A self-adaptive particle swarm optimization based K-means (SAPSO-K) clustering method to evaluate fabric tactile comfort
JOURNAL OF THE TEXTILE INSTITUTE(2022)
摘要
This article proposes a new clustering algorithm of the self-adaptive particle swarm optimization (PSO) based K-means (SAPSO-K) to evaluate the fabric tactile comfort and compared with other algorithms. In this study, the efficient characterization of Quick-Intelligent Handle Evaluation System (QIHES) method was used to detect the mechanical properties, where 10 feature indexes were extracted from the testing force-displacement curves. The correlation analysis was used to determine the appropriate clustering data set and the different number of groups was applied to determine the optimal cluster number. Moreover, subjective evaluation was conducted and compared with the conventional clustering algorithms of K-means, agglomerative hierarchical clustering (AHC), PSO-based K-means(PSO-K) and SAPSO-K. The results show that the clustering results based on 10 indexes are significantly better than the eight indexes with good correlation, which indicate the performance of the stretching stage has a certain degree of influence on the fabric tactile comfort. The PSO-K and SAPSO-K algorithms have the minimum average relative error compared to the subjective rating values (RV) as 4.455 with the optimal cluster number as 5. Nevertheless, SAPSO-K achieved the best fitness of 6.192 after eight iterations, while PSO-K needs 136 times. Therefore, the SAPSO-K clustering algorithm is the most effective and reliable method to predict the RV and evaluate the tactile comfort with good accuracy.
更多查看译文
关键词
Tactile comfort, SAPSO-K, QIHES, clustering algorithms, PSO-K
AI 理解论文
溯源树
样例
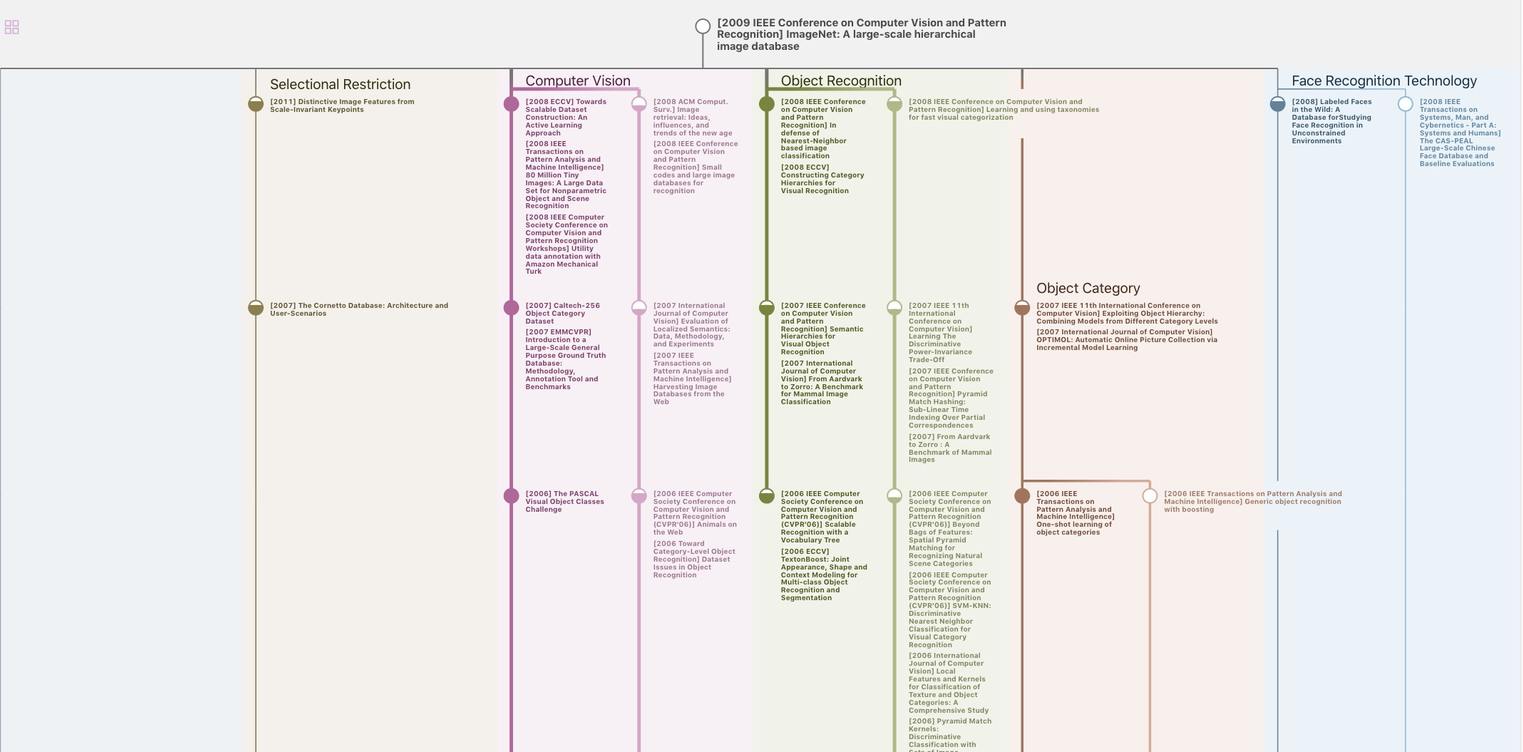
生成溯源树,研究论文发展脉络
Chat Paper
正在生成论文摘要