Ensemble Oscillation Correction (Enoc): Leveraging Oscillatory Modes To Improve Forecasts Of Chaotic Systems
JOURNAL OF CLIMATE(2021)
摘要
Oscillatory modes of the climate system are among its most predictable features, especially at intraseasonal time scales. These oscillations can be predicted well with data-driven methods, often with better skill than dynamical models. However, since the oscillations only represent a portion of the total variance, a method for beneficially combining oscillation forecasts with dynamical forecasts of the full system was not previously known. We introduce Ensemble Oscillation Correction (EnOC), a general method to correct oscillatory modes in ensemble forecasts from dynamical models. We compute the ensemble mean-or the ensemble probability distribution-with only the best ensemble members, as determined by their discrepancy from a data-driven forecast of the oscillatory modes. We also present an alternate method that uses ensemble data assimilation to combine the oscillation forecasts with an ensemble of dynamical forecasts of the system (EnOC-DA). The oscillatory modes are extracted with a time series analysis method called multichannel singular spectrum analysis (M-SSA), and forecast using an analog method. We test these two methods using chaotic toy models with significant oscillatory components and show that they robustly reduce error compared to the uncorrected ensemble. We discuss the applications of this method to improve prediction of monsoons as well as other parts of the climate system. We also discuss possible extensions of the method to other data-driven forecasts, including machine learning.
更多查看译文
关键词
Climate prediction, Forecasting techniques, Statistical forecasting, Data assimilation, Nonlinear dynamics, Machine learning
AI 理解论文
溯源树
样例
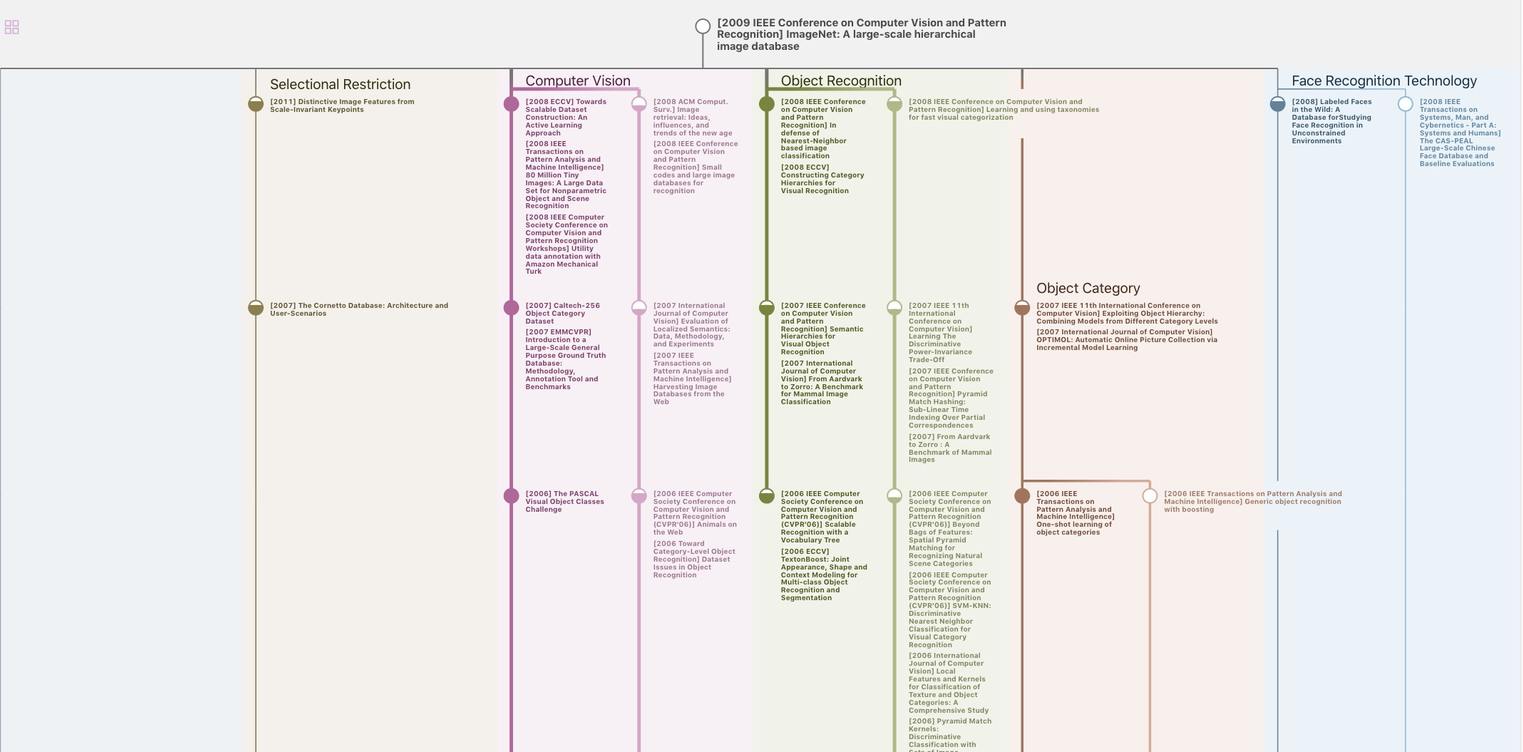
生成溯源树,研究论文发展脉络
Chat Paper
正在生成论文摘要