Gated Spatial Memory and Centroid-Aware Network for Building Instance Extraction
IEEE TRANSACTIONS ON GEOSCIENCE AND REMOTE SENSING(2022)
摘要
Automatic building extraction from high-resolution remote sensing images plays an important role in many application fields, such as the urban planning and photogrammetry. However, the complex background and large variety in building appearances in high-resolution remote sensing images make the building instance extraction challenging. In this study, we propose a novel two-stage instance segmentation network named gated spatial memory and centroid-aware network (GSMC) to handle these problems. Two new modules, including a gated spatial memory module (GSM) and a centroid-aware head (CH), are developed in our GSMC. The GSM is a top-down spatial structure and semantic information transmission module, where two gates including an input gate and a state gate are designed to strengthen the important features and replenish the lacking information. The CH is a new task head for regressing the geometric center of each instance, which can help to promote the accurate and complete recognition for irregularly shaped buildings. Experiments on the WHU Aerial data set, the WHU Satellite data set, and the Massachusetts Building data set demonstrate that the proposed GSMC can achieve consistently superior performances when compared with the recent state-of-the-art deep learning methods.
更多查看译文
关键词
Feature extraction, Buildings, Logic gates, Image segmentation, Semantics, Task analysis, Shape, Building extraction, centroid-aware head (CH), gating mechanism, instance segmentation, remote sensing image
AI 理解论文
溯源树
样例
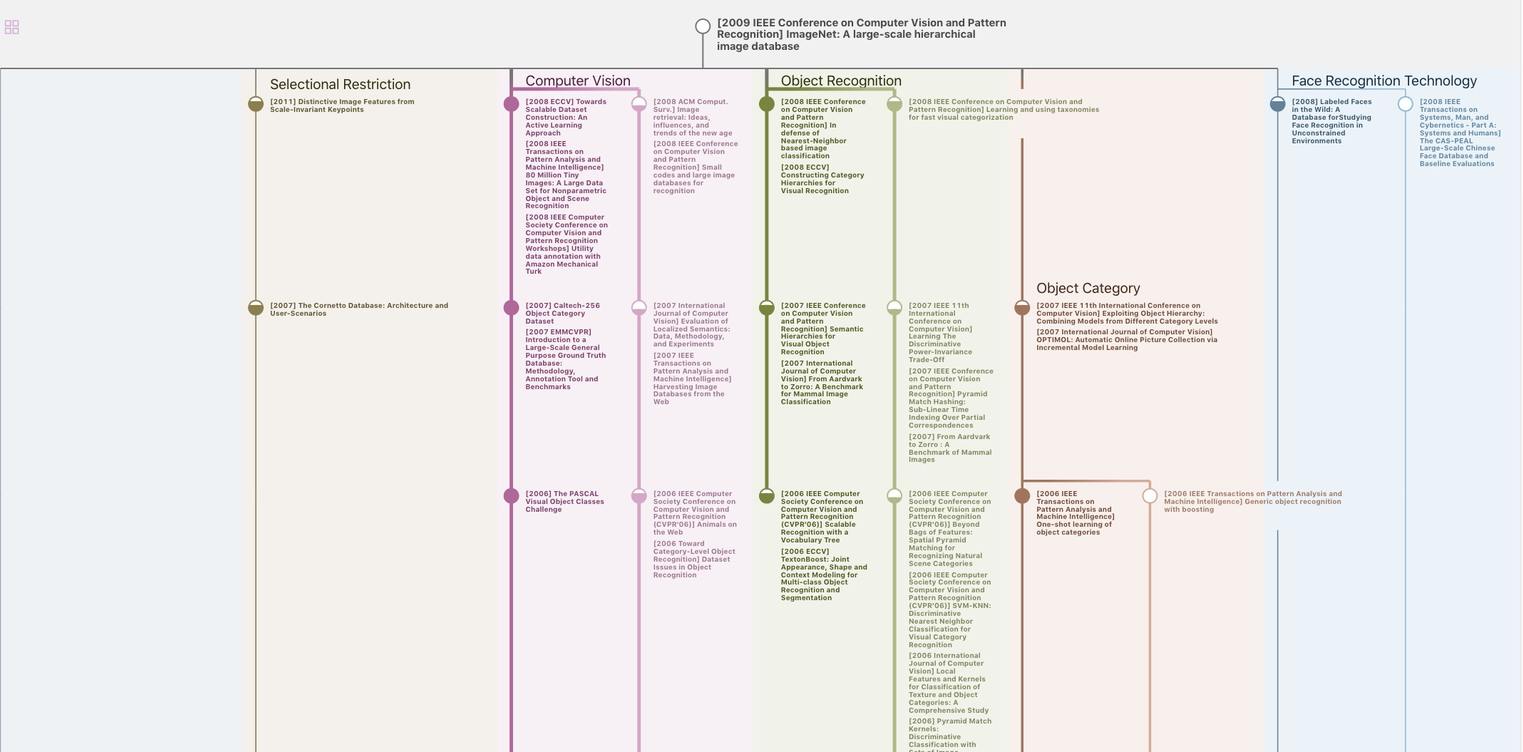
生成溯源树,研究论文发展脉络
Chat Paper
正在生成论文摘要