Predictive Analytics As A Service For Vehicle Health Monitoring Using Edge Computing And Ak-Nn Algorithm
Materials Today: Proceedings(2021)
摘要
Smart logistics is a part of Industry 4.0. With the increased development of the technology in the vehicle industry, the machine learning algorithms are applied on sensor data in order to detect the failure of the components of the vehicle. Several systems for vehicle health monitoring are presented in the literature for delivery of services in real-time. The sensor values obtained from the cloud are processed with machine learning algorithms, but have problem with delay in execution and data center failure. Edge computing is introduced in recent years so that intensive operations are performed at the edge of the device than at the cloud. This paper presents edge computing based fault prediction system that will predict vehicle health using internal and external sensors in real-time. Risk details are displayed through a mobile application in the form of notifications as well as a dashboard at the terminal. Such a system reduces latency between sending and processing vehicle data. The proposed system uses ensemble of ANN and k-NN classifiers named as AK-NN so as to improve prediction performance. In the first step, ANN is trained and validated on the Chevrolet car OBD dataset. Error reported from this best trained network is used by k-NN for statistical analysis of the error distributions. Three different experiments based on ANN, k-NN and AK-NN are made and evaluated using NRMSE, COD, cross entropy loss, accuracy and ROC measures. 85% accuracy for k-NN model when k = 3, 78% accuracy using ANN and 98.7% for ensemble method are achieved. Comparative study using key performance indicators such as Mean time between failure (MTBF) and Mean time to Repair (MTTR) is also made on 84 vehicles for prediction alert over mobile phone using analytical dashboard and proved to reach availability objective. (c) 2021 Elsevier Ltd. All rights reserved. Selection and peer-review under responsibility of the scientific committee of the 3rd International Conference on Materials, Manufacturing and Modelling.
更多查看译文
关键词
Edge Computing, k-Nearest Neighbor (k-NN), Artificial Neural Network (ANN), Vehicle data, Mobile application, Dynamic health monitoring, Predictive analytics
AI 理解论文
溯源树
样例
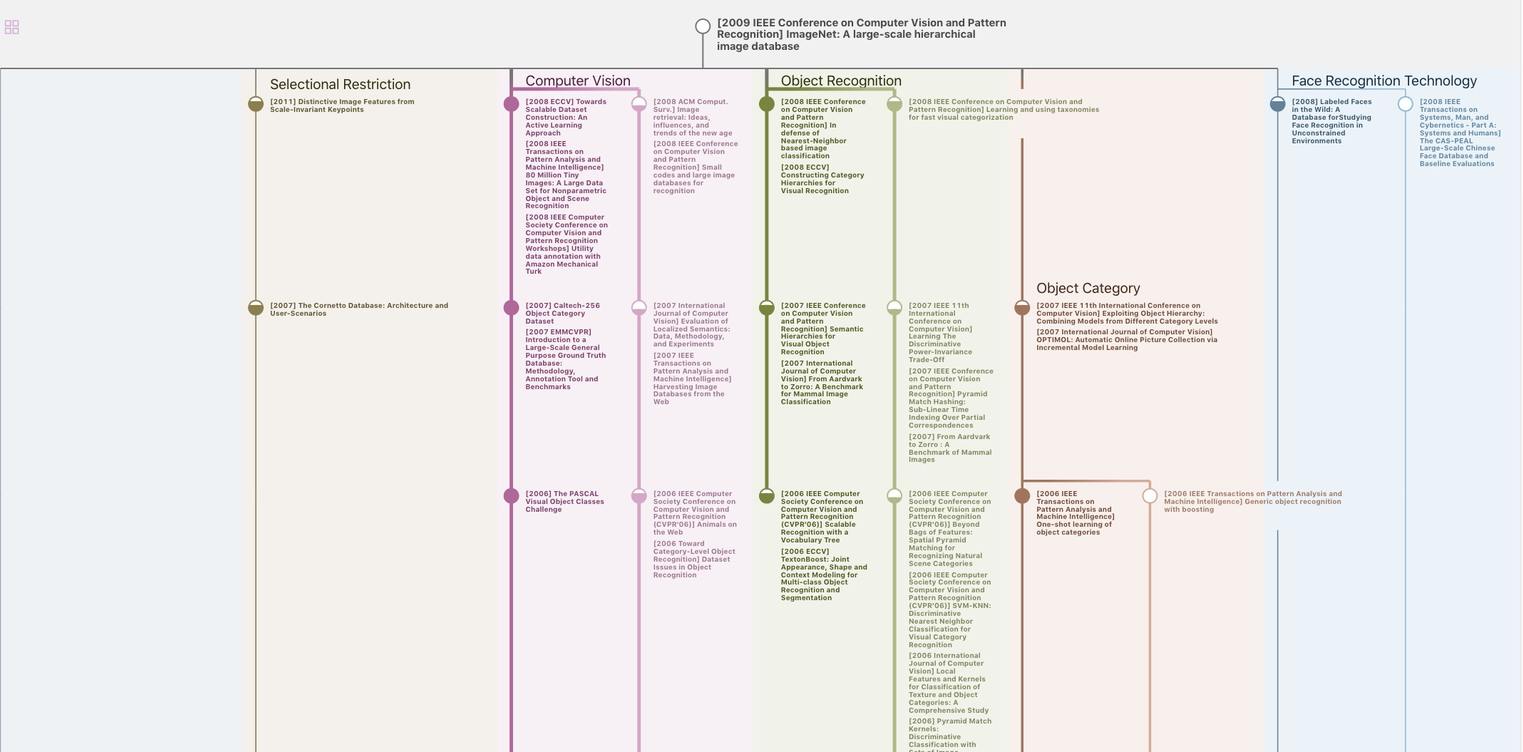
生成溯源树,研究论文发展脉络
Chat Paper
正在生成论文摘要