A Neural Network Quality-Control Scheme For Improved Quantitative Precipitation Estimation Accuracy On The Uk Weather Radar Network
JOURNAL OF ATMOSPHERIC AND OCEANIC TECHNOLOGY(2021)
摘要
In this work, we present a new quantitative precipitation estimation (QPE) quality-control (QC) algorithm for the U.K. weather radar network. The real-time adaptive algorithm uses a neural network (NN) to select data from the lowest useable elevation scan to optimize the combined performance of two other radar data correction algorithms: ground-clutter mitigation [using Clutter Environment Analysis using Adaptive Processing (CLEAN-AP)] and vertical profile of reflectivity (VPR) correction. The NN is trained using 3D tiles of observed uncontaminated weather signals that are systematically combined with ground-clutter signals collected under dry weather conditions. This approach provides a way to simulate radar signals with a wide range of clutter contamination conditions and with realistic spatial structures while providing the uncontaminated "truth" with respect to which the performance of the QC algorithm can be measured. An evaluation of QPE products obtained with the proposed QC algorithm demonstrates superior performance as compared to those obtained with the QC algorithm currently used in operations. Similar improvements are also illustrated using radar observations from two periods of prolonged precipitation, showing a better balance between overestimation errors from using clutter-contaminated low-elevation radar data and VPR-induced errors from using high-elevation radar data.
更多查看译文
关键词
Precipitation, Data quality control, Weather radar signal processing, Artificial intelligence
AI 理解论文
溯源树
样例
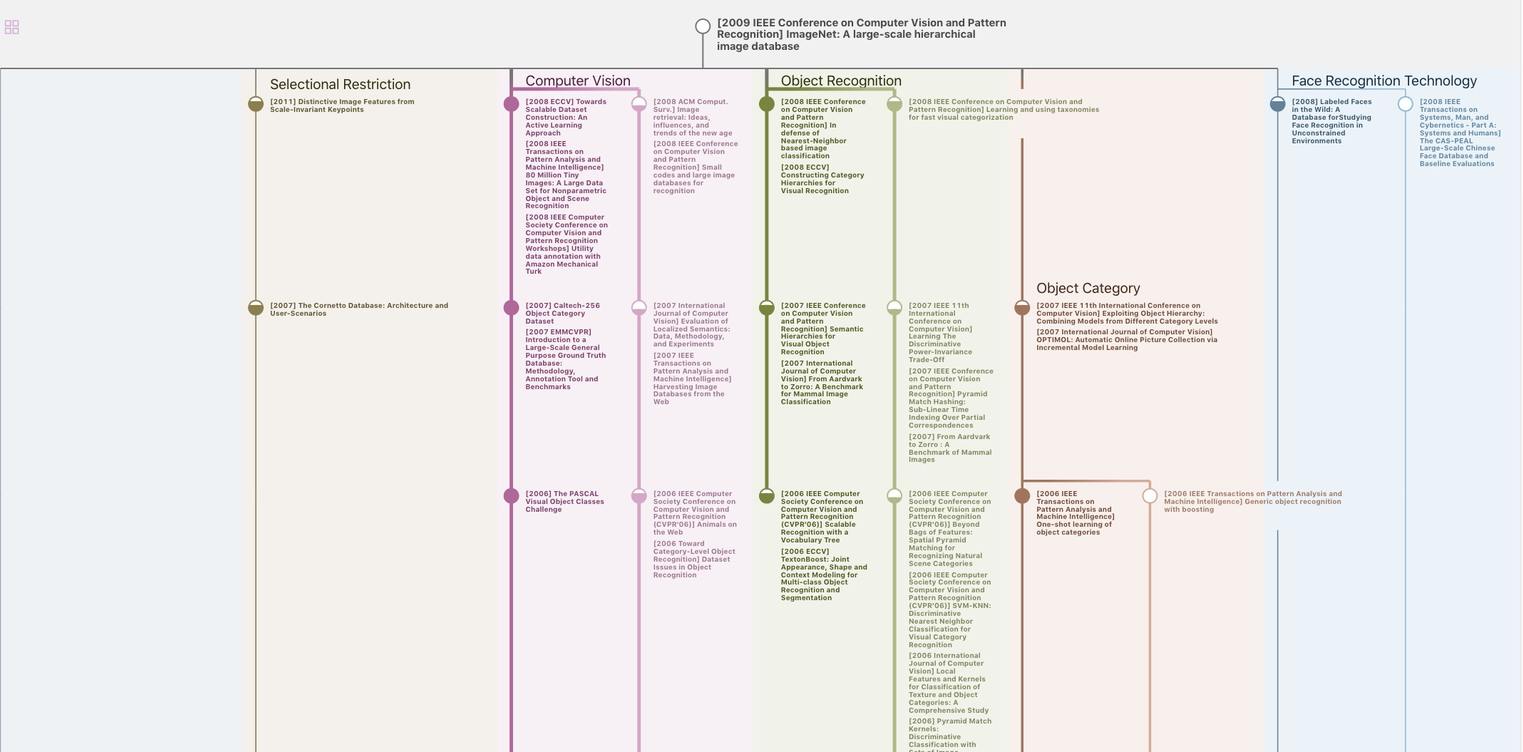
生成溯源树,研究论文发展脉络
Chat Paper
正在生成论文摘要