Enhancing social recommendation via two-level graph attentional networks.
Neurocomputing(2021)
摘要
As an effective deep representation learning technique for graph data, graph convolutional network (GCN) has recently been widely applied to obtain better embedding of vertex. The existing studies have successfully explored user-item interaction via GCN for recommendation task and proved its effectiveness. We argue that a significant limitation of these methods is that social relation, which has been proven to impose positive effects for recommendation in many loss optimization models, has received relatively little scrutiny in GCN. Thus, the resultant embeddings are insufficient to model the potential social propagation effect.In our work, we propose to obtain embeddings by using the neighborhood propagation mechanism on two coupled graphs, i.e. user-item interaction graph and social relation graph, which is capable of capturing the interplay between the user’s item taste and user’s friend relationship to integrate social effect into the embeddings. In particular, to address the challenge that different factors on neighborhood propagation process make different contributions for the embedding, we develop Attentional Social Recommendation system (ASR), a new social recommendation framework with hierarchical attention(i.e., neighbor-level and graph-level attention). This allows much flexibility for adaptively acquiring relative importance for different factors. Extensive experiment on three real-world datasets not only show the superior performance of our proposed model over the baselines, but also demonstrate the effectiveness of simultaneous neighborhood propagation on two graphs.
更多查看译文
关键词
Social relation,Recommender system,Two-level attentional mechanism,Graph convolutional network
AI 理解论文
溯源树
样例
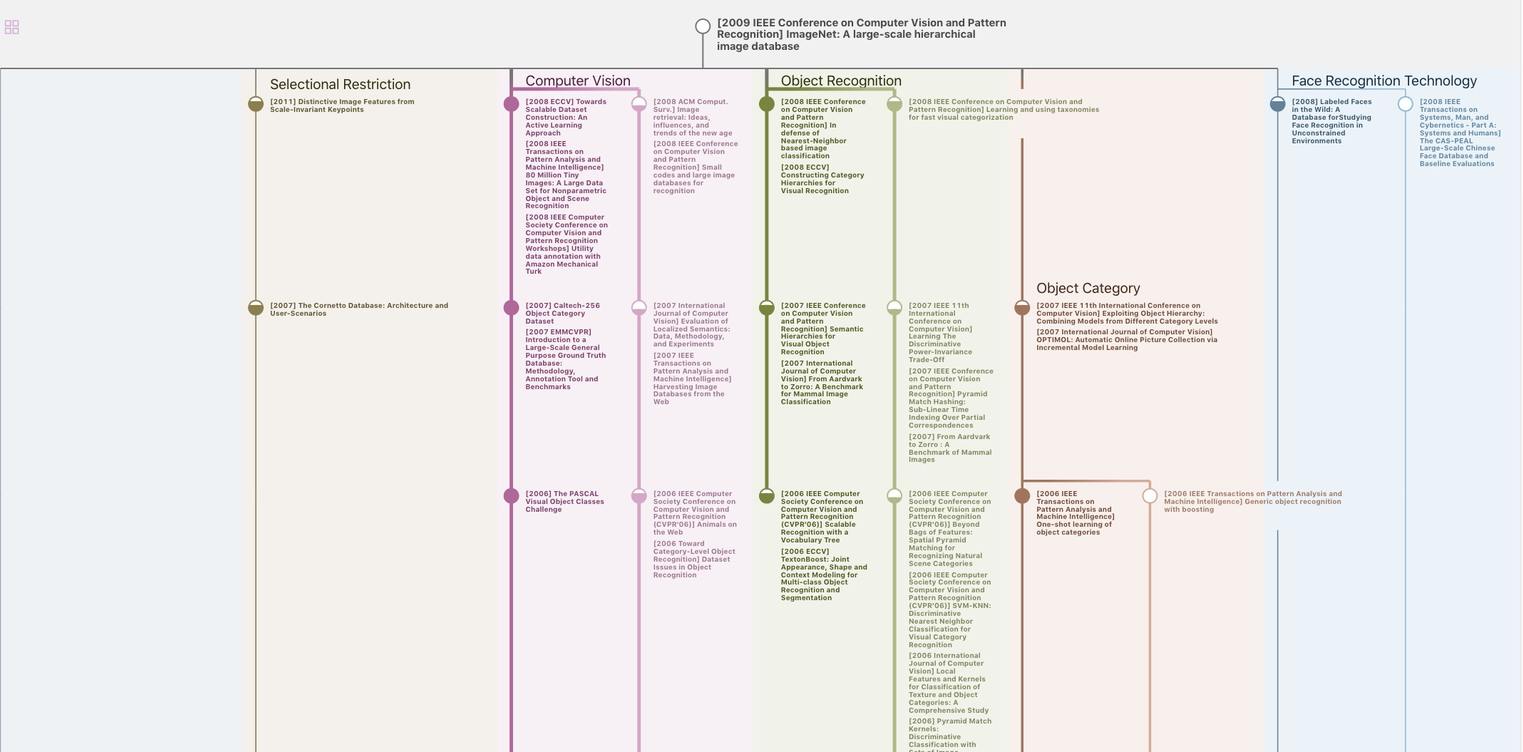
生成溯源树,研究论文发展脉络
Chat Paper
正在生成论文摘要