A new feature selection metric for text classification: eliminating the need for a separate pruning stage
INTERNATIONAL JOURNAL OF MACHINE LEARNING AND CYBERNETICS(2021)
摘要
Terms that occur too frequently or rarely in various texts are not useful for text classification. Pruning can be used to remove such irrelevant terms reducing the dimensionality of the feature space and, thus making feature selection more efficient and effective. Normally, pruning is achieved by manually setting threshold values. However, incorrect threshold values can result in the loss of many useful terms or retention of irrelevant ones. Existing feature ranking metrics can assign higher ranks to these irrelevant terms, thus degrading the performance of a text classifier. In this paper, we propose a new feature ranking metric, which can select the most useful terms in the presence of these too frequently and rarely occurring terms, thus eliminating the need for pruning these terms. To investigate the usefulness of the proposed metric, we compare it against seven well-known feature selection metrics on five data sets namely Reuters-21578 (re0, re1, r8) and WebACE (k1a, k1b) using multinomial naive Bayes and support vector machines classifiers. Our results based on a paired t-test show that the performance of our metric is statistically significant than that of the other seven metrics.
更多查看译文
关键词
Text classification, Feature selection, Feature ranking metrics, Pruning
AI 理解论文
溯源树
样例
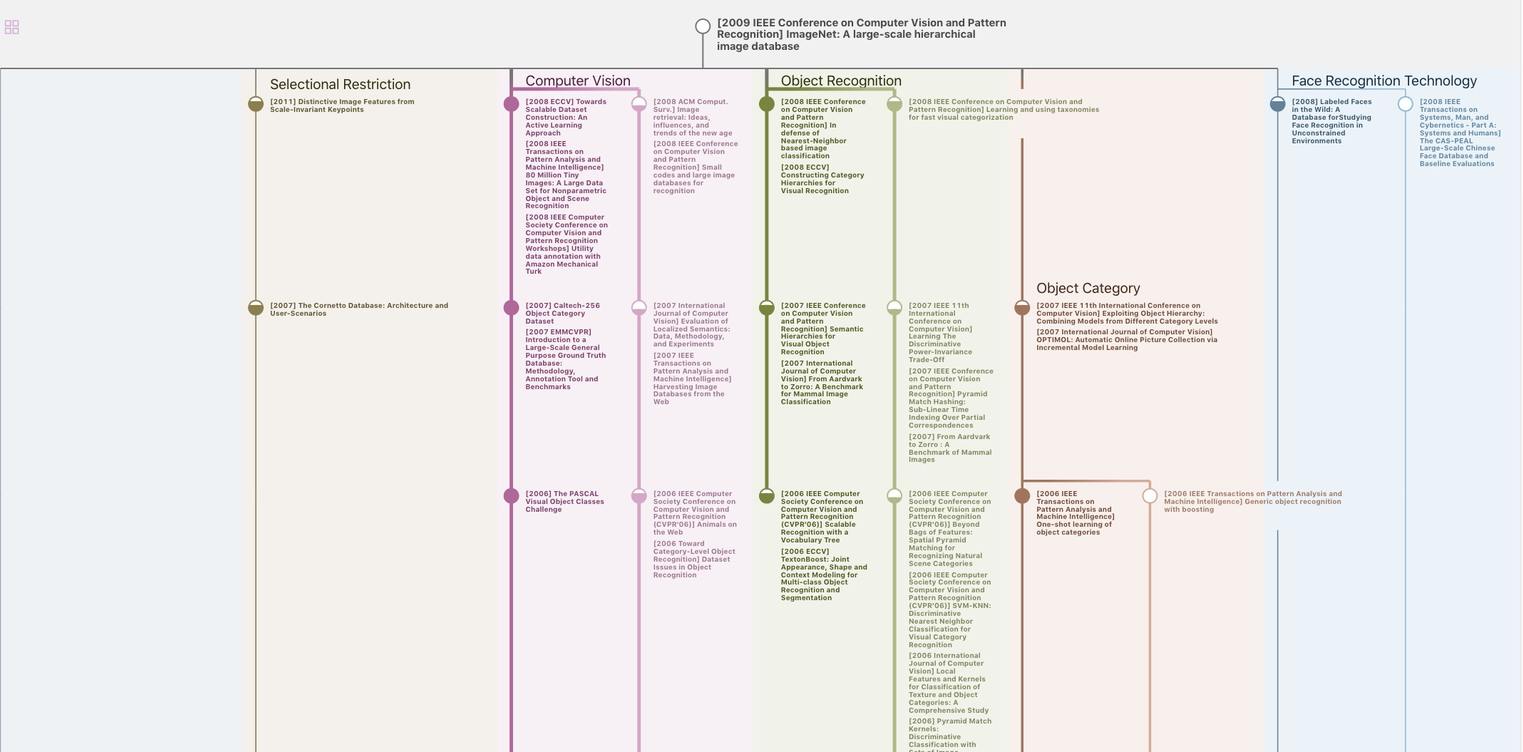
生成溯源树,研究论文发展脉络
Chat Paper
正在生成论文摘要