Machine-learning-based dynamic-importance sampling for adaptive multiscale simulations
NATURE MACHINE INTELLIGENCE(2021)
摘要
Multiscale simulations are a well-accepted way to bridge the length and time scales required for scientific studies with the solution accuracy achievable through available computational resources. Traditional approaches either solve a coarse model with selective refinement or coerce a detailed model into faster sampling, both of which have limitations. Here, we present a paradigm of adaptive, multiscale simulations that couple different scales using a dynamic-importance sampling approach. Our method uses machine learning to dynamically and exhaustively sample the phase space explored by a macro model using microscale simulations and enables an automatic feedback from the micro to the macro scale, leading to a self-healing multiscale simulation. As a result, our approach delivers macro length and time scales, but with the effective precision of the micro scale. Our approach is arbitrarily scalable as well as transferable to many different types of simulations. Our method made possible a multiscale scientific campaign of unprecedented scale to understand the interactions of RAS proteins with a plasma membrane in the context of cancer research running over several days on Sierra, which is currently the second-most-powerful supercomputer in the world.
更多查看译文
关键词
Computational biology and bioinformatics,Computational science,Machine learning,Engineering,general
AI 理解论文
溯源树
样例
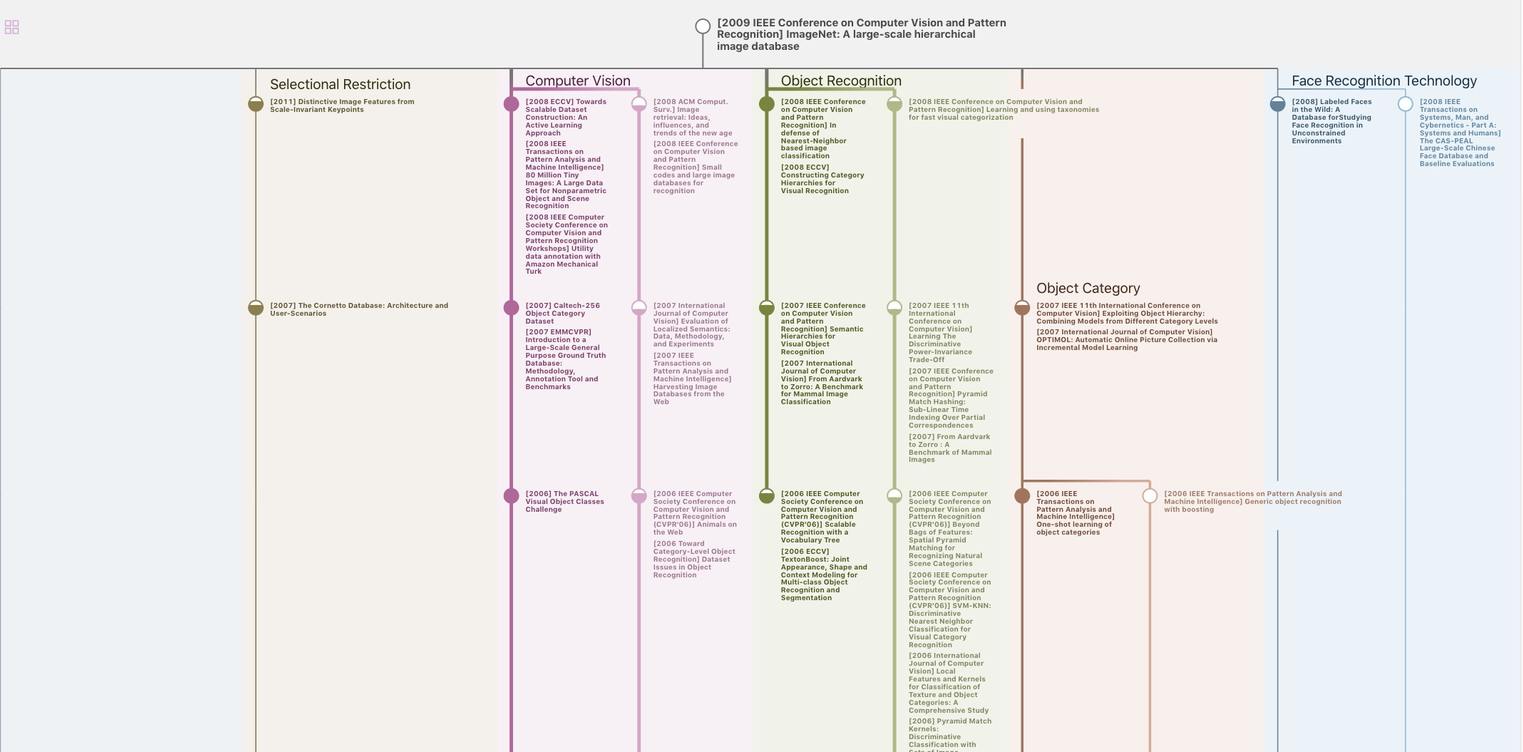
生成溯源树,研究论文发展脉络
Chat Paper
正在生成论文摘要