Changes In Air Pollutants During The Covid-19 Lockdown In Beijing: Insights From A Machine-Learning Technique And Implications For Future Control Policy
ATMOSPHERIC AND OCEANIC SCIENCE LETTERS(2021)
摘要
`The COVID-19 lockdowns led to abrupt reductions in human-related emissions worldwide and had an unintended impact on air quality improvement. However, quantifying this impact is difficult as meteorological conditions may mask the real effect of changes in emissions on the observed concentrations of pollutants. Based on the air quality and meteorological data at 35 sites in Beijing from 2015 to 2020, a machine learning technique was applied to decouple the impacts of meteorology and emissions on the concentrations of air pollutants. The results showed that the real ("deweathered") concentrations of air pollutants (expect for O-3) dropped significantly due to lockdown measures. Compared with the scenario without lockdowns (predicted concentrations), the observed values of PM2.5, PM10, SO2, NO2, and CO during lockdowns decreased by 39.4%, 50.1%, 51.8%, 43.1%, and 35.1%, respectively. In addition, a significant decline for NO2 and CO was found at the background sites (51% and 37.8%) rather than the traffic sites (37.1% and 35.5%), which is different from the common belief. While the primary emissions reduced during the lockdown period, episodic haze events still occurred due to unfavorable meteorological conditions. Thus, developing an optimized strategy to tackle air pollution in Beijing is essential in the future.
更多查看译文
关键词
Random forest model, Air pollutants, Meteorological normalization, COVID-19, Emission control strategy
AI 理解论文
溯源树
样例
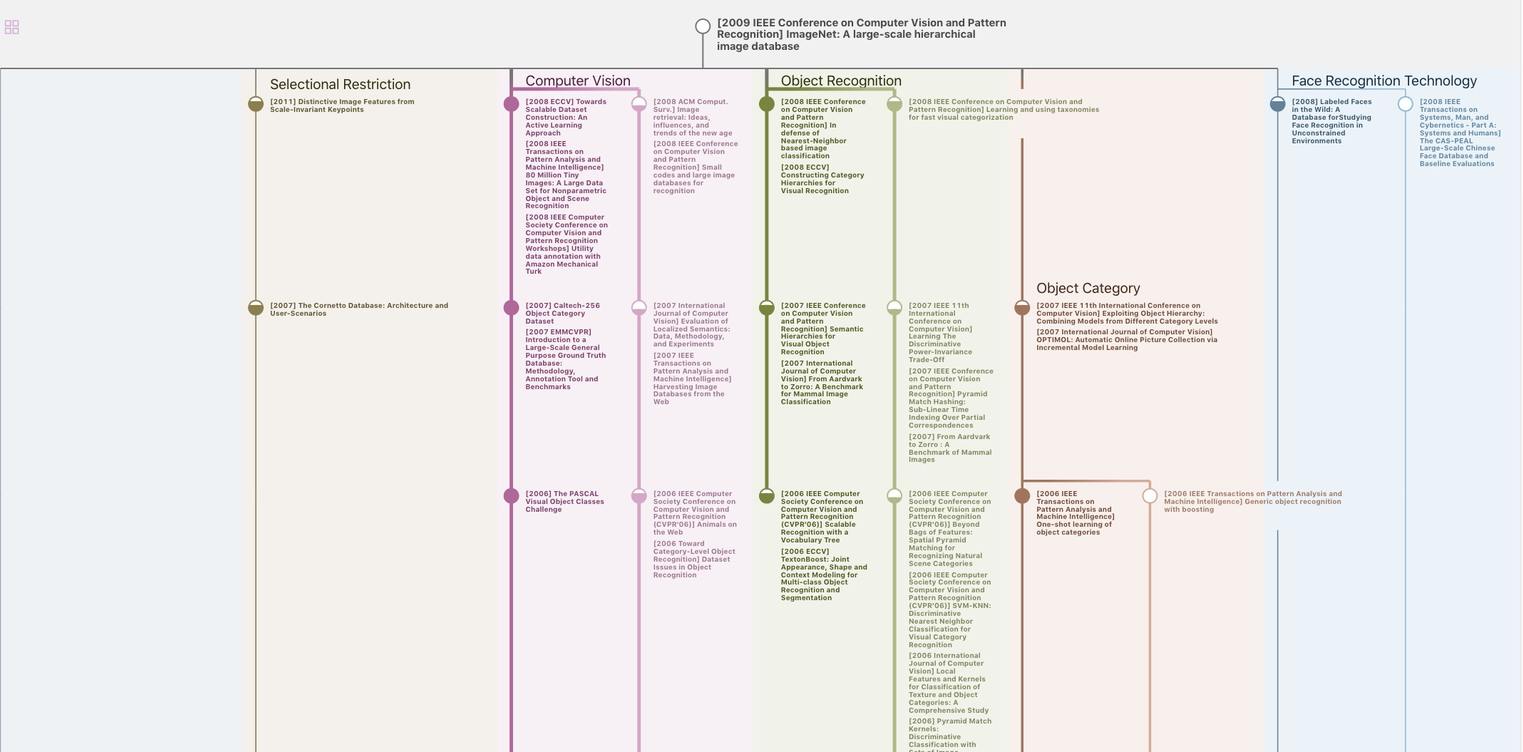
生成溯源树,研究论文发展脉络
Chat Paper
正在生成论文摘要