Automatic Detection of Melanins and Sebums from Skin Images Using a Generative Adversarial Network
Cognitive Computation(2021)
摘要
Melanins and sebums are two important criteria for the quality evaluation of skin, and they are capable of providing customized suggestions for skin care. Currently, their detection is heavily relied on manual process performed by specialists in laboratory. Although efficient, such a manual detection is an expensive and labor-intensive procedure, and hence, there has been great interest in developing computational models for automatic detection of melanins and sebums from skin images. In this work, we propose an automatic detection algorithm, namely DAME, to identify these two kinds of substances based on a generative adversarial network (GAN). To do so, DAME makes use of a variant of GAN, i.e., pix2pix, due to its strength in image generation by learning the structural and contextual information of melanins and sebums observed from skin images. With these additional augmented images, a robust U-Net model can be learned for automatically detecting and marking melanins and sebums. To evaluate the performance of DAME, we have conducted a series of experiments by comparing it with several existing algorithms on real image datasets, and the results have demonstrated that DAME yields a substantially better detection accuracy than previously published algorithms in terms of several independent evaluation metrics. Moreover, DAME is believed to be more robust than other algorithms, as it obtains the smallest variance for each metric. Hence, DAME makes it possible to automatically detect melanins and sebums with a promising performance. Due to the strong learning ability of GAN, DAME is also able to identify melanins and sebums that are possibly ignored by specialists. The source codes of DAME and datasets used in the experiments are available at https://github.com/reBioco-der/DAME .
更多查看译文
关键词
Automatic detection, Skin images, Generative adversarial network, pix2pix
AI 理解论文
溯源树
样例
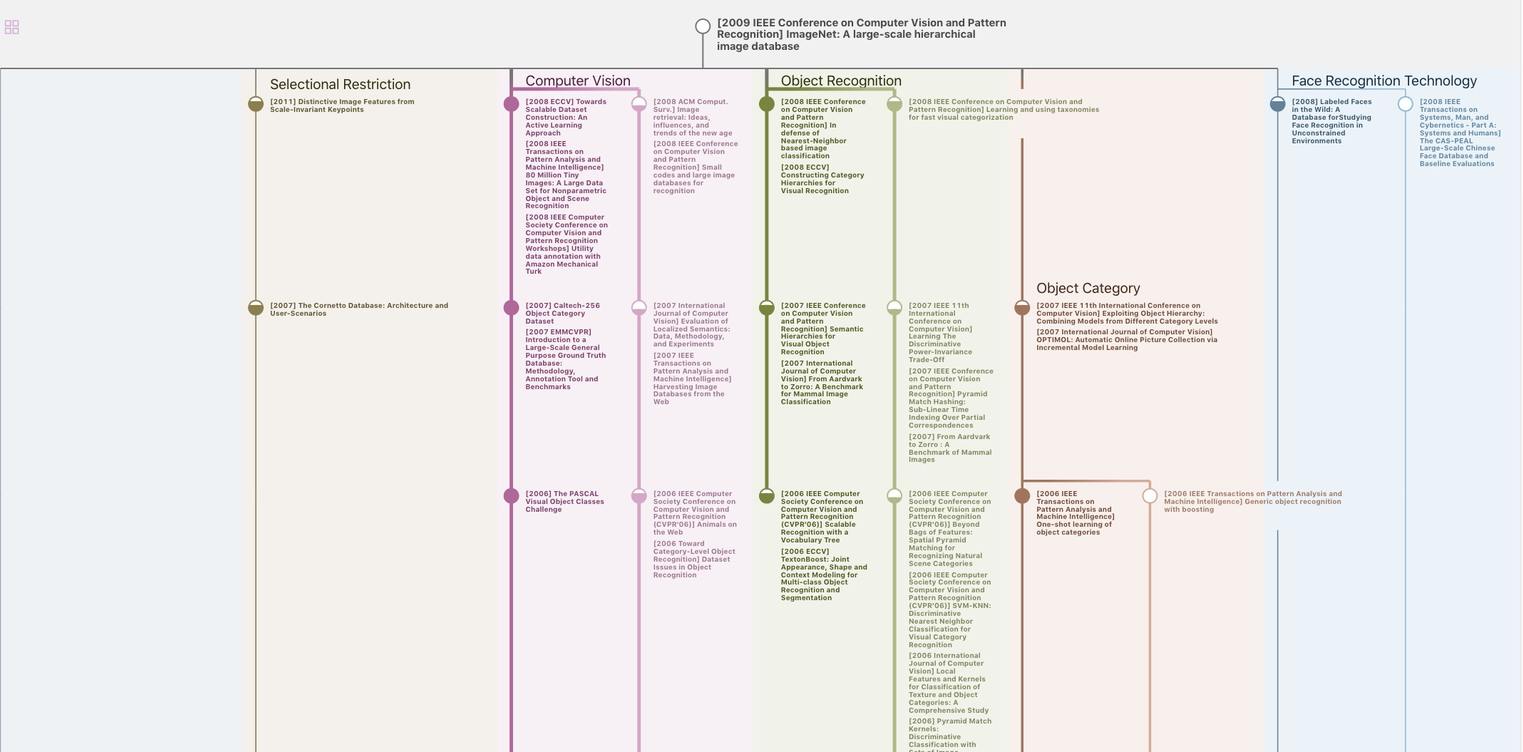
生成溯源树,研究论文发展脉络
Chat Paper
正在生成论文摘要