A New Metric for Characterizing Dynamic Redundancy of Dense Brain Chronnectome and Its Application to Early Detection of Alzheimer’s Disease
Lecture Notes in Computer Science(2020)
摘要
Graph theory has been used extensively to investigate information exchange efficiency among brain regions represented as graph nodes. In this work, we propose a new metric to measure how the brain network is robust or resilient to any attack on its nodes and edges. The metric measures redundancy in the sense that it calculates the minimum number of independent, not necessarily shortest, paths between every pair of nodes. We adopt this metric for characterizing (i) the redundancy of time-varying brain networks, i.e., chronnectomes, computed along the progression of Alzheimer’s disease (AD), including early mild cognitive impairment (EMCI), and (ii) changes in progressive MCI compared to stable MCI by calculating the probabilities of having at least 2 (or 3) independent paths between every pair of brain regions in a short period of time. Finally, we design a learning-based early AD detection framework, coined “REdundancy Analysis of Dynamic functional connectivity for Disease Diagnosis (READ\(^3\))”, and show its superiority over other AD early detection methods. With the ability to measure dynamic resilience and robustness of brain networks, the metric is complementary to the commonly used “cost-efficiency” in brain network analysis.
更多查看译文
关键词
Graph theory,Complex brain networks,Disease diagnosis
AI 理解论文
溯源树
样例
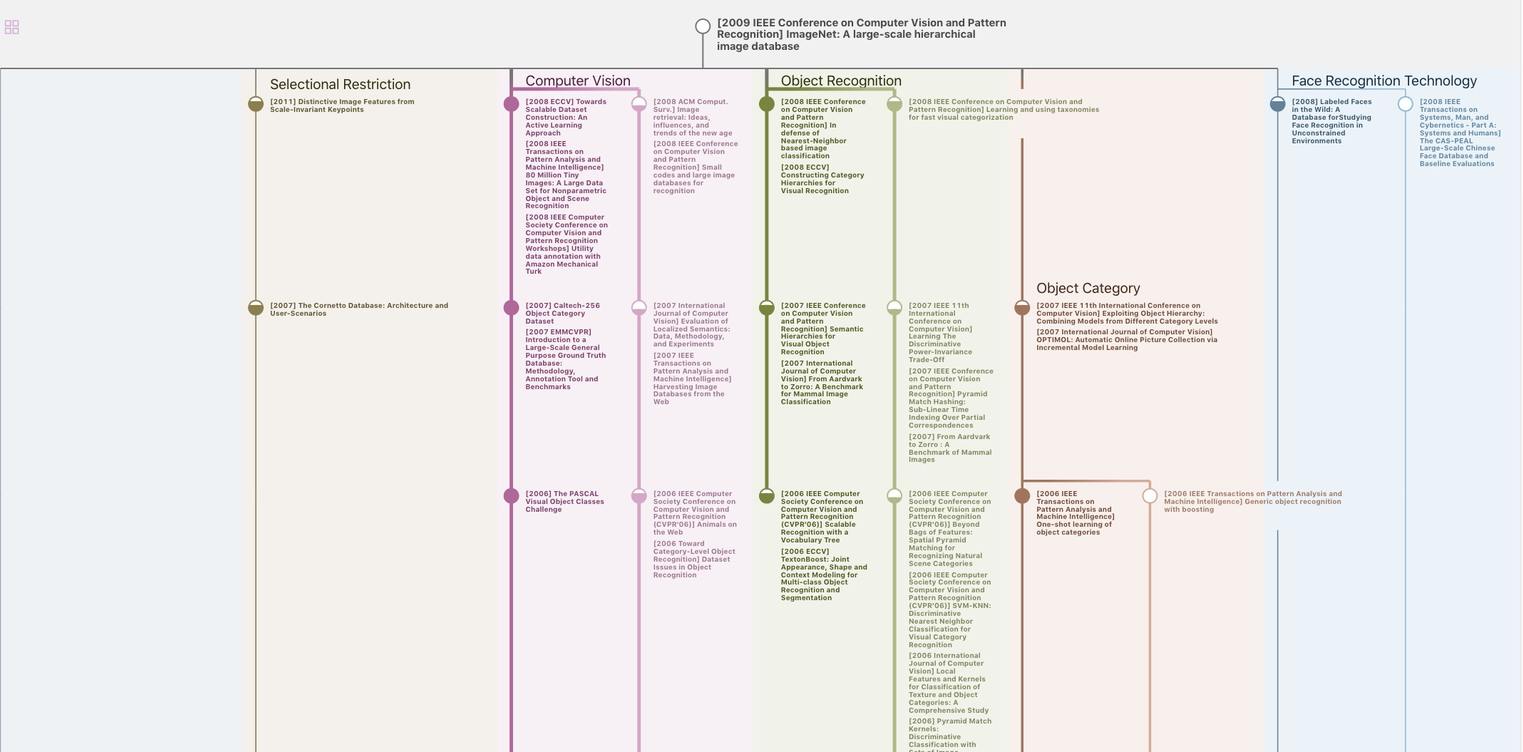
生成溯源树,研究论文发展脉络
Chat Paper
正在生成论文摘要