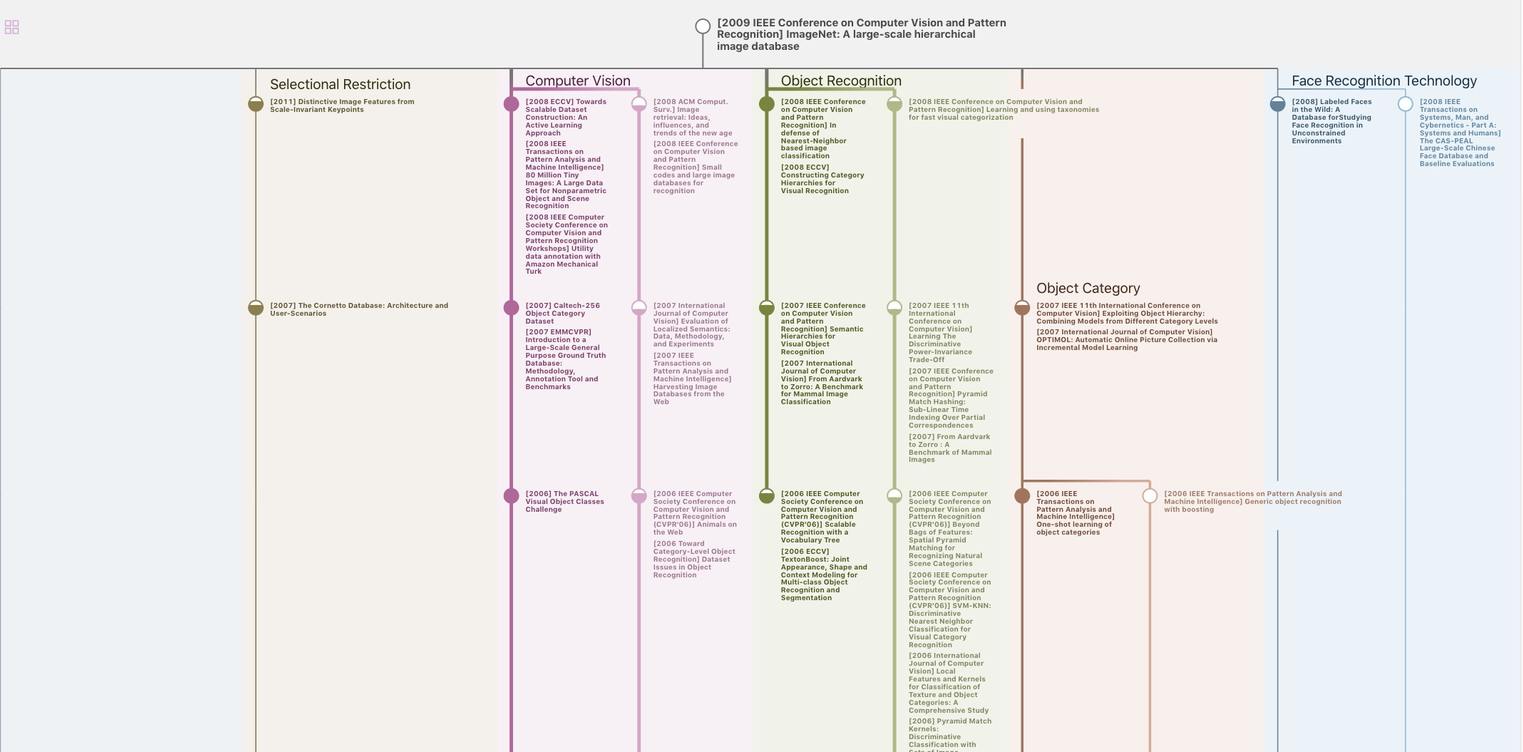
生成溯源树,研究论文发展脉络
Chat Paper
正在生成论文摘要