An Efficient Learning Algorithm for Direct Training Deep Spiking Neural Networks
IEEE Transactions on Cognitive and Developmental Systems(2022)
摘要
It is challenging to train deep spiking neural networks (SNNs) directly due to the difficulties associated with the nondifferentiable neuron model. In this work, an end-to-end learning algorithm based on discrete current-based leaky integrate-and-fire (C-LIF) neuron model and surrogate gradient is proposed to leverage the encoder–decoder network architecture to train deep SNNs directly. The proposed algorithm is capable of learning deep spatiotemporal features relying on current time step only, and several acceleration techniques including backward phase skipping and layerwise FreezeOut are proposed to accelerate the training. Experimental results show that the proposed learning algorithm achieved the classification accuracies of 98.40% and 95.83% on the dynamic neuromorphic data sets MNIST-DVS and DVS-Gestures, respectively, and of 99.58% and 95.97% on static vision data sets MNIST and SVHN, respectively, which are comparable to the existing state-of-the-art results. The training speed was accelerated by up to 49.5% on MNIST and 36.6% on DVS-Gestures with the proposed acceleration techniques while maintaining the same level of accuracy.
更多查看译文
关键词
Backward phase skipping,layerwise FreezeOut (LFO),leaky integrate-and-fire (LIF) neuron model,spiking neural networks (SNNs),surrogate gradient
AI 理解论文
溯源树
样例
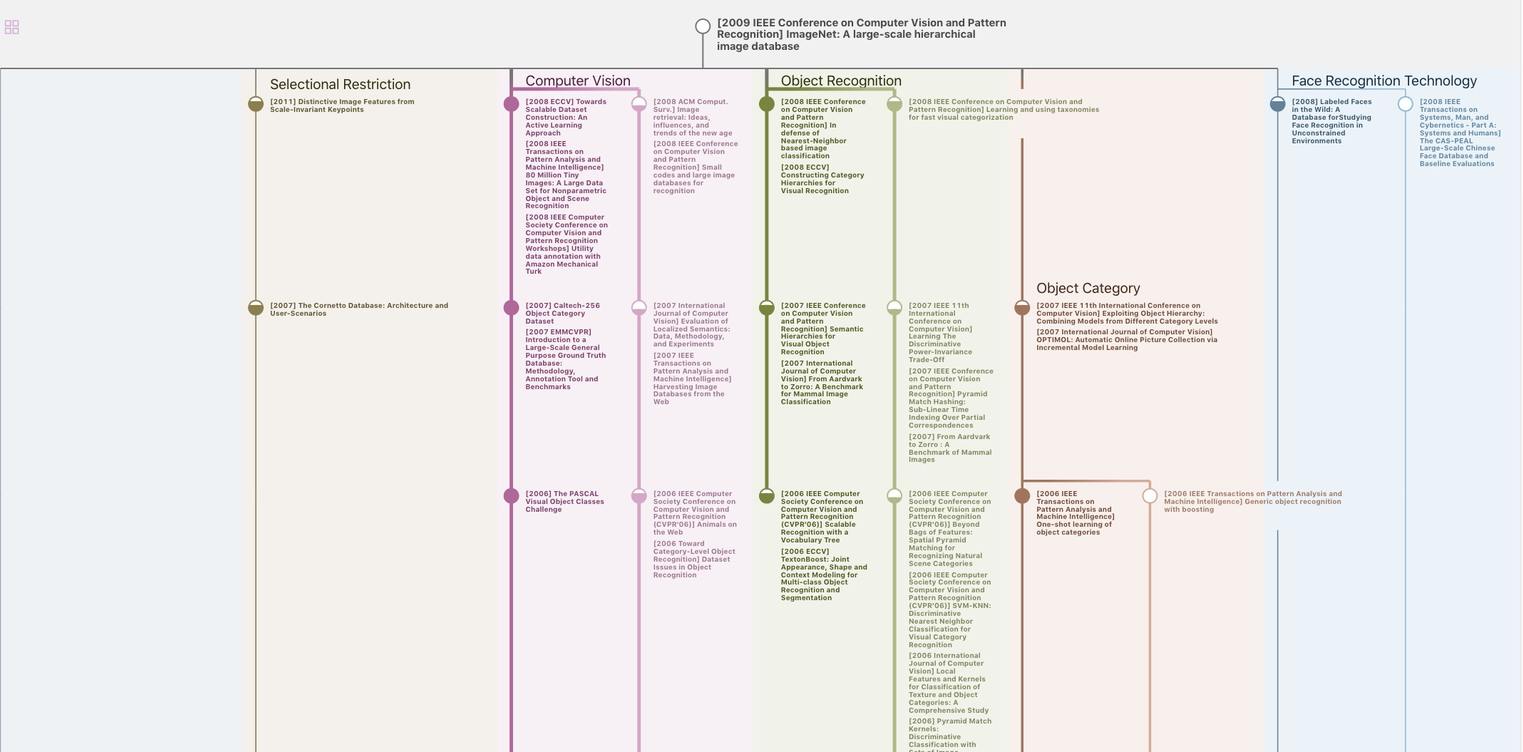
生成溯源树,研究论文发展脉络
Chat Paper
正在生成论文摘要