Dirac: Dynamic-Irregular Clustering Algorithm With Incremental Learning For Rf-Based Trust Augmentation In Iot Device Authentication
2021 IEEE INTERNATIONAL SYMPOSIUM ON CIRCUITS AND SYSTEMS (ISCAS)(2021)
摘要
Unlike traditional radio frequency device authentication which utilizes security keys in conjunction with a digital subsystem for verification, human voice communication involves probabilistic identification of a person based on his/her voice signatures and improves the detection probability over time. Inspired by voice-based human identification, we implement a novel method of augmenting trust during device detection and authentication, involving dynamic irregular clustering which exploits the unique nonidealities in IoT devices as physical signatures originated from Radio Frequency (RF) circuitry. The proposed method increases the confidence level of the classification as more data come in from a particular device, and is also able to detect new devices that do not fall into any of the previous clusters. Using 30 Xbee modules as transmitters, we show that our proposed method can detect a transmitter with > 95% sensitivity (similar to 100% with optimum parameters) using only 0.2 milliseconds of test data which makes it suitable for a very low latency communication system. Also, the incremental learning feature of the proposed method renders a gradual increase in sensitivity as more data are available from the transmitter end. The proposed method can provide an additional security layer in conjunction with the existing methods without adding any additional burden, which is extremely important for resource-limited asymmetric IoT nodes.
更多查看译文
关键词
Clustering, radio frequency, circuits and systems nonideality, voice-inspired, incremental learning, IoT, security, device authentication
AI 理解论文
溯源树
样例
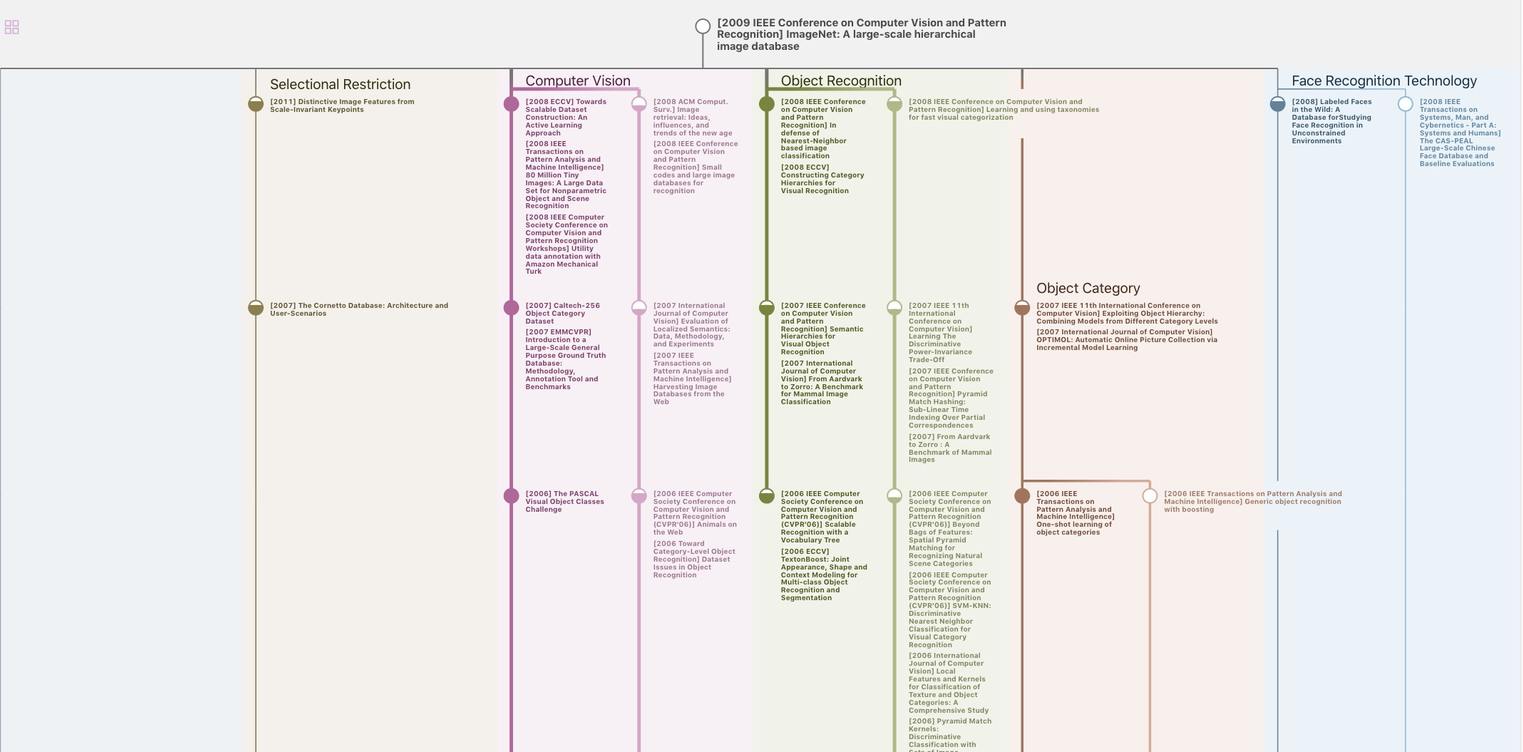
生成溯源树,研究论文发展脉络
Chat Paper
正在生成论文摘要