Discriminative Invariant Alignment for Unsupervised Domain Adaptation
IEEE TRANSACTIONS ON MULTIMEDIA(2022)
摘要
As one of the most prevalent branches of transfer learning, domain adaptation is dedicated to generalizing the knowledge of a source domain to a target domain to perform machine learning tasks. In domain adaptation, the key strategy is to overcome the shift between different domains and learn shared features with domain invariance. However, most existing methods focus on extracting the common features of the source and target domains, and do not consider the shift problem of class center in the target domain caused by this process. Specifically, when we align the domain distributions, we often ignore the inherent feature attributes of the data, or under the guidance of false pseudo-labels, cause the target domain data to be far away from the class center after projection. This is not conducive to classification task. To address these problems, in this study, we propose a novel domain adaptation method, referred to as discriminative invariant alignment (DIA), for image representation. DIA enriches the knowledge matrix by combining the class discriminative information of the source domain and local data structure information of the target domain into a new framework. By introducing the maximum margin criterion of the source domain, the classification boundaries are expanded. To verify the performance of the proposed method, we compared DIA with several state-of-the-art methods on five benchmark databases. The experimental results show that DIA is superior to the state-of-the-art methods.
更多查看译文
关键词
Task analysis, Feature extraction, Manifolds, Degradation, Neural networks, Kernel, Data structures, Domain adaptation, subspace learning, maximum margin criterion
AI 理解论文
溯源树
样例
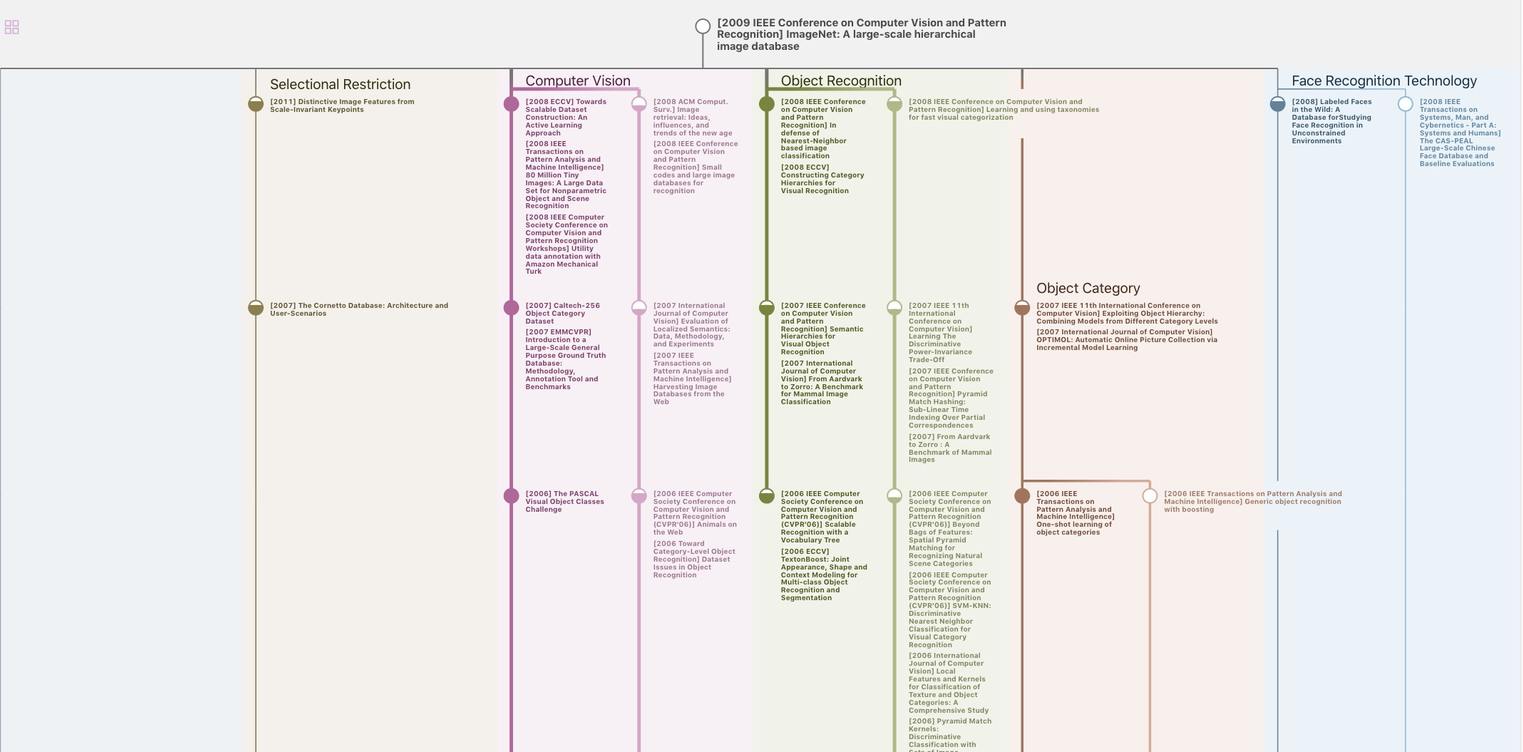
生成溯源树,研究论文发展脉络
Chat Paper
正在生成论文摘要