Long Short-Term Memory Networks For Pattern Recognition Of Synthetical Complete Earthquake Catalog
SUSTAINABILITY(2021)
摘要
Exploring the spatiotemporal distribution of earthquake activity, especially earthquake migration of fault systems, can greatly to understand the basic mechanics of earthquakes and the assessment of earthquake risk. By establishing a three-dimensional strike-slip fault model, to derive the stress response and fault slip along the fault under regional stress conditions. Our study helps to create a long-term, complete earthquake catalog. We modelled Long-Short Term Memory (LSTM) networks for pattern recognition of the synthetical earthquake catalog. The performance of the models was compared using the mean-square error (MSE). Our results showed clearly the application of LSTM showed a meaningful result of 0.08% in the MSE values. Our best model can predict the time and magnitude of the earthquakes with a magnitude greater than Mw = 6.5 with a similar clustering period. These results showed conclusively that applying LSTM in a spatiotemporal series prediction provides a potential application in the study of earthquake mechanics and forecasting of major earthquake events.
更多查看译文
关键词
long short-term memory networks, pattern recognition, earthquake catalog, physics-based simulation
AI 理解论文
溯源树
样例
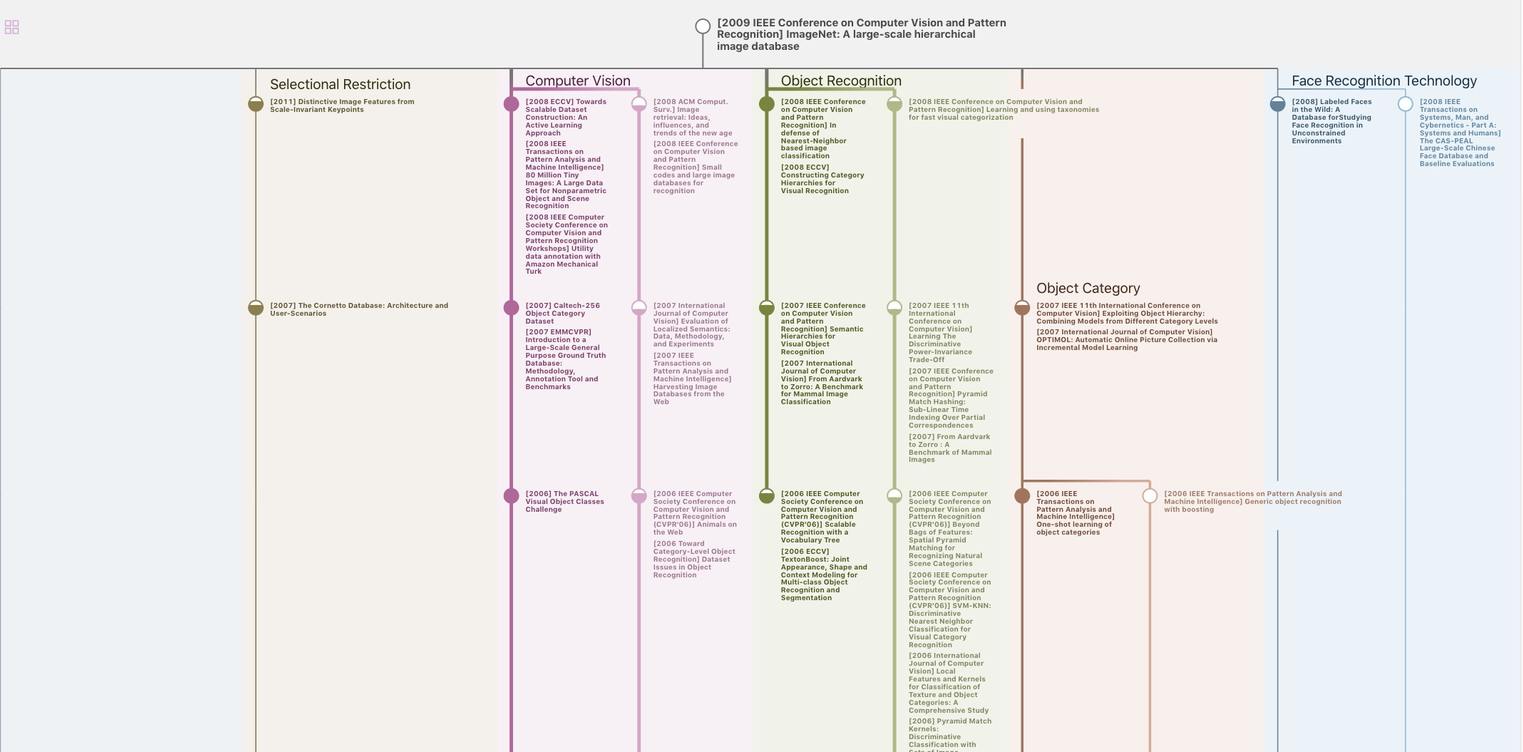
生成溯源树,研究论文发展脉络
Chat Paper
正在生成论文摘要