Empirical Analysis of Policy Gradient Algorithms where Starting States are Sampled accordingly to Most Frequently Visited States
IFAC-PapersOnLine(2020)
摘要
Abstract In this paper, we propose an extension to the policy gradient algorithms by allowing starting states to be sampled from a probability distribution that may differ from the one used to specify the reinforcement learning task. In particular, we suggest that, between policy updates, starting states should be sampled from a probability density function which approximates the state visitation frequency of the current policy. Results generated from various environments clearly demonstrate a performance improvement in terms of mean cumulative rewards and substantial update stability compared to vanilla policy gradient algorithms where the starting state distributions are either as specified by the environment or uniform distributions over the state space. A sensitivity analysis over a subset of the hyper-parameters of our algorithm also suggests that they should be adapted after each policy update to maximise the improvements of the policies.
更多查看译文
关键词
Reinforcement learning control
AI 理解论文
溯源树
样例
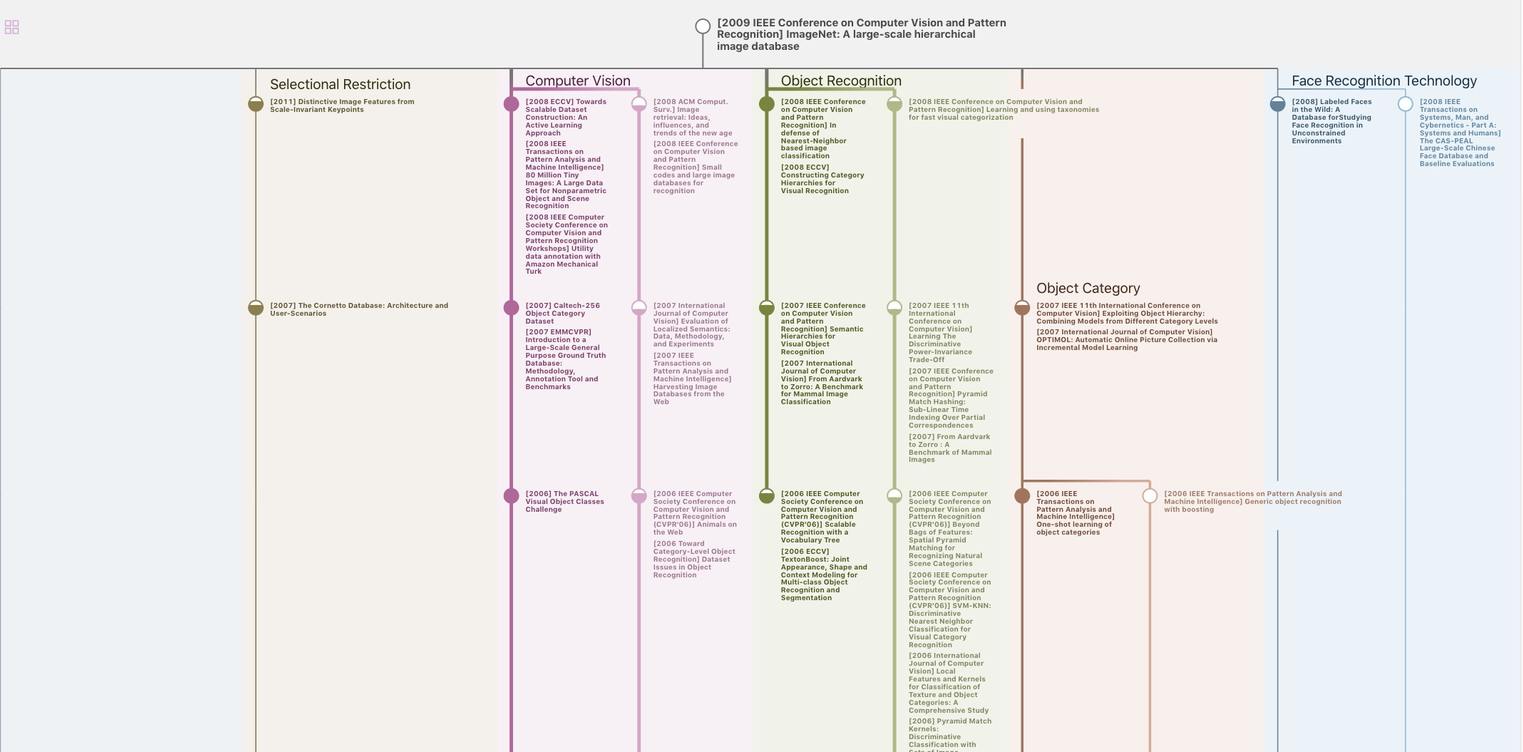
生成溯源树,研究论文发展脉络
Chat Paper
正在生成论文摘要