Egfr Assessment In Lung Cancer Ct Images: Analysis Of Local And Holistic Regions Of Interest Using Deep Unsupervised Transfer Learning
IEEE ACCESS(2021)
摘要
Statistics have demonstrated that one of the main factors responsible for the high mortality rate related to lung cancer is the late diagnosis. Precision medicine practices have shown advances in the individualized treatment according to the genetic profile of each patient, providing better control on cancer response. Medical imaging offers valuable information with an extensive perspective of the cancer, opening opportunities to explore the imaging manifestations associated with the tumor genotype in a non-invasive way. This work aims to study the relevance of physiological features captured from Computed Tomography images, using three different 2D regions of interest to assess the Epidermal growth factor receptor (EGFR) mutation status: nodule, lung containing the main nodule, and both lungs. A Convolutional Autoencoder was developed for the reconstruction of the input image. Thereafter, the encoder block was used as a feature extractor, stacking a classifier on top to assess the EGFR mutation status. Results showed that extending the analysis beyond the local nodule allowed the capture of more relevant information, suggesting the presence of useful biomarkers using the lung with nodule region of interest, which allowed to obtain the best prediction ability. This comparative study represents an innovative approach for gene mutations status assessment, contributing to the discussion on the extent of pathological phenomena associated with cancer development, and its contribution to more accurate Artificial Intelligence-based solutions, and constituting, to the best of our knowledge, the first deep learning approach that explores a comprehensive analysis for the EGFR mutation status classification.
更多查看译文
关键词
Computed tomography, Lung, Feature extraction, Lung cancer, Transfer learning, Databases, Tumors, Convolutional autoencoder, EGFR prediction, lung cancer, transfer learning, unsupervised feature learning
AI 理解论文
溯源树
样例
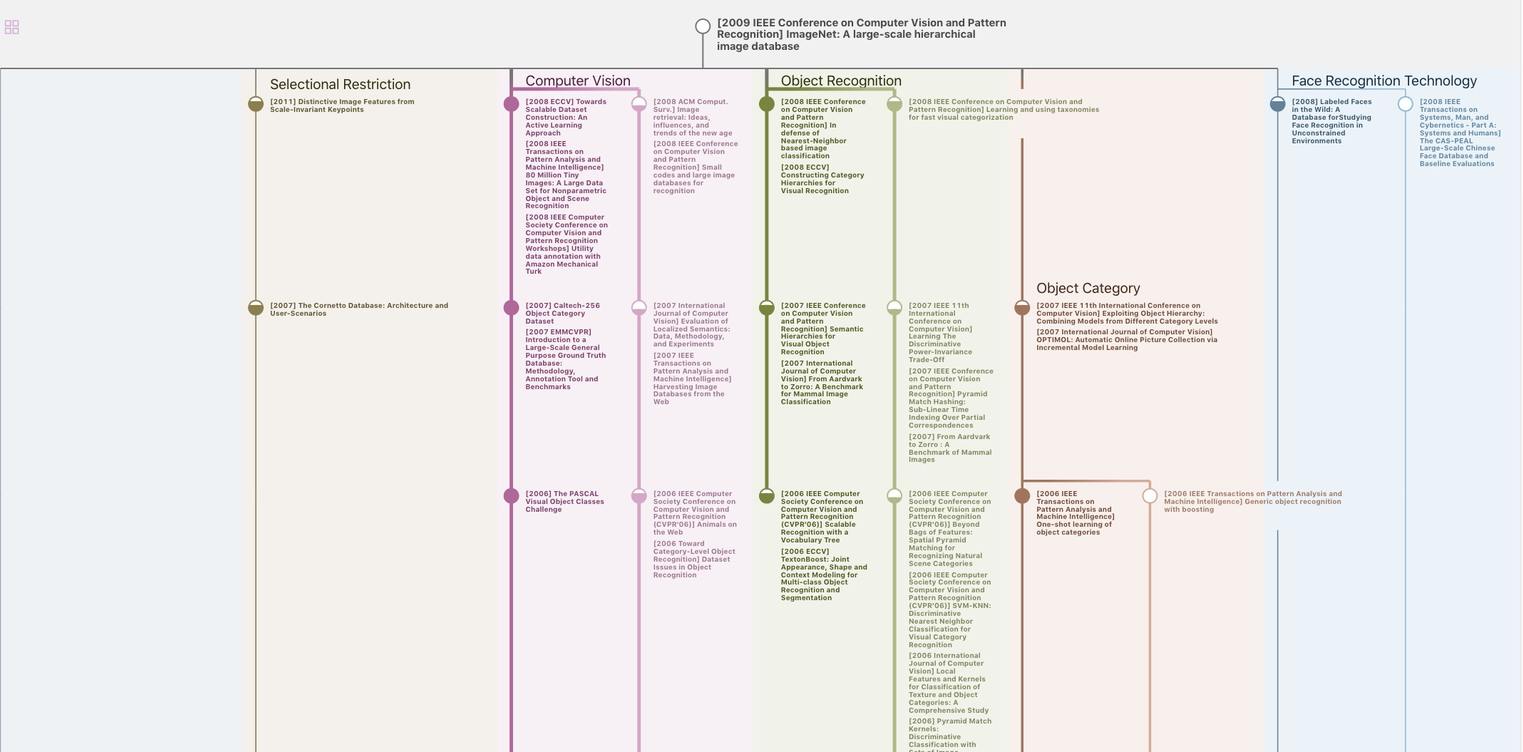
生成溯源树,研究论文发展脉络
Chat Paper
正在生成论文摘要