Finding An Optimal Geometric Configuration For Tdoa Location Systems With Reinforcement Learning
IEEE ACCESS(2021)
摘要
In TDOA passive location tasks, the geometric configuration can greatly affect the positioning precision due to the complicated characteristics of electromagnetic environment. How to find an appropriate path to a good geometry to locate the transmitter accurately is vital in practical location tasks. This paper proposes a novel geometry optimization method based on deep reinforcement learning. In the proposed method, stations are regarded as mobile agents that can receive wireless signals decide where to go. All agents are controlled by an actor-critic learner, which is trained on the experiences collected from executing the TDOA location task repeatedly. To evaluate the trained agents, a TDOA location simulator environment with complex electromagnetic characteristics is developed. The empirical results show that, the learner mastered useful strategies and navigated to optimal geometric configurations efficiently. A visual depiction of highlights of the learner's behavior in TDOA passive location tasks can be viewed in the video provided in the supplementary material.
更多查看译文
关键词
Transmitters, Reinforcement learning, Geometry, Electromagnetics, Task analysis, Estimation, Neural networks, Geometry optimaztion, passive location, TDOA, reinforcement learning, actor-critic
AI 理解论文
溯源树
样例
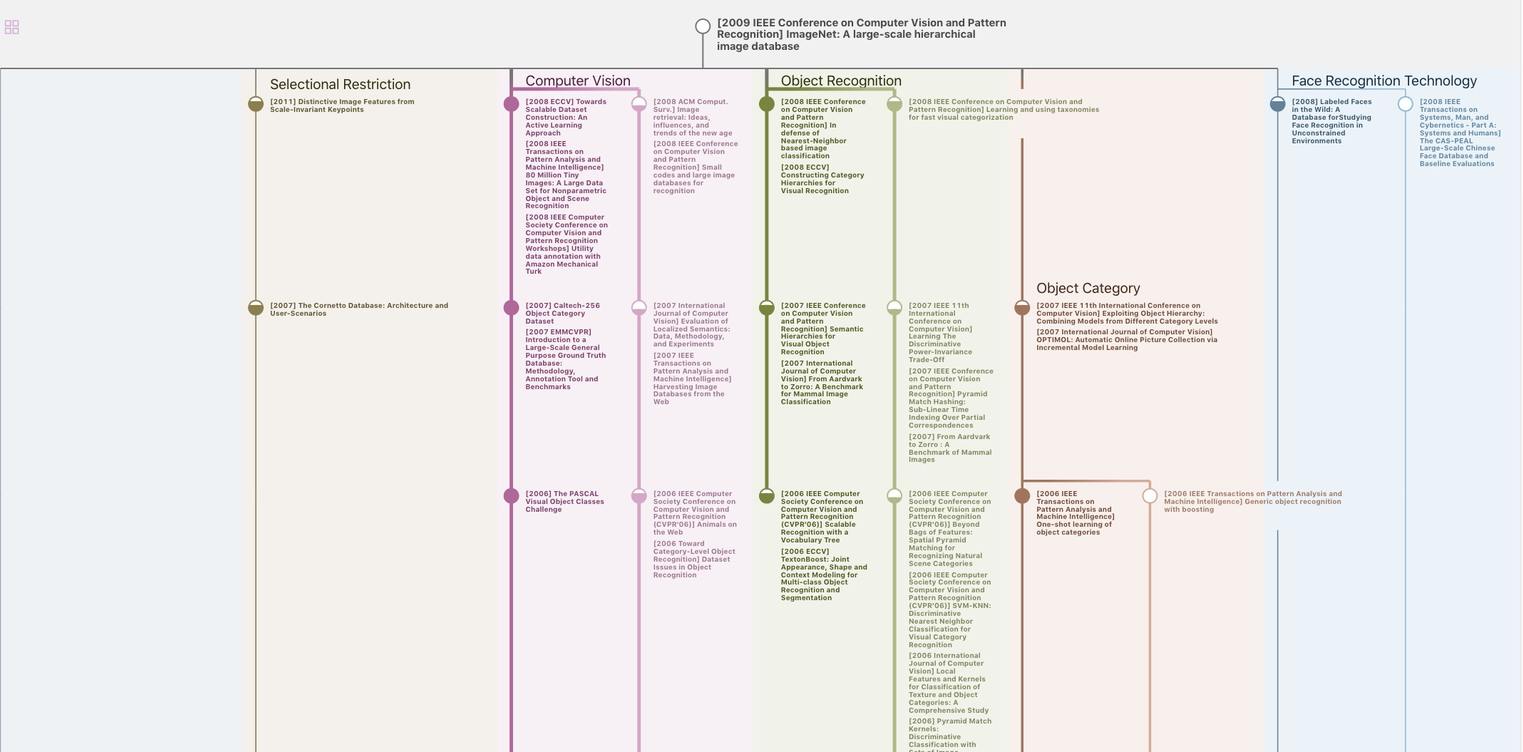
生成溯源树,研究论文发展脉络
Chat Paper
正在生成论文摘要