Depth Classification Of Defects Based On Neural Architecture Search
IEEE ACCESS(2021)
摘要
As an important part of non-destructive testing, infrared thermography testing is widely used in various fields of industrial development for monitoring the quality of metal parts. Considering the problem of low detection rate of surface defects on steel parts, we explored the application of neural architecture search (NAS) in infrared thermography area for the first time. On the one hand, we compared different time-series temperature features of defect locations in infrared images and validate the performance of three different features such as heating, cooling and full process by machine learning methods. On the other hand, we searched for multilayer perceptron through NAS technology to classify defects with different depths. Experiments have proved that the time-series temperature feature is very effective when used in the depth classification of defects, and the accuracy rate can reach 93% under the verification of traditional machine learning methods. The NAS technique used in this paper can search 100 multilayer perceptrons in a minimum of 121s and achieve 100% defect classification accuracy.
更多查看译文
关键词
Steel, Neural networks, Lasers, Testing, Reinforcement learning, Multilayer perceptrons, Machine learning, Non-destructive testing, infrared thermography, neural architecture search, classification
AI 理解论文
溯源树
样例
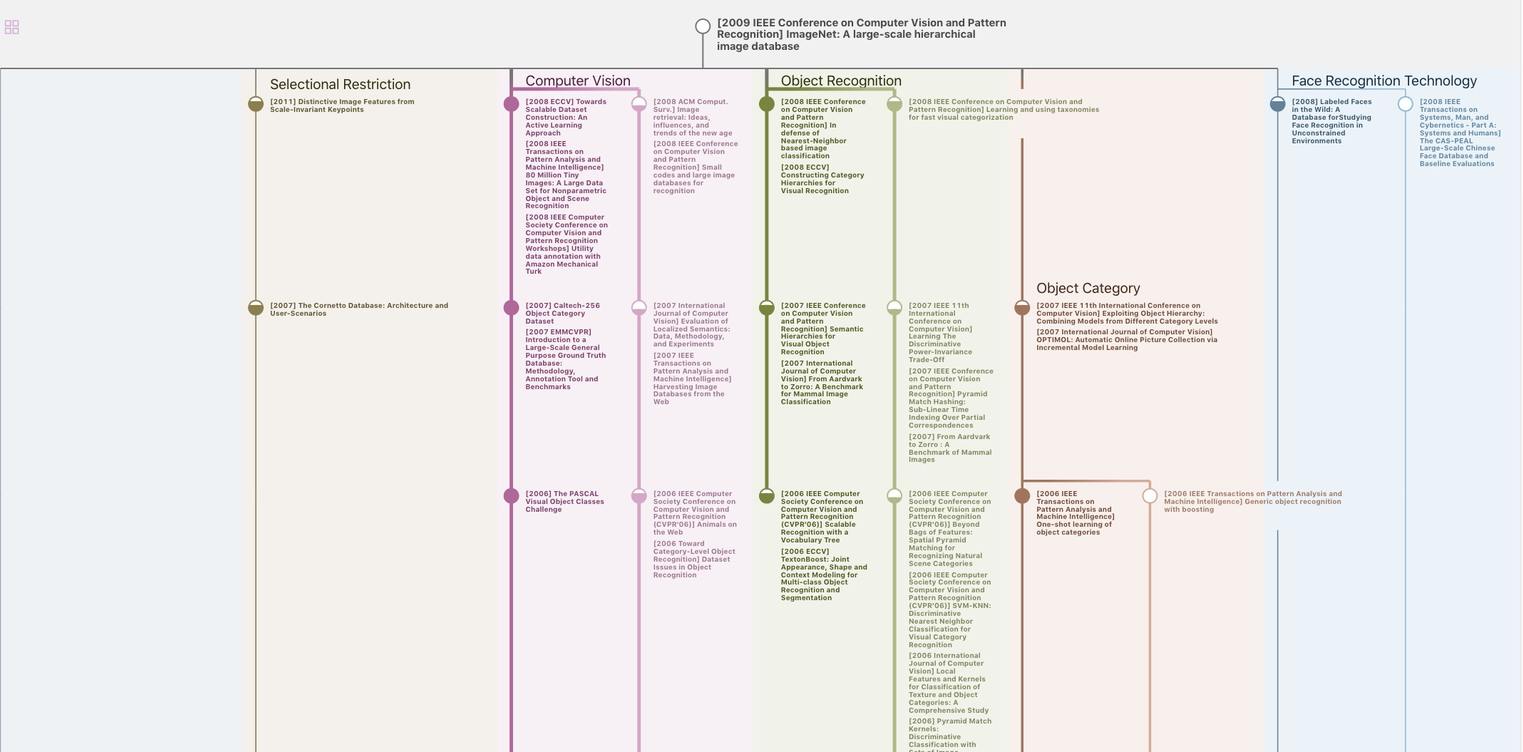
生成溯源树,研究论文发展脉络
Chat Paper
正在生成论文摘要