A differentially private location generalization approach to guarantee non-uniform privacy in moving objects databases
Knowledge-Based Systems(2021)
摘要
Recently there has been much interest in moving objects databases because of their applications in many domains, such as location-based services and traffic management. Moving objects databases store and manage information representing changes in the spatial properties of moving objects over time. Meanwhile, privacy protection has been one of the most important concerns in these databases. In this paper, we study this problem by presenting DPLG, a location generalization approach for moving objects databases that preserves the strong guarantee of differential privacy. Our main goal is to guarantee non-uniform privacy for locations with different privacy protection requirements while being scalable for spatial domains with a large number of locations. For this purpose, we use location generalization in such a way that locations with higher privacy protection requirements are generalized to larger ones. Location generalization also has the advantage that it enables DPLG to reduce the number of locations and, thus, to keep the running time and space requirements as reasonable as possible. We also present two post-processing techniques, namely, consistency constraints enforcement and quality improvement, to have consistent query answers and to reduce query errors caused by location generalization. The quality improvement technique divides the noisy count of each generalized location among the reference locations it contains homogeneously or heterogeneously. Extensive experiments demonstrate that, in addition to keeping reasonable the running time and space requirements, DPLG improves the utility of query answers for locations with lower privacy protection requirements in comparison to those with higher privacy protection requirements while satisfying differential privacy.
更多查看译文
关键词
Moving objects database,Trajectory,Differentially private location generalization,Differential privacy,Non-uniform privacy,Quality improvement
AI 理解论文
溯源树
样例
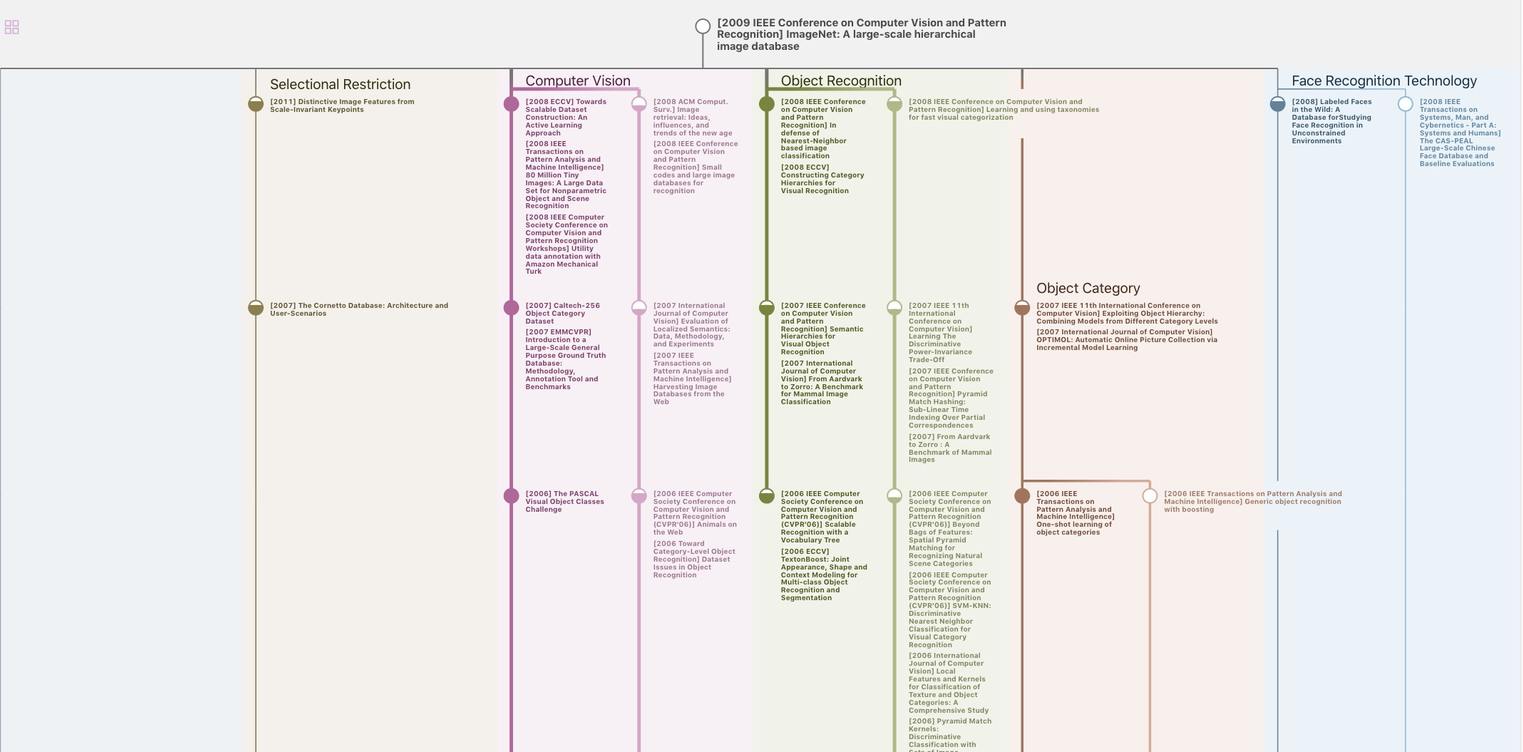
生成溯源树,研究论文发展脉络
Chat Paper
正在生成论文摘要