Face Spoof Attack Detection With Hypergraph Capsule Convolutional Neural Networks
INTERNATIONAL JOURNAL OF COMPUTATIONAL INTELLIGENCE SYSTEMS(2021)
摘要
Face authentication has been widely used in personal identification. However, face authentication systems can be attacked by fake images. Existing methods try to detect such attacks with different features. Among them, using color images become popular since it is flexible and generic. In this paper, a novel feature representation for face spoof attack detection, namely hypergraph capsule convolutional neural networks (HGC-CNNs), is proposed, which takes advantage of multiple features. To achieve it, capsule neural networks are used to integrate different types of features. In addition, hypergraph regularization is applied to learn the correlations among samples. In this way, the descriptive power is improved. The proposed feature representation is compared with existing features for face spoof attack detection and experimental results on two commonly used datasets emphasize the effectiveness of HGC-CNN. (C) 2021 The Authors. Published by Atlantis Press B.V.
更多查看译文
关键词
Face spoof attack detection, Multiple-feature learning, Capsule neural networks, Hypergraph regularization
AI 理解论文
溯源树
样例
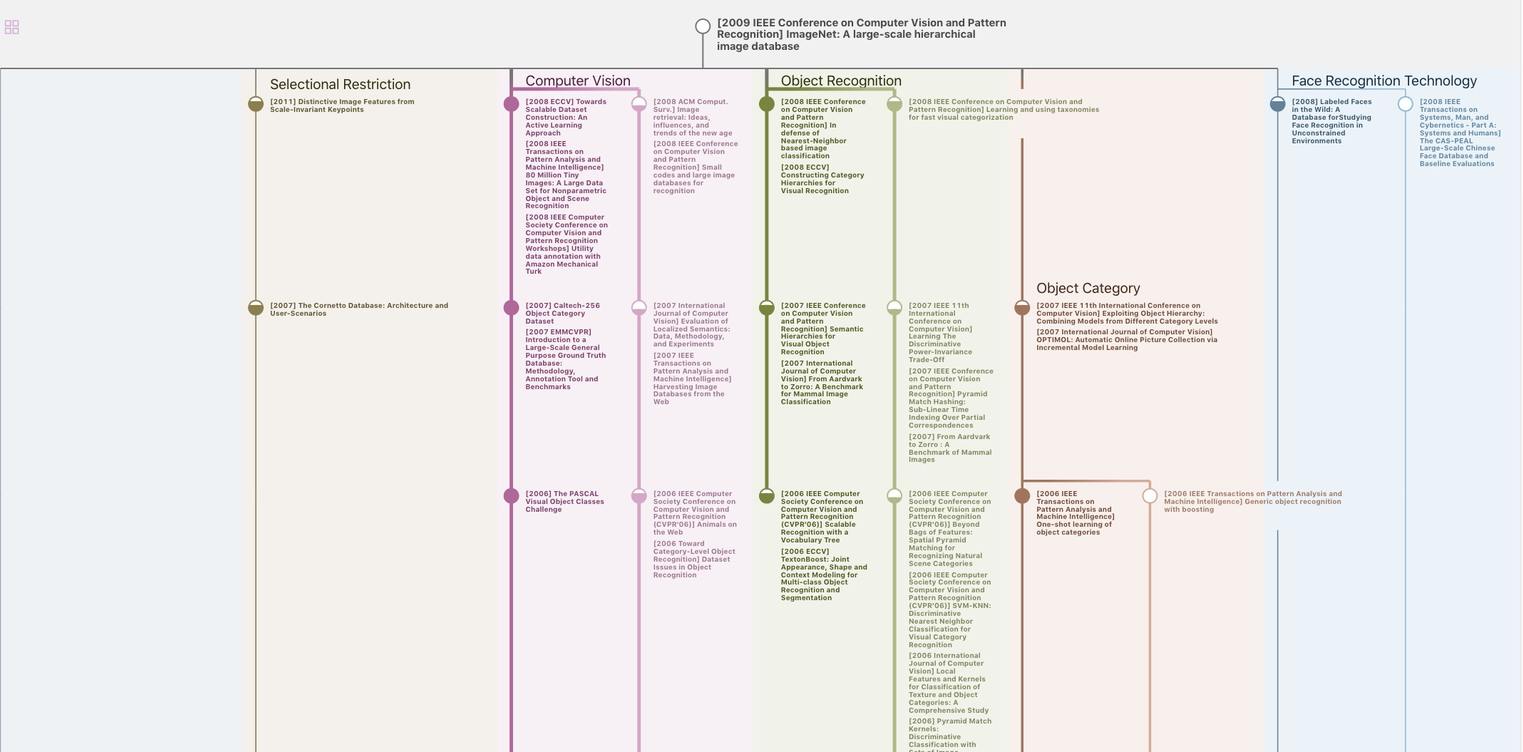
生成溯源树,研究论文发展脉络
Chat Paper
正在生成论文摘要