Community Detection In Large-Scale Bipartite Biological Networks
FRONTIERS IN GENETICS(2021)
摘要
Networks are useful tools to represent and analyze interactions on a large, or genome-wide scale and have therefore been widely used in biology. Many biological networks-such as those that represent regulatory interactions, drug-gene, or gene-disease associations-are of a bipartite nature, meaning they consist of two different types of nodes, with connections only forming between the different node sets. Analysis of such networks requires methodologies that are specifically designed to handle their bipartite nature. Community structure detection is a method used to identify clusters of nodes in a network. This approach is especially helpful in large-scale biological network analysis, as it can find structure in networks that often resemble a "hairball" of interactions in visualizations. Often, the communities identified in biological networks are enriched for specific biological processes and thus allow one to assign drugs, regulatory molecules, or diseases to such processes. In addition, comparison of community structures between different biological conditions can help to identify how network rewiring may lead to tissue development or disease, for example. In this mini review, we give a theoretical basis of different methods that can be applied to detect communities in bipartite biological networks. We introduce and discuss different scores that can be used to assess the quality of these community structures. We then apply a wide range of methods to a drug-gene interaction network to highlight the strengths and weaknesses of these methods in their application to large-scale, bipartite biological networks.
更多查看译文
关键词
networks, genomic networks, community detection algorithms, community detection analysis, genomic data analysis, network analysis, biological network analysis, biological network clustering
AI 理解论文
溯源树
样例
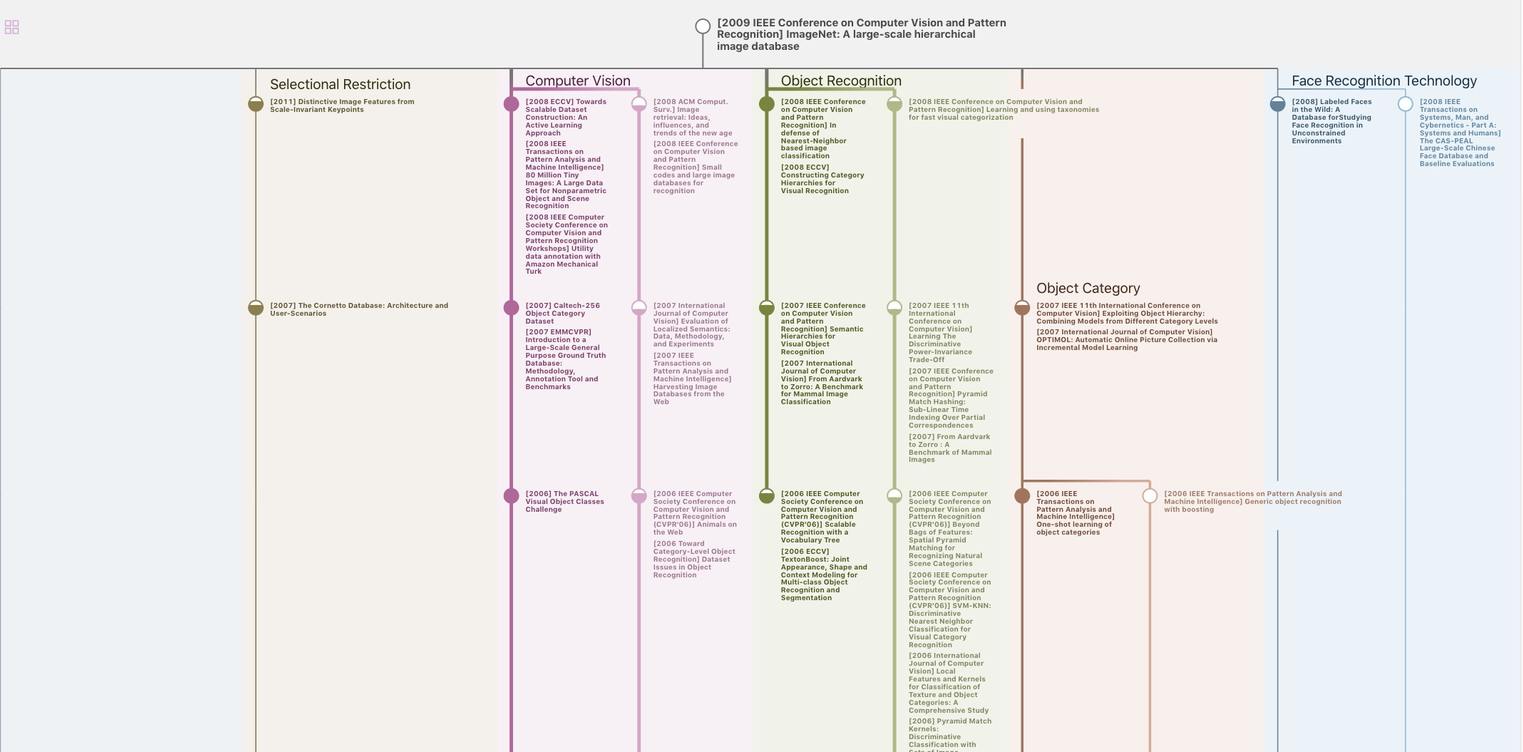
生成溯源树,研究论文发展脉络
Chat Paper
正在生成论文摘要