Online-adaptive classification and regression network with sample-efficient meta learning for long-term tracking
Image and Vision Computing(2021)
Abstract
Classification and regression-based trackers (CAR) are widely adopted to tackle the short-term visual tracking task. However, the existing CAR tackers either employ offline-trained regression models based on predefined anchor-boxes, or online update their models in a rough and inflexible way, which leads to the lack of long-term adaptability for target deformations and appearance variations. To overcome this limitation, we propose a novel long-term tracking framework LT-CAR utilizing sample-efficient meta learning to online optimize both the classification and regression model. Specifically, we first introduce the ridge regression to a fully convolutional network as our regression branch, and then implement a vertically stacked GRU module termed as Meta-Sample-Filter to keep historical information about the target as well as help our model learn what to learn. Moreover, we extend our framework for long-term tracking by introducing a carefully designed spatial–temporal verification network to identify tracking failures, and a query-guided detector to conduct global re-detection. Experimental results on LaSOT, VOT-LT2018, VOT-LT2019, and TLP benchmarks show that our LT-CAR achieves comparable performance to the state-of-the-art long-term algorithms.
MoreTranslated text
Key words
Long-term tracking,Target regression,Online learning,Meta learning
AI Read Science
Must-Reading Tree
Example
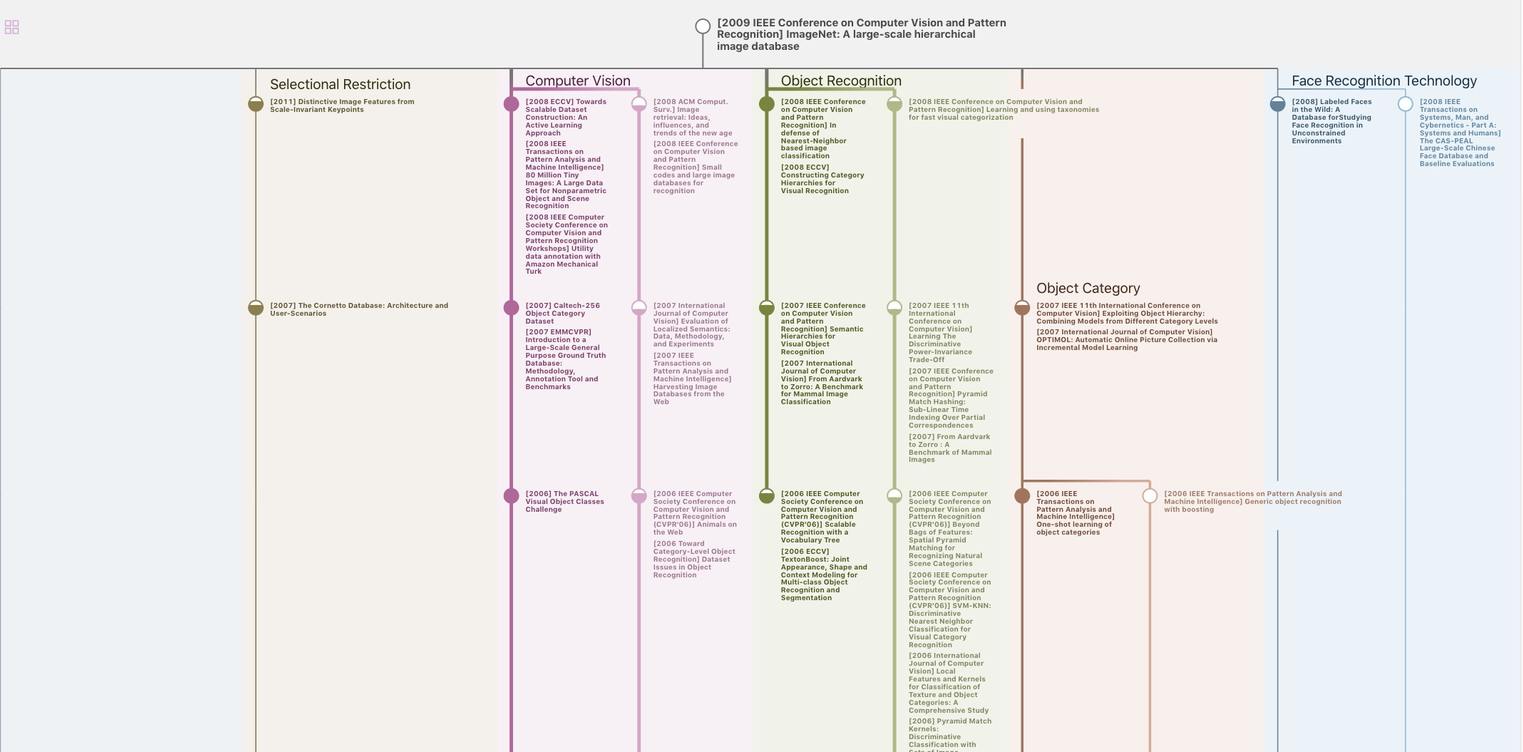
Generate MRT to find the research sequence of this paper
Chat Paper
Summary is being generated by the instructions you defined