Spatial Interpretability Of Time-Frequency Relevance Optimized In Motor Imagery Discrimination Using Deep&Wide Networks
BIOMEDICAL SIGNAL PROCESSING AND CONTROL(2021)
摘要
Medical diagnosis and monitoring benefit from exploiting the advantages of motor imagery (MI) training, which highly depends on the proper interpretation of elicited brain activity responses. Convolutional neural networks (CNN) are increasingly used to improve MI classification performance by employing multi-view extracted features (time, frequency, and spatial). However, deep learning gains knowledge from abundant, complex neural models, resulting in poor interpretability assessments. Here, to enhance the understanding of imagined actions, we develop the relevance analysis of topographic time-frequency representation, preserving an adequate classification performance. Namely, to deal better with the subject variability, a 2D feature combination of continuous wavelet transform and common spatial patterns is extracted to feed a Deep&Wide learning model, assessing the relevance of input multi-view representation that contribute the best to the classifier accuracy. We estimate the feature contribution through the information back-propagated across the hidden layers' weights before predicting the output label. Evaluation, presented in two databases with bi- and four-label MI tasks, proves that the developed Deep&Wide-based relevance analysis reveals insights about the electrodes, time segments, and frequency bands with relevant MI neural responses, favoring explanation of inter and intra-subject variability because of coordination skills.
更多查看译文
关键词
Motor imagery, Time-frequency, Spatial relevance, Deep&, Wide network
AI 理解论文
溯源树
样例
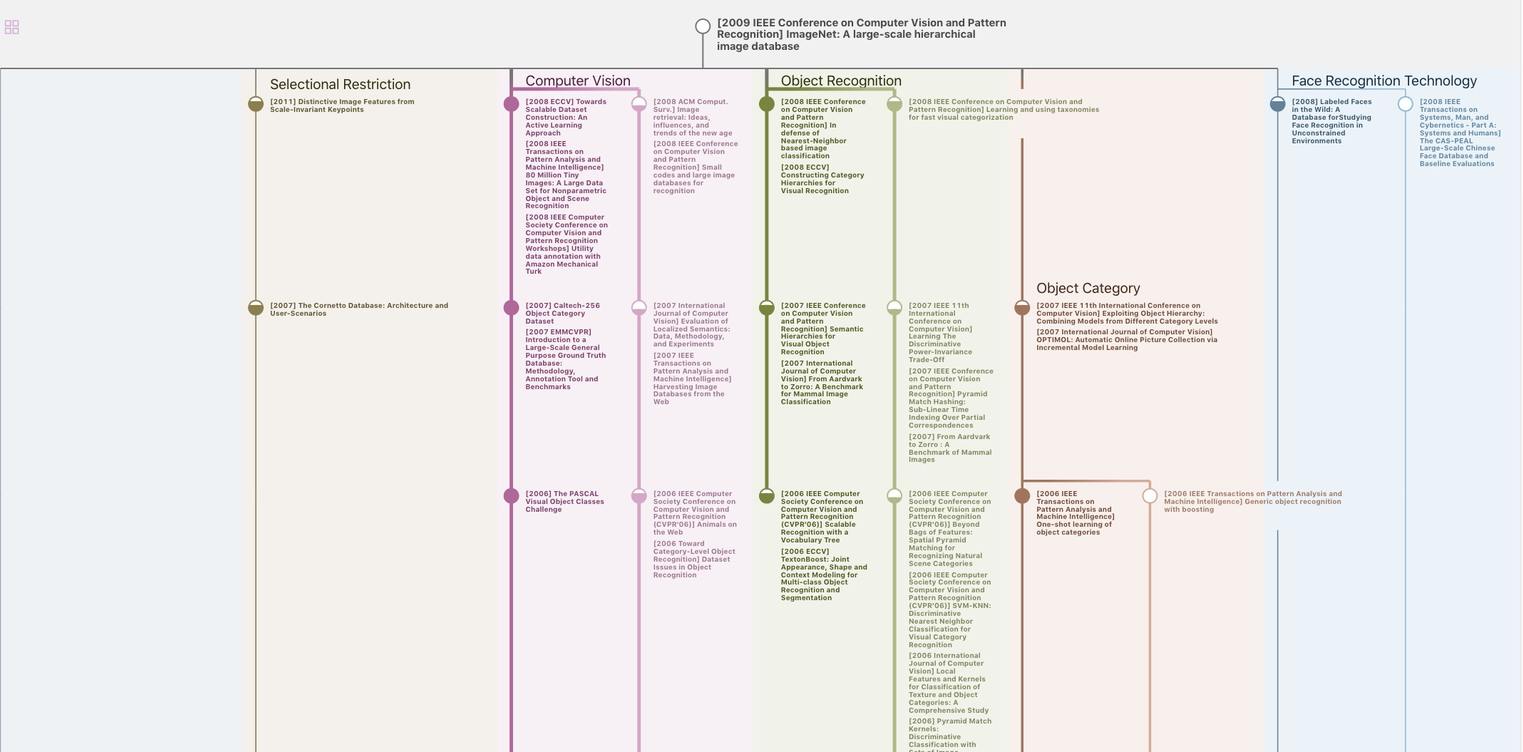
生成溯源树,研究论文发展脉络
Chat Paper
正在生成论文摘要