Compute-in-eDRAM with Backend Integrated Indium Gallium Zinc Oxide Transistors
2021 IEEE INTERNATIONAL SYMPOSIUM ON CIRCUITS AND SYSTEMS (ISCAS)(2021)
摘要
With rapid growth in data intensive applications, there is an ever-increasing need for energy efficient machine learning/AI hardware accelerators. The performance and the energy efficiency of such accelerators are primarily limited due of massive amount of data movement between processing engines and the off-chip memory. This memory wall bottleneck can be mitigated by performing accelerator specific computations in the memory (CIM) array embedded with the rest of the logic blocks. Multiple embedded memory technologies are being explored to advance CIM designs. Among these, embedded Dynamic Random Access Memory (eDRAM) using backend of the line (BEOL) integrated C-Axis Aligned Crystalline ( CAAC) Indium Gallium Zinc Oxide (IGZO) transistors is a promising candidate. IGZO transistor having extremely low leakage when used as an access transistor of the eDRAM bitcell can enable multi-level cell (MLC) eDRAM functionality. Moreover, higher bandwidth can be achieved by 3D stacking multiple layers of BEOL integrated IGZO devices in a monolithic manner improving the CIM performance. In this paper, we analyze various IGZO based eDRAM bitcell topologies and present an IGZO eDRAM CIM architecture. It supports 8-bit inputs/activations and 8-bit signed weights. 2-bit Flash Analog to Digital converter (ADC) is used for MLC weight bit read sensing. A representative neural network model using IGZO eDRAM and peripheral 8-b A/D converters based CIM design achieves 80% Top-1 inference accuracy for the CIFAR-10 dataset, which is within 3% of ideal software accuracy.
更多查看译文
关键词
Accelerators, Compute in memory, Embedded DRAM, Indium Gallium Zinc Oxide, Multi-level cell
AI 理解论文
溯源树
样例
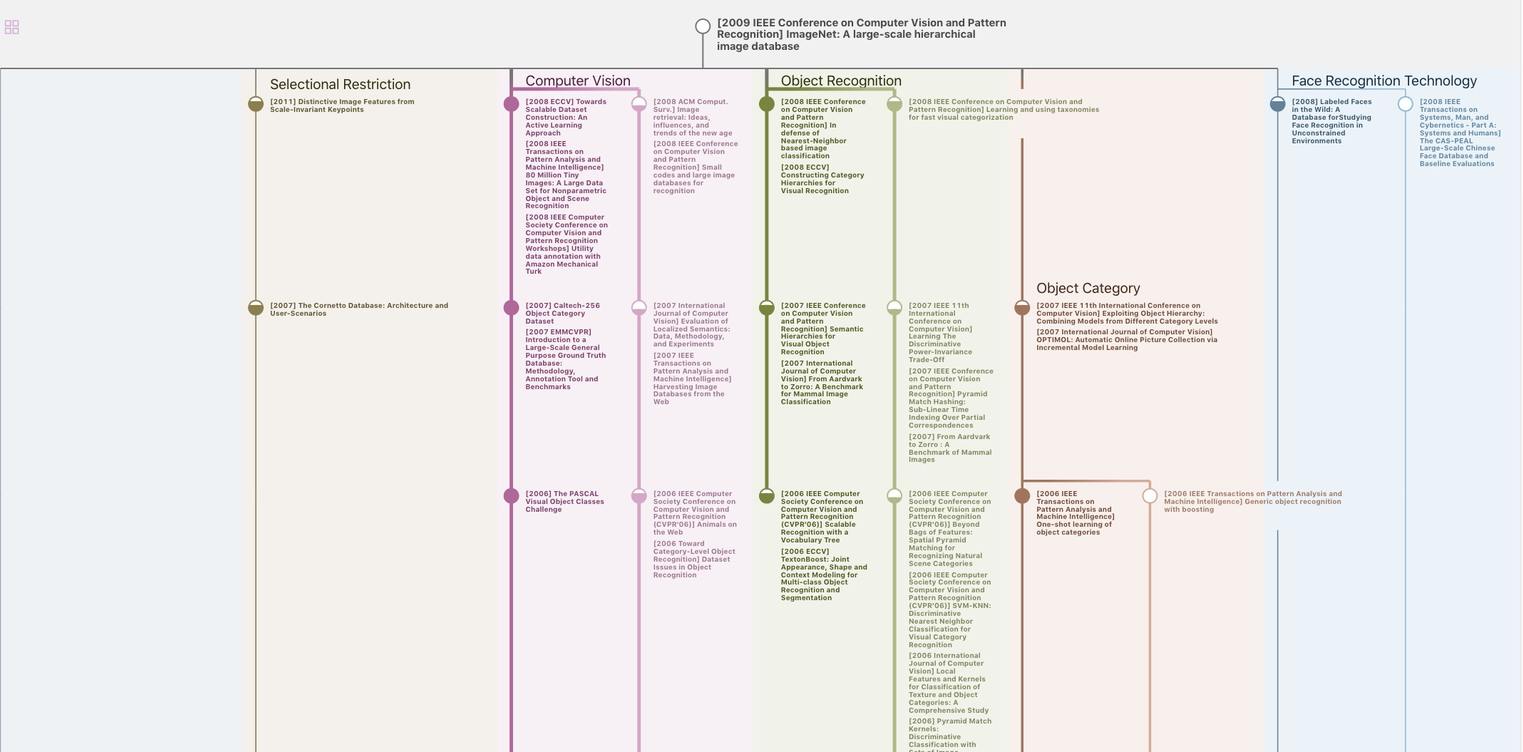
生成溯源树,研究论文发展脉络
Chat Paper
正在生成论文摘要