Data‐driven modeling of bridge buffeting in the time domain using long short‐term memory network based on structural health monitoring
Structural Control & Health Monitoring(2021)
摘要
A data-driven approach for modeling bridge buffeting in the time domain is proposed based on the structural health monitoring (SHM) system. The long short-term memory (LSTM) network is applied to model the bridge aerodynamic system with the potential fluid memory effect which is characterized by an uncertain time lag between inflow wind and the structural response. SHM is incorporated into this data-driven approach due to the advantages of prototype measurements such as the ability to consider the high Reynolds number effects and the real natural winds with nonuniformity and nonstationarity. The cell state in the LSTM module is applied to carry the potential fluid memory effects for predicting the aerodynamic response. We compare the obtained data-driven model and the conventional finite element model in the buffeting response prediction. The data-driven model shows higher accuracy than the conventional model, indicating that the proposed data-driven approach has promising potential in modeling bridge aerodynamics. The incorporation of the proposed LSTM-based bridge aerodynamic model and the field monitoring enables us to move buffeting predictions from lab theory to practical engineering.
更多查看译文
关键词
bridge aerodynamics, buffeting, data‐, driven approach, long short‐, term memory, long‐, span bridge, recurrent neural network, structural health monitoring
AI 理解论文
溯源树
样例
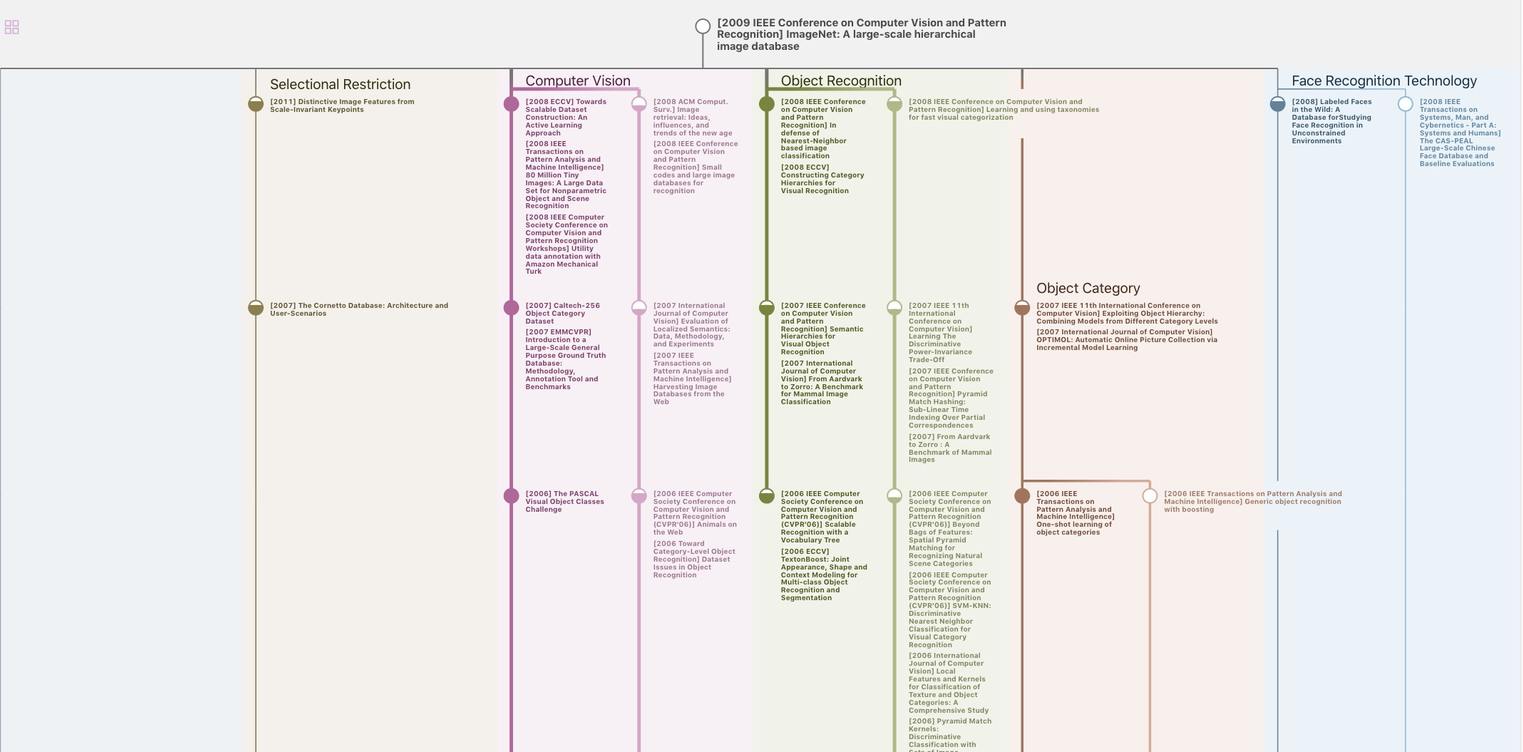
生成溯源树,研究论文发展脉络
Chat Paper
正在生成论文摘要