A Hierarchical Feature-Based Methodology To Perform Cervical Cancer Classification
APPLIED SCIENCES-BASEL(2021)
摘要
Prevention of cervical cancer could be performed using Pap smear image analysis. This test screens pre-neoplastic changes in the cervical epithelial cells; accurate screening can reduce deaths caused by the disease. Pap smear test analysis is exhaustive and repetitive work performed visually by a cytopathologist. This article proposes a workload-reducing algorithm for cervical cancer detection based on analysis of cell nuclei features within Pap smear images. We investigate eight traditional machine learning methods to perform a hierarchical classification. We propose a hierarchical classification methodology for computer-aided screening of cell lesions, which can recommend fields of view from the microscopy image based on the nuclei detection of cervical cells. We evaluate the performance of several algorithms against the Herlev and CRIC databases, using a varying number of classes during image classification. Results indicate that the hierarchical classification performed best when using Random Forest as the key classifier, particularly when compared with decision trees, k-NN, and the Ridge methods.
更多查看译文
关键词
image classification, learning algorithm, Random Forest classifier, hierarchical model, cervical lesions, cancer classification, feature extraction, Pap smear
AI 理解论文
溯源树
样例
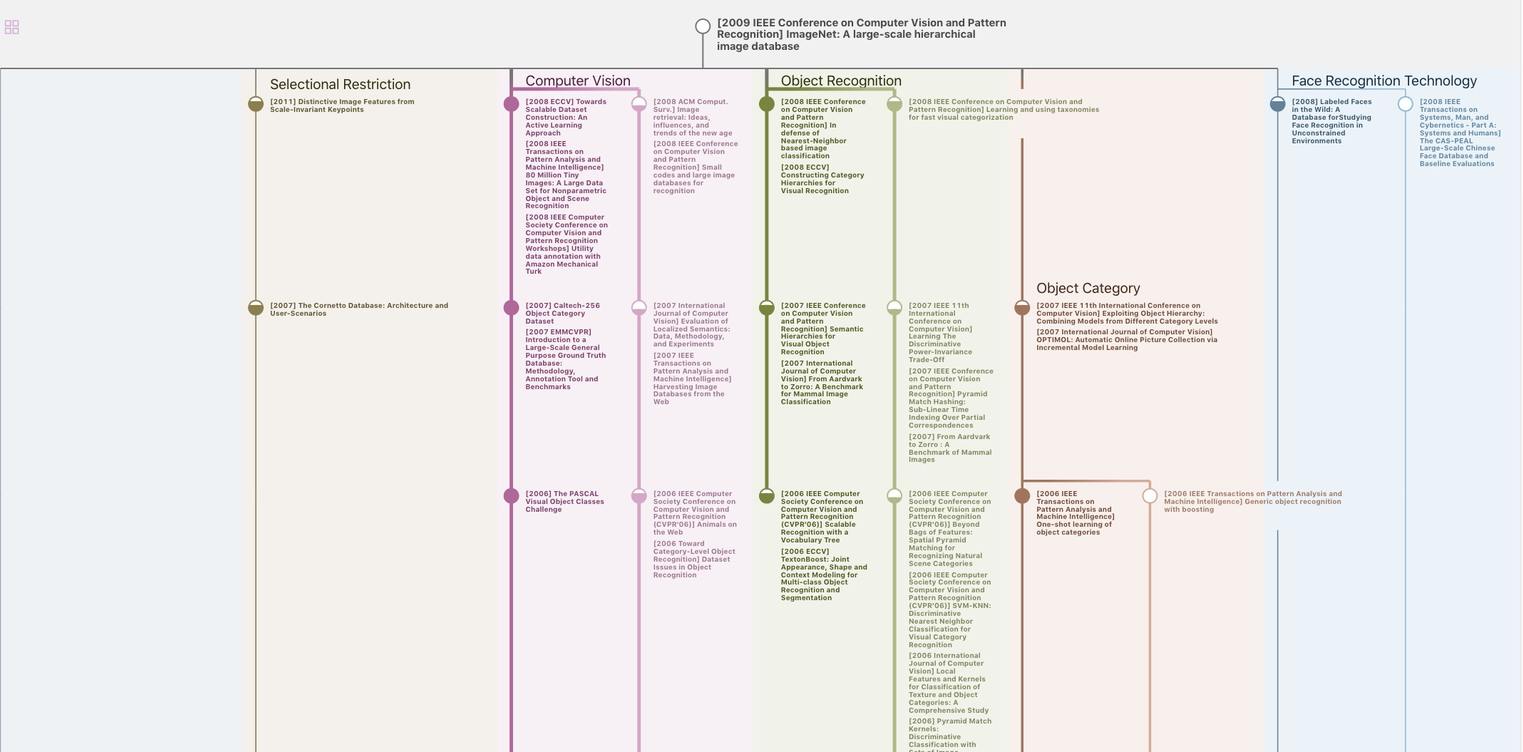
生成溯源树,研究论文发展脉络
Chat Paper
正在生成论文摘要