SODA: Similar 3D Object Detection Accelerator at Network Edge for Autonomous Driving
IEEE INFOCOM 2021 - IEEE Conference on Computer Communications(2021)
摘要
Offloading the 3D object detection from autonomous vehicles to MEC is appealing because of the gains on quality, latency, and energy. However, detection requests lead to repetitive computations since the multitudinous requests share approximate detection results. It is crucial to reduce such fuzzy redundancy by reusing the previous results. A key challenge is that the requests mapping to the reusable result are only similar but not identical. An efficient method for similarity matching is needed to justify the use case. To this end, by taking advantage of TCAM's ap-proximate matching capability and NMC's computing efficiency, we design SODA, a first-of-its-kind hardware accelerator which sits in the mobile base stations between autonomous vehicles and MEC servers. We design efficient feature encoding and partition algorithms for SODA to ensure the quality of the similarity matching and result reuse. Our evaluation shows that SODA significantly improves the system performance and the detection results exceed the accuracy requirements on the subject matter, qualifying SODA as a practical domain-specific solution.
更多查看译文
关键词
autonomous vehicles,fuzzy redundancy,SODA,hardware accelerator,MEC servers,partition algorithms,similar 3D object detection accelerator,network edge,autonomous driving,mobile base stations,feature encoding,similarity matching quality,mobile edge computing
AI 理解论文
溯源树
样例
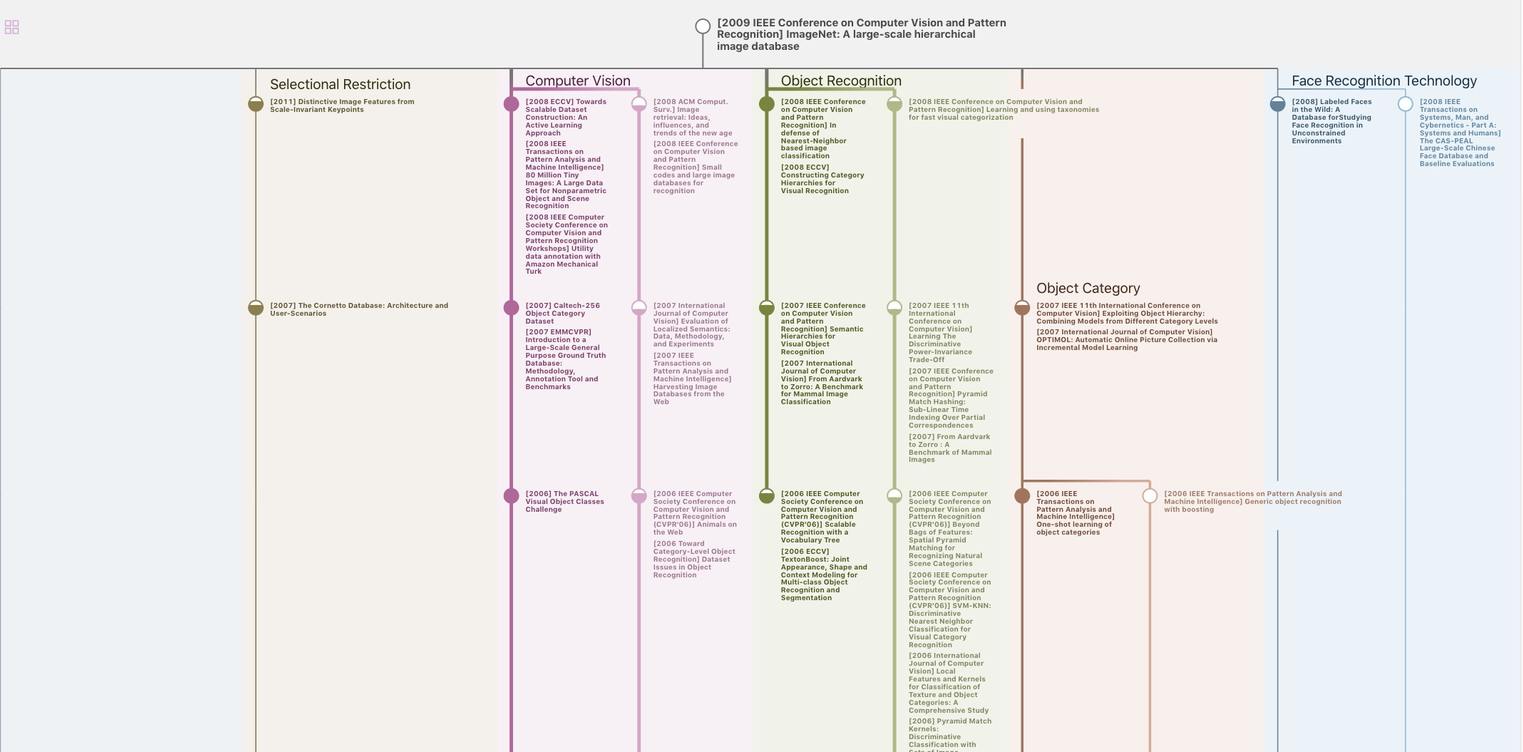
生成溯源树,研究论文发展脉络
Chat Paper
正在生成论文摘要