Automatic Segmentation To Characterize Anthropometric Parameters And Cardiovascular Indicators In Children
WILEY INTERDISCIPLINARY REVIEWS-DATA MINING AND KNOWLEDGE DISCOVERY(2021)
摘要
A new predictive model to classify childhood obesity was implemented using machine learning techniques. The first step was to calculate the most relevant anthropomorphic and cardiovascular parameters of 187 children through principal component analysis (PCA) and cluster classification. Then Naive-Bayes method classified these children into six groups using anthropometric Z Score, measurements of abdominal obesity, and arterial pressure: Group I (20.32% of total): composed mainly by accentuated malnutrition and malnutrition children; Group II (36.36%): composed primarily by eutrophic children; Group III (21.4%): constituted by eutrophic plus overweight children; Group IV (14.97%): comprised mainly by overweight and obese children; Group V (5.34%): Obese and overweight children; and Group VI (1.6%): obese at risk children. From Group II to VI, the proportion of pre-hypertensive and hypertensive children increased monotonically from 5 to 33%. This classification modes was tested on 66 children that were not originally included with a success rate of 97%. This predictive model will facilitate future longitudinal studies of obesity in children and will help plan interventions and evaluations of their results.This article is categorized under:Algorithmic Development > Biological Data Mining
更多查看译文
关键词
anthropometry, blood pressure, childhood obesity, cluster classification, Naï, ve‐, Bayes classifier, predictive childhood obesity classification, machine learning, automatic segmentation
AI 理解论文
溯源树
样例
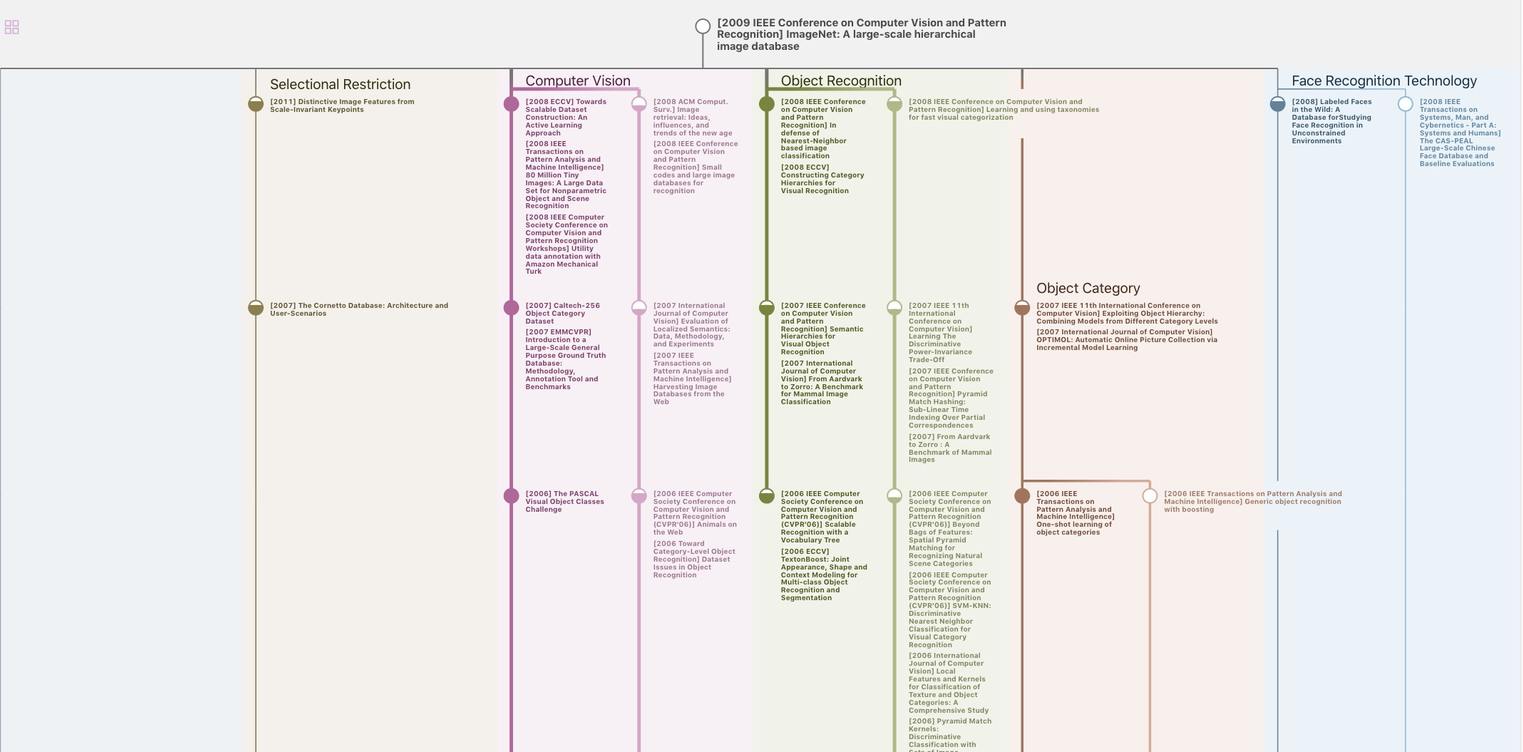
生成溯源树,研究论文发展脉络
Chat Paper
正在生成论文摘要