Lightweight boundary refinement module based on point supervision for semantic segmentation
Image and Vision Computing(2021)
Abstract
An effective semantic segmentation approach should contain fine object boundaries and continuous regions. However, recent mask-based segmentation cannot extract boundary features well on the coarse prediction, which causes obvious problems of blurry edges. Although several segmentation methods embed boundary detection branch to calculate contours directly, this type of architecture will lead to the increased computational complexity and miss the edge detailed information such as inter-class distinction. In order to obtain fine boundaries, we present a lightweight boundary refinement module with point supervision named BRPS to improve the boundary quality for the segmentation result generated by various existing segmentation models. Firstly, a direction field is learned to complete the initial feature rectification, which is defined as pointing away from the nearest object boundary to each pixel, where the weighted Euclidean and Cosine distance function is used as the loss between predicted boundary pixels and Ground-truth labels. Then, point-based supervised learning is performed at uncertain and certain locations including random distribution and key feature points based on a new point convolutional operation to output final crisp object boundaries. Finally, we verify that our BRPS module can effectively reduce the prediction errors for segmentation results generated from various state-of-the-art models such as DeepLabv3 and HRNet on the Pascal VOC2012, NYUD v2 datasets, Cityscapes and BDD100K datasets.
MoreTranslated text
Key words
Semantic segmentation,Boundary refinement,Point supervision,Point convolution,Direction field
AI Read Science
Must-Reading Tree
Example
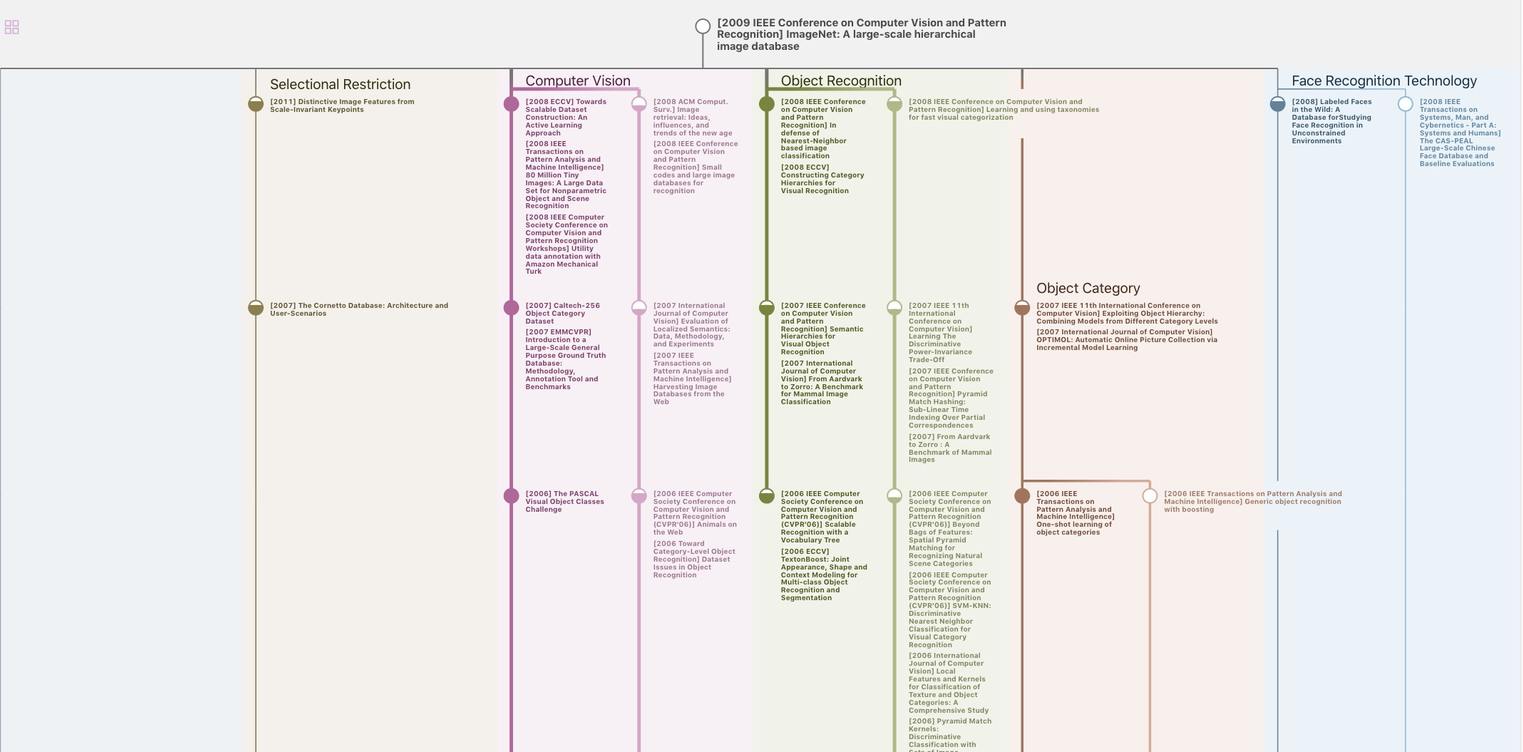
Generate MRT to find the research sequence of this paper
Chat Paper
Summary is being generated by the instructions you defined