Deep Learning Modulation Recognition for RF Spectrum Monitoring
2021 IEEE INTERNATIONAL SYMPOSIUM ON CIRCUITS AND SYSTEMS (ISCAS)(2021)
摘要
This paper presents a classification Convolutional Neural Network model for modulation recognition. The model is capable of classifying 11 different modulation techniques based on their In-phase and Quadrature components at baseband. The classification accuracy is higher than 80% for signals with a Signal-to-Noise Ratio higher than 2 dB. The model performance is evaluated using the same In-phase and Quadrature component data-sets used in the state of the art. Compared to previous work, the number of parameters and multiplications/additions is reduced by several orders of magnitude. The proposed Convolutional Neural Network is implemented on FPGA and achieves the same performance as the GPU model. Compared to other FPGA implementations of RF signal classifiers, the proposed implementation classifies twice as much modulation schemes while consuming only half the dynamic power.
更多查看译文
关键词
Deep Learning, Convolutional Neural Networks, Modulation Recognition, Cognitive Radio, Spectrum Monitoring, Dynamic Spectrum Access, FPGA
AI 理解论文
溯源树
样例
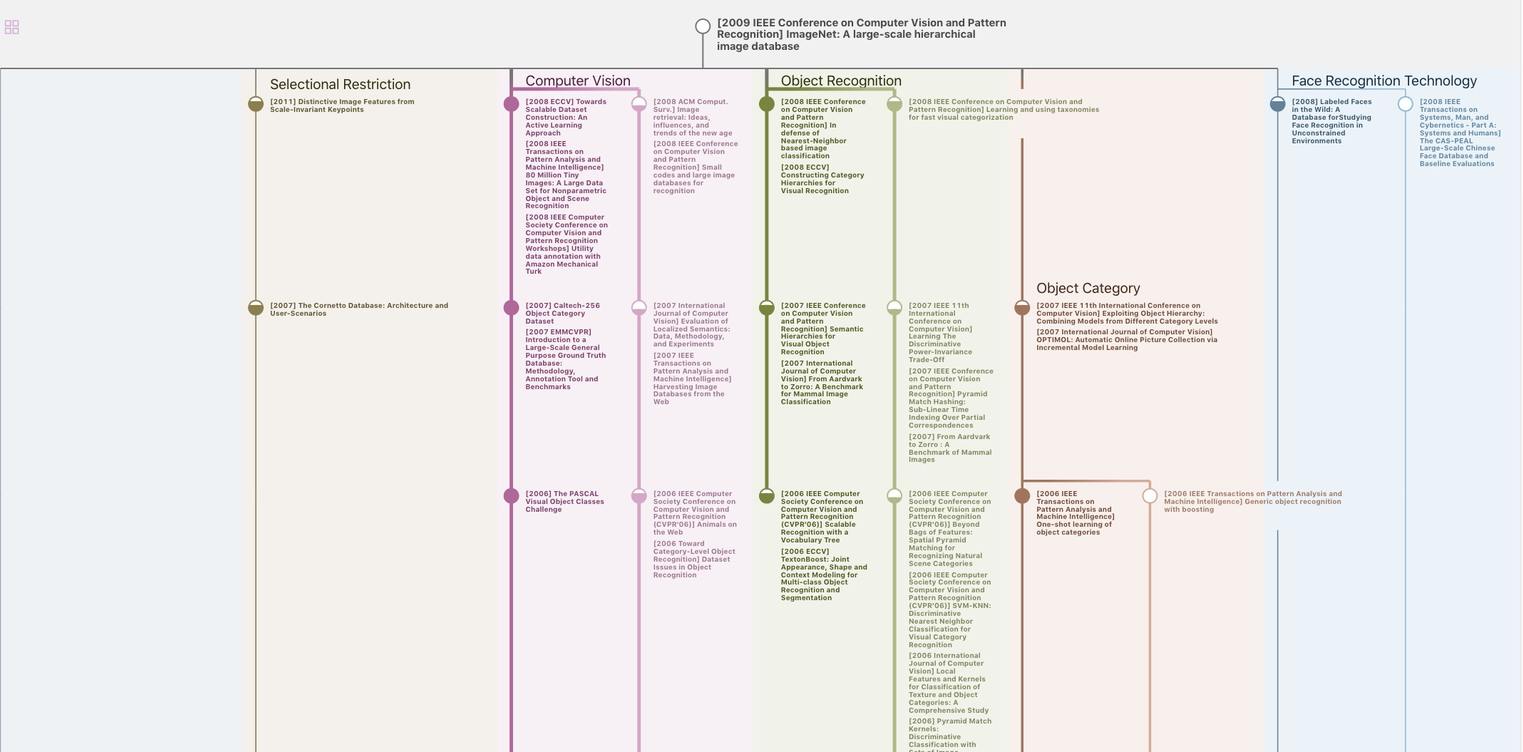
生成溯源树,研究论文发展脉络
Chat Paper
正在生成论文摘要