Franken-Ct: Head And Neck Mr-Based Pseudo-Ct Synthesis Using Diverse Anatomical Overlapping Mr-Ct Scans
APPLIED SCIENCES-BASEL(2021)
摘要
Featured ApplicationThe Franken-CT approach allows synthesizing pseudo-CT images from using diverse anatomical overlapping MR-CT datasets as a potential application in PET/MR attenuation correction.Typically, pseudo-Computerized Tomography (CT) synthesis schemes proposed in the literature rely on complete atlases acquired with the same field of view (FOV) as the input volume. However, clinical CTs are usually acquired in a reduced FOV to decrease patient ionization. In this work, we present the Franken-CT approach, showing how the use of a non-parametric atlas composed of diverse anatomical overlapping Magnetic Resonance (MR)-CT scans and deep learning methods based on the U-net architecture enable synthesizing extended head and neck pseudo-CTs. Visual inspection of the results shows the high quality of the pseudo-CT and the robustness of the method, which is able to capture the details of the bone contours despite synthesizing the resulting image from knowledge obtained from images acquired with a completely different FOV. The experimental Zero-Normalized Cross-Correlation (ZNCC) reports 0.9367 +/- 0.0138 (mean +/- SD) and 95% confidence interval (0.9221, 0.9512); the experimental Mean Absolute Error (MAE) reports 73.9149 +/- 9.2101 HU and 95% confidence interval (66.3383, 81.4915); the Structural Similarity Index Measure (SSIM) reports 0.9943 +/- 0.0009 and 95% confidence interval (0.9935, 0.9951); and the experimental Dice coefficient for bone tissue reports 0.7051 +/- 0.1126 and 95% confidence interval (0.6125, 0.7977). The voxel-by-voxel correlation plot shows an excellent correlation between pseudo-CT and ground-truth CT Hounsfield Units (m = 0.87; adjusted R-2 = 0.91; p < 0.001). The Bland-Altman plot shows that the average of the differences is low (-38.6471 +/- 199.6100; 95% CI (-429.8827, 352.5884)). This work serves as a proof of concept to demonstrate the great potential of deep learning methods for pseudo-CT synthesis and their great potential using real clinical datasets.
更多查看译文
关键词
deep learning, image synthesis, PET, MR, pseudo-CT
AI 理解论文
溯源树
样例
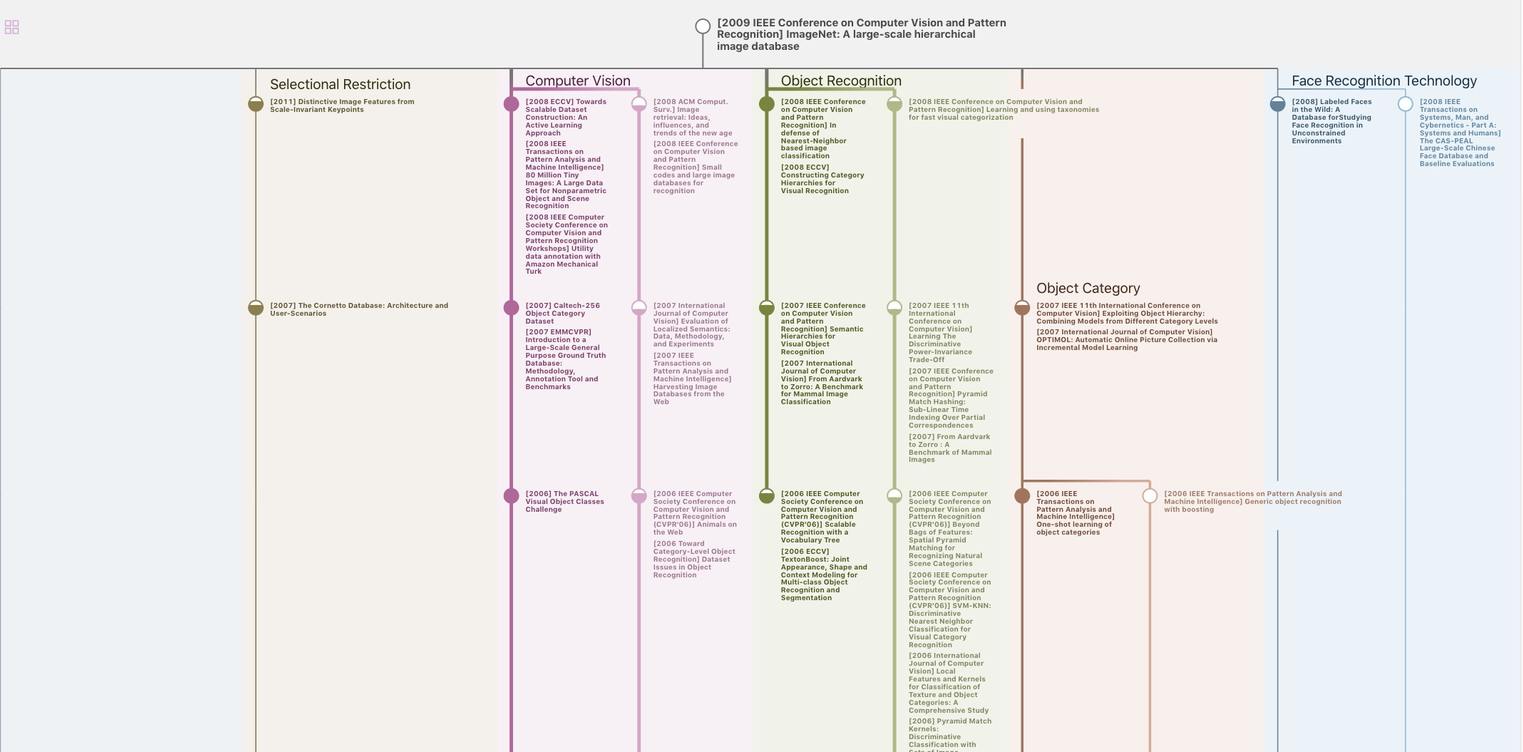
生成溯源树,研究论文发展脉络
Chat Paper
正在生成论文摘要