A Primer On Applying Ai Synergistically With Domain Expertise To Oncology
BIOCHIMICA ET BIOPHYSICA ACTA-REVIEWS ON CANCER(2021)
摘要
Background: The concurrent growth of large-scale oncology data alongside the computational methods with which to analyze and model it has created a promising environment for revolutionizing cancer diagnosis, treatment, prevention, and drug discovery. Computational methods applied to large datasets have accelerated the drug discovery process by reducing bottlenecks and widening the search space beyond what is experimentally tractable. As the research community gains understanding of the myriad genetic underpinnings of cancer via sequencing, imaging, screens, and more that are ingested, transformed, and modeled by top open-source machine learning and artificial intelligence tools readily available, the next big drug candidate might seem merely an "Enter" key away. Of course, the reality is more convoluted, but still promising.Scope of review: We present methods to approach the process of building an AI model, with strong emphasis on the aspects of model development we believe to be crucial to success but that are not commonly discussed: diligence in posing questions, identifying suitable datasets and curating them, and collaborating closely with biology and oncology experts while designing and evaluating the model. Digital pathology, Electronic Health Records, and other data types outside of high-throughput molecular data are reviewed well by others and outside of the scope of this review.This review emphasizes the importance of considering the limitations of the datasets, computational methods, and our minds when designing AI models. For example, datasets can be biased towards areas of research interest, funding, and particular patient populations. Neural networks may learn representations and correlations within the data that are grounded not in biological phenomena, but statistical anomalies erroneously extracted from the training data. Researchers may mis-interpret or over-interpret the output, or design and evaluate the training process such that the resultant model generalizes poorly.Fortunately, awareness of the strengths and limitations of applying data analytics and AI to drug discovery enables us to leverage them carefully and insightfully while maximizing their utility. These applications when performed in close collaboration with domain experts, together with continuous critical evaluation, generation of new data to minimize known blind spots as they are found, and rigorous experimental validation, increases the success rate of the study. We will discuss applications including AI-assisted target identification, drug repurposing, patient stratification, and gene prioritization.Major conclusions: Data analytics and AI have demonstrated capabilities to revolutionize cancer research, prevention, and treatment by maximizing our understanding and use of the expanding panoply of experimental data. However, to separate promise from true utility, computational tools must be carefully designed, critically evaluated, and constantly improved. Once that is achieved, a human-computer hybrid discovery process will outperform one driven by each alone. General significance: This review highlights the challenges and promise of synergizing predictive AI models with human expertise towards greater understanding of cancer.
更多查看译文
AI 理解论文
溯源树
样例
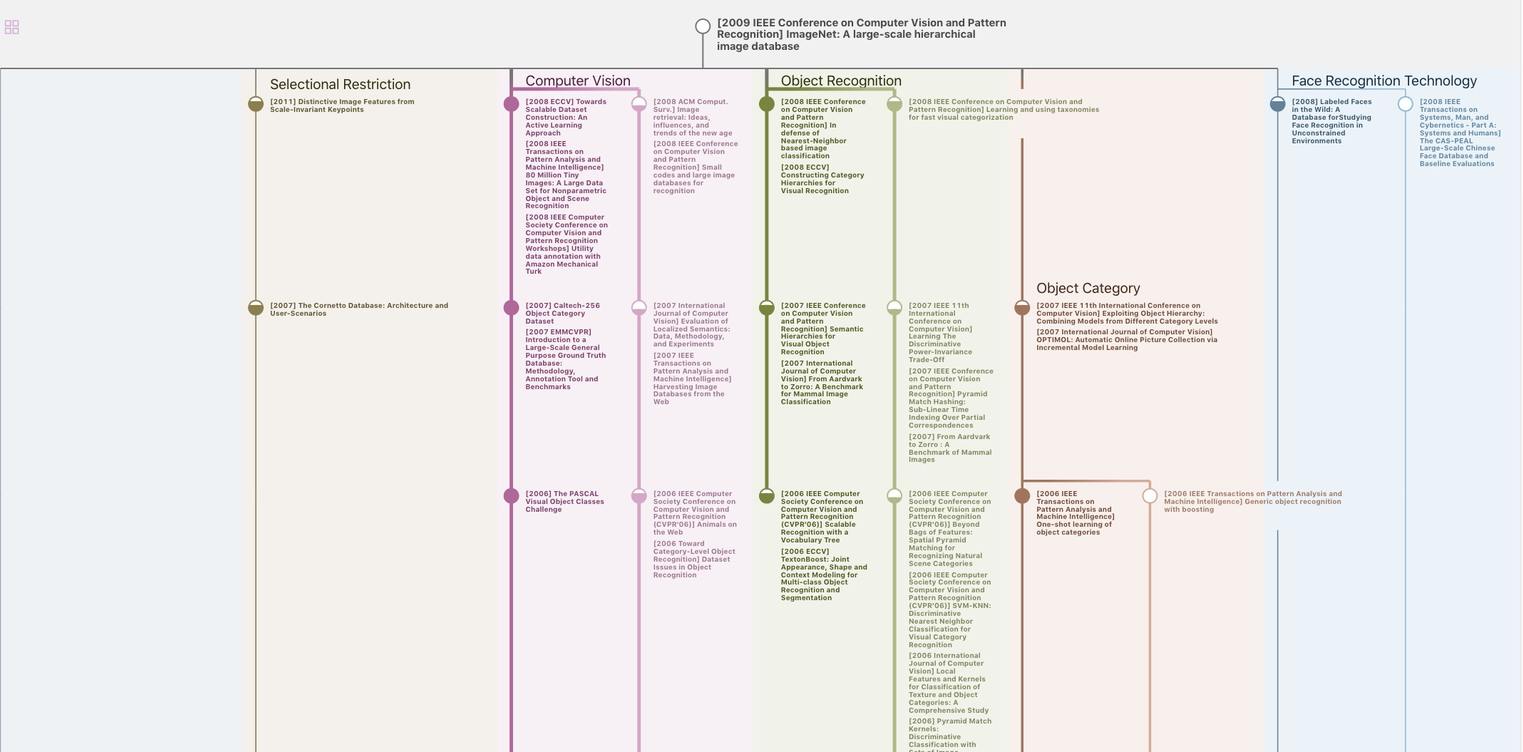
生成溯源树,研究论文发展脉络
Chat Paper
正在生成论文摘要