DAEANet: Dual auto-encoder attention network for depth map super-resolution.
Neurocomputing(2021)
摘要
Recently, depth map super-resolution (DSR) has obtained remarkable performance with the development of convolutional neural networks (CNNs). High-resolution (HR) depth map can be inferred from a low-resolution (LR) one with the guidance of its corresponding HR intensity image. However, most of the existing CNNs-based methods unilaterally transfer structures information of guidance image to the input depth map, which ignores the corresponding relations between the depth map and the intensity map. In this paper, we propose a novel dual auto-encoder attention network (DAEANet) for DSR. The proposed DAEANet includes two auto-encoder networks, where guidance auto-encoder network (GAENet) and target auto-encoder network (TAENet) aim to extract feature information from intensity image and depth map. Specifically, all auto-encoder networks are similar and trained simultaneously to ensure structural consistency. Furthermore, to preserve the structure information in the process of training, the attention mechanism is employed to our DAEANet. Extensive experiments on several popular benchmarks show that the proposed DAEANet outperforms existing state-of-the-art algorithms.
更多查看译文
关键词
Depth map super-resolution,Convolutional neural network,Auto-encoder network,Attention mechanism
AI 理解论文
溯源树
样例
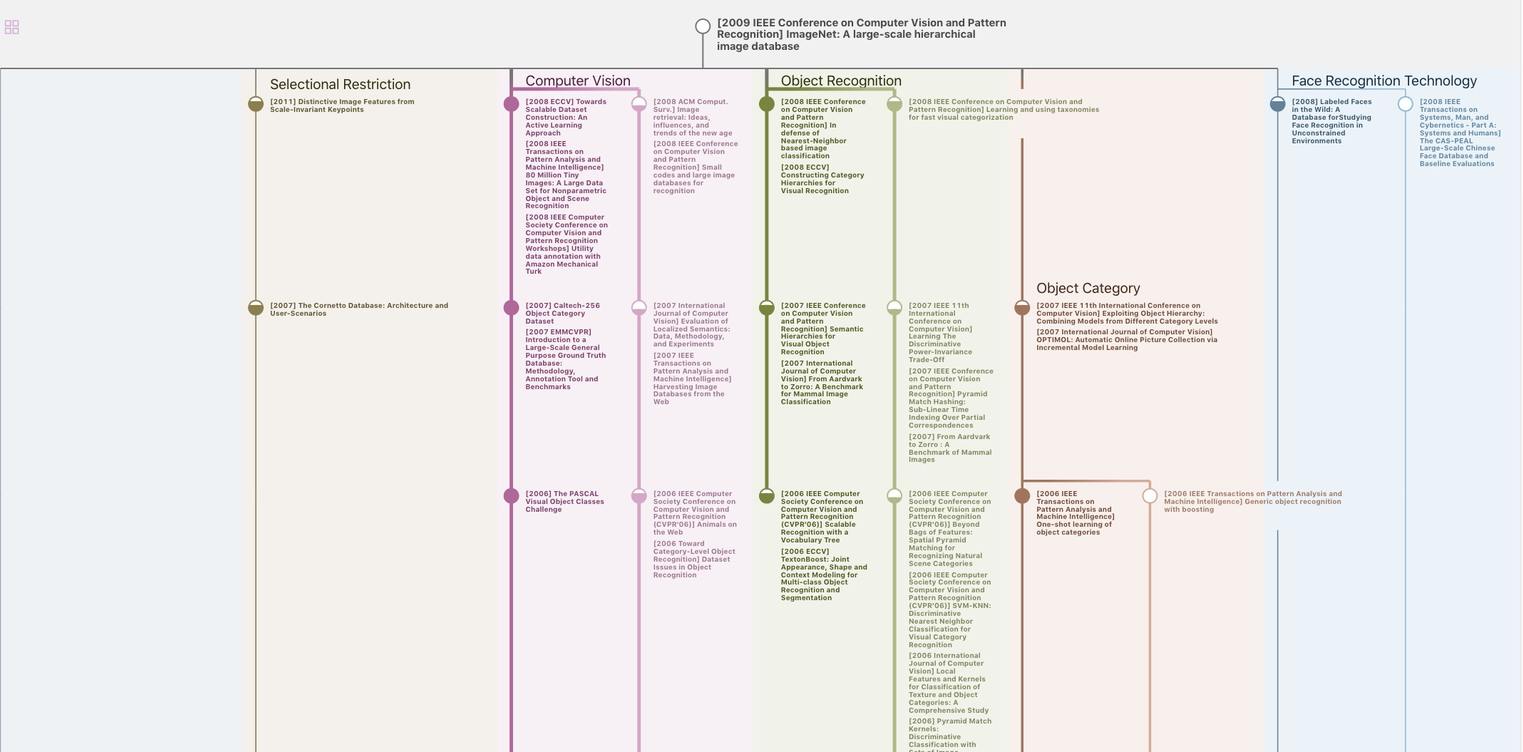
生成溯源树,研究论文发展脉络
Chat Paper
正在生成论文摘要