FGCNet: Fast Graph Convolution for Matching Features
2022 IEEE International Symposium on Mixed and Augmented Reality Adjunct (ISMAR-Adjunct)(2022)
摘要
This paper proposes a fast graph convolution network (FGCNet) to match two sets of sparse features. FGCNet has three new modules connected in sequence: (i) a local graph convolution block takes point-wise features as inputs and encodes local contextual infor-mation to extract local features; (ii) a fast graph message-passing network takes local features as inputs, encodes two-view global contextual information, to improve the discriminativeness of point-wise features; (iii) a preemptive optimal matching layer takes point-wise features as inputs, regress point-wise matchedness scores and es-timate a 2D joint probability matrix, with each item describes the matchedness of a feature correspondence. We validate the proposed method on three AR/VR related tasks: two-view matching, 3D re-construction and visual localization. Experiments show that our method significantly reduces the computational complexity compared with state-of-the-art methods, while achieving competitive or better performance.
更多查看译文
关键词
Two-view matching-3D reconstruction-Visual localization-Graph convolution
AI 理解论文
溯源树
样例
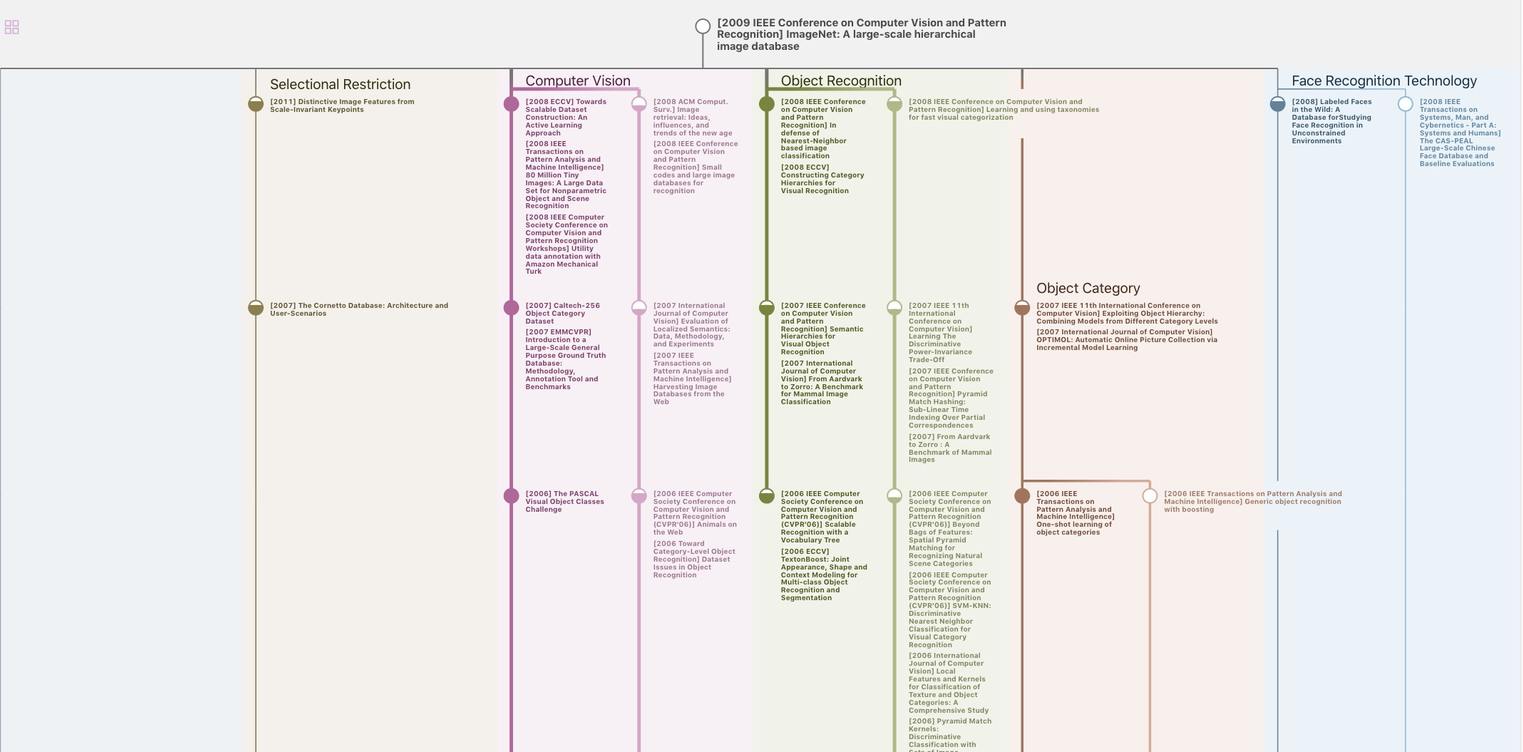
生成溯源树,研究论文发展脉络
Chat Paper
正在生成论文摘要