Anomaly Detection In Seismic Data-Metadata Using Simple Machine-Learning Models
SEISMOLOGICAL RESEARCH LETTERS(2021)
Abstract
In modern seismological analysis, it is not unusual to process huge amounts of data, as illustrated by two case studies exemplified in this work, both assessing the quality of several millions of segments selected for computing local and energy magnitudes. In this scenario, quality control tools to filter, discard, or rank data are of extreme importance and should ideally be simple, fast, and generalizable. Using machine-learning tools, we present here a simple and efficient model based on the isolation forest algorithm for detecting amplitude anomalies on any seismic waveform segment, with no restriction on the segment record content (earthquake vs. noise) and no additional requirements than the segment metadata. By considering a simple feature space composed of amplitudes of each segment's power spectral density (PSD) evaluated at selected periods suitable for both local and teleseismic applications, feature selection revealed that one single feature, the PSD at 5 s, is sufficient to achieve the best predicting performances. The evaluation results report average precision scores around 0.97, and maximum F1 scores above 0.9, both remarkable results with respect to the simplicity of the approach used and the generality of the problem tackled. The trained model producing the best evaluation results is the backbone of a publicly available software, which computes an amplitude anomaly score in [0, 1] for any given seismic waveform, and can be beneficial in several applications such as discarding anomalies from data sets, ideally in a preprocessing stage, and detecting potential metadata problems on data center side. When applied to our two case studies, the software was revealed to be fast and effective, and the computed anomaly scores allow additional flexibility in addition to the proven wide-range applicability.
MoreTranslated text
Key words
seismic data–metadata,anomaly detection,machine-learning machine-learning
AI Read Science
Must-Reading Tree
Example
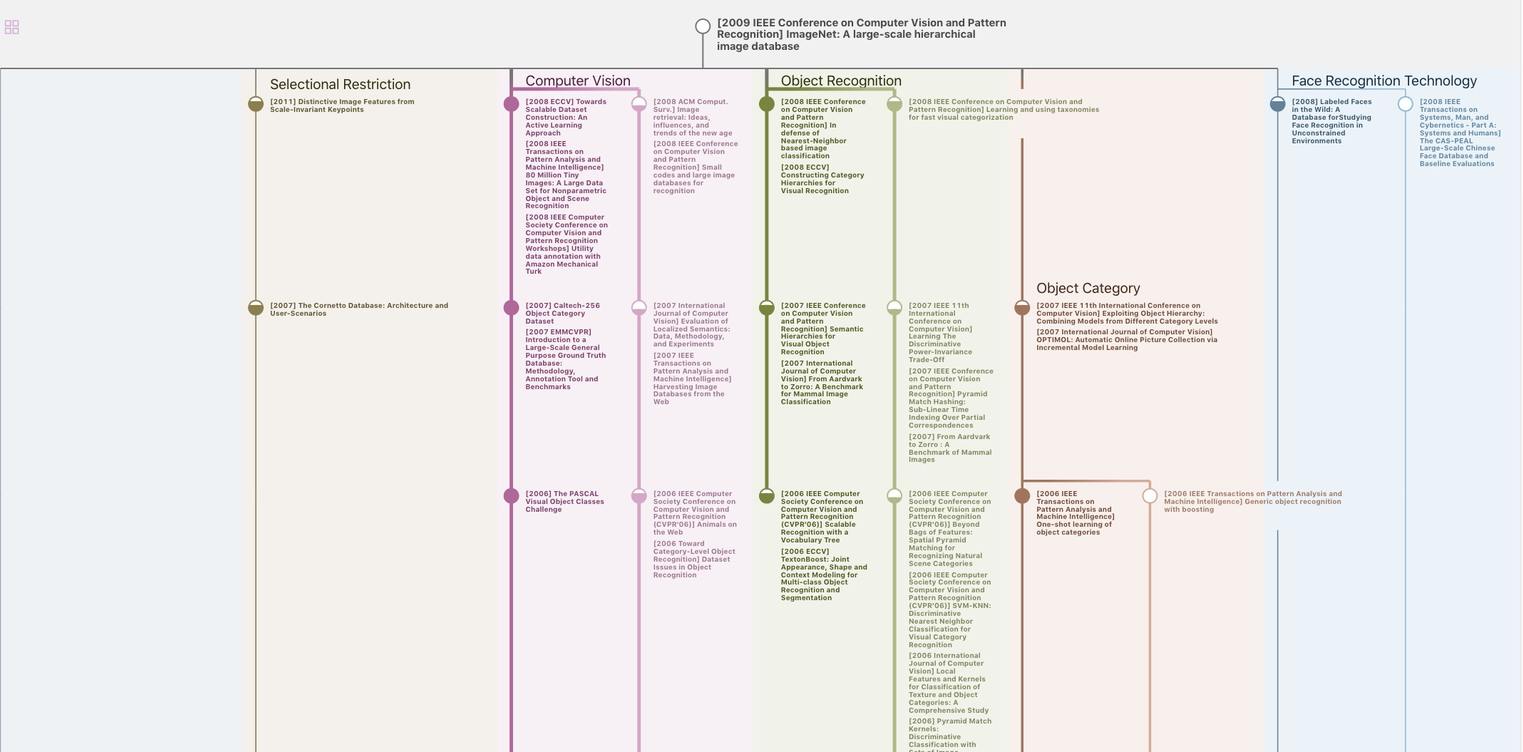
Generate MRT to find the research sequence of this paper
Chat Paper
Summary is being generated by the instructions you defined