Automated Asphalt Pavement Raveling Detection and Classification using Convolutional Neural Network and Macrotexture Analysis
TRANSPORTATION RESEARCH RECORD(2021)
摘要
Raveling is one of the most common asphalt pavement distresses. The survey of its condition is required for transportation agencies to ensure roadway safety and appropriately apply preservation and rehabilitation treatments. However, the traditional raveling condition survey, including the determination of the raveling severity, is typically manually conducted by in-field visual inspection methods that are time consuming, labor intensive, and error prone. Although automated raveling detection and severity classification models have been developed, these existing models have shortcomings. Therefore, there is an urgent need to develop a more accurate and reliable model to automatically detect and classify raveling. This study proposes the first convolutional neural network (CNN)-based model for automated raveling detection and classification. Compared with general CNNs, the proposed model combines the data-driven features learned from training data and macrotexture features of 3D pavement surface data to achieve better performance. The proposed model was evaluated and compared with existing machine learning models using real-world 3D pavement surface data collected from the state of Georgia, U.S. By combining data-driven features with macrotexture features, the proposed model achieved the highest accuracy of 90.8% on raveling classification. The proposed model also achieved classification precision and recall higher than 85% for all raveling severity levels, which is more accurate and robust than existing models. It is concluded that, with multi-type features extraction and proper model design, the proposed model can provide more accurate and reliable predictions for raveling detection and classification.
更多查看译文
AI 理解论文
溯源树
样例
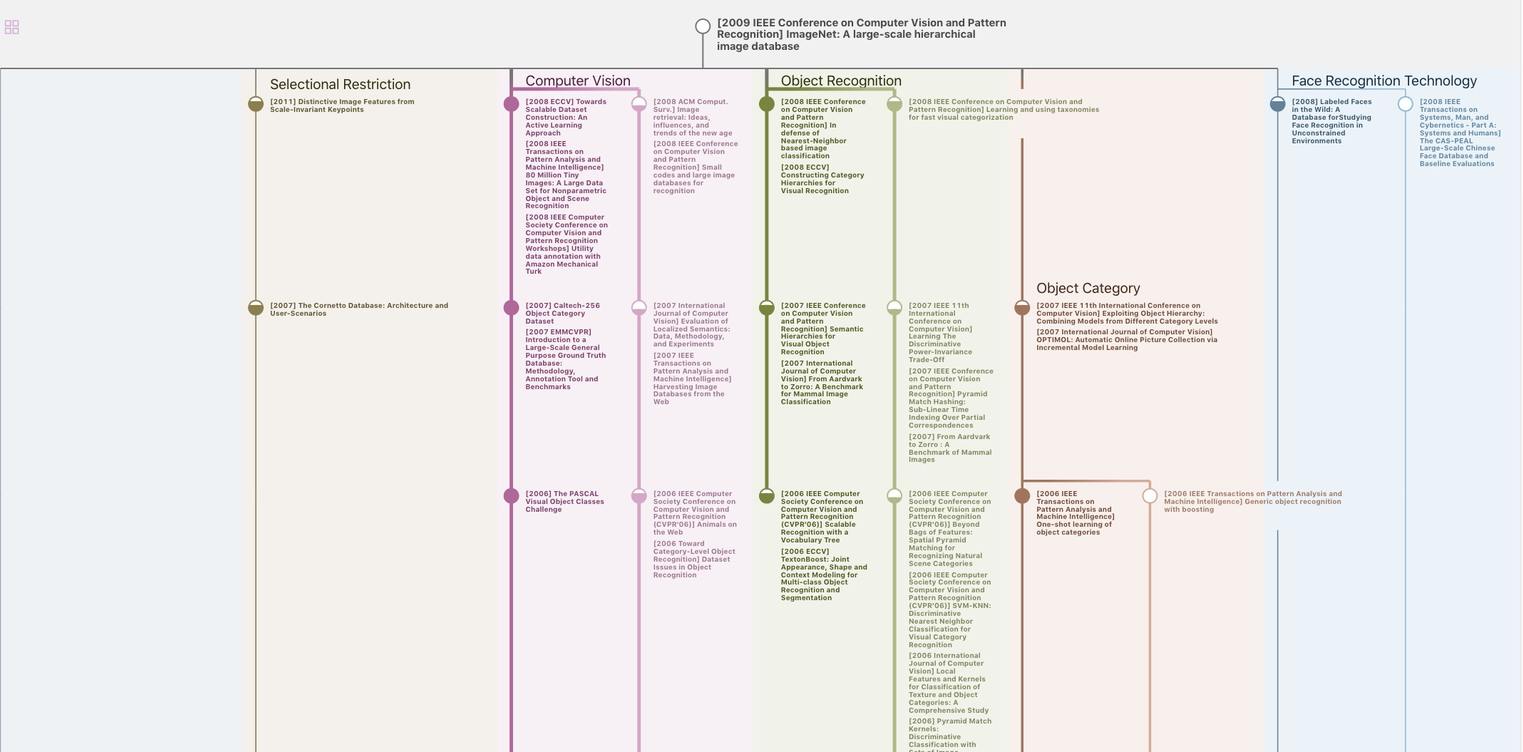
生成溯源树,研究论文发展脉络
Chat Paper
正在生成论文摘要