Geo-Spatial Market Segmentation & Characterization Exploiting User Generated Text Through Transformers & Density-Based Clustering
IEEE ACCESS(2021)
摘要
In data analysis, context information plays a significant role in enhancing the quality of the insight obtained. Furthermore, spatial analysis helps understand spatial relationships among entities. Nevertheless, findings of a comprehensive literature review show that the characterization of geographic areas based on user generated content, such as text messages, has not been sufficiently explored. This paper focuses on investigating how to combine and exploit geographic information with user generated text content to detect geographic clusters of textual events, and infer relationships between each cluster and a fixed set of retail product categories, which we consider as an insightful way to perform spatial market segmentation. We propose a workflow composed of several machine learning models incorporating Transformers as an attention mechanism and BERT-based data augmentation capable of predicting product classes from Amazon product reviews and Twitter message corpora, and then characterizing the obtained geographic clusters based on their aggregated scores. The output of our system is an effective visualization of the geographic areas with their corresponding relevance score against a fixed set of categories. We trained a product document classifier achieving an F1-Score of 86% in the test set for product reviews, and of 76% in the test set for tweets; and validated our approach by manually annotating a subset of Twitter data with respect to ten product categories. Our approach provides practitioners with a mechanism to combine location context, a Transformer encoder, and transfer learning to derive insights from geo-spatial and text data; and researchers with opportunities to continue advancing the field.
更多查看译文
关键词
Social networking (online), Blogs, Electronic mail, Advertising, User-generated content, Data models, Transfer learning, Advertising, context awareness, machine learning, natural language processing, clustering algorithms, transformers
AI 理解论文
溯源树
样例
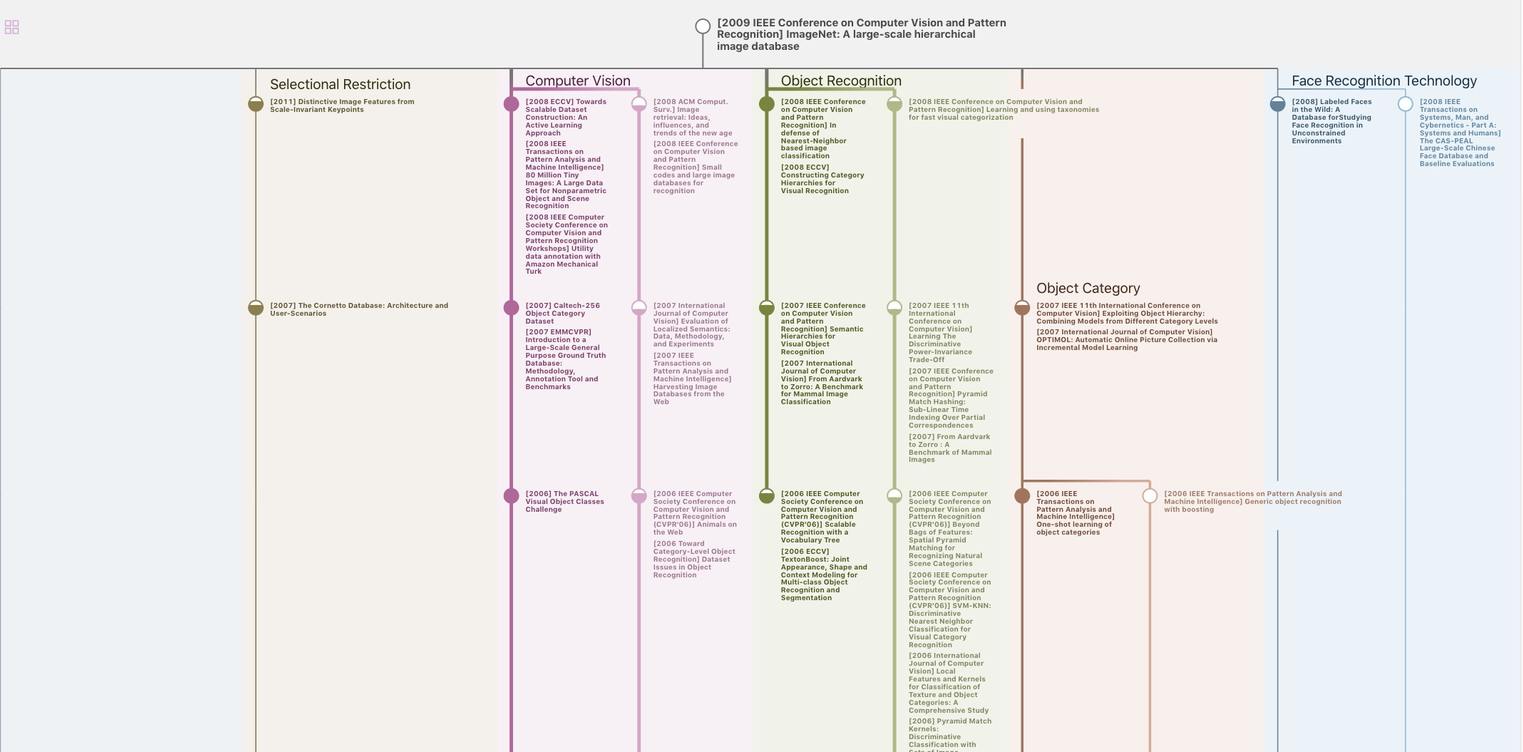
生成溯源树,研究论文发展脉络
Chat Paper
正在生成论文摘要