Statistical And Machine Learning Link Selection Methods For Brain Functional Networks: Review And Comparison
BRAIN SCIENCES(2021)
摘要
Network-based representations have introduced a revolution in neuroscience, expanding the understanding of the brain from the activity of individual regions to the interactions between them. This augmented network view comes at the cost of high dimensionality, which hinders both our capacity of deciphering the main mechanisms behind pathologies, and the significance of any statistical and/or machine learning task used in processing this data. A link selection method, allowing to remove irrelevant connections in a given scenario, is an obvious solution that provides improved utilization of these network representations. In this contribution we review a large set of statistical and machine learning link selection methods and evaluate them on real brain functional networks. Results indicate that most methods perform in a qualitatively similar way, with NBS (Network Based Statistics) winning in terms of quantity of retained information, AnovaNet in terms of stability and ExT (Extra Trees) in terms of lower computational cost. While machine learning methods are conceptually more complex than statistical ones, they do not yield a clear advantage. At the same time, the high heterogeneity in the set of links retained by each method suggests that they are offering complementary views to the data. The implications of these results in neuroscience tasks are finally discussed.
更多查看译文
关键词
brain functional networks, link selection, statistics, machine learning
AI 理解论文
溯源树
样例
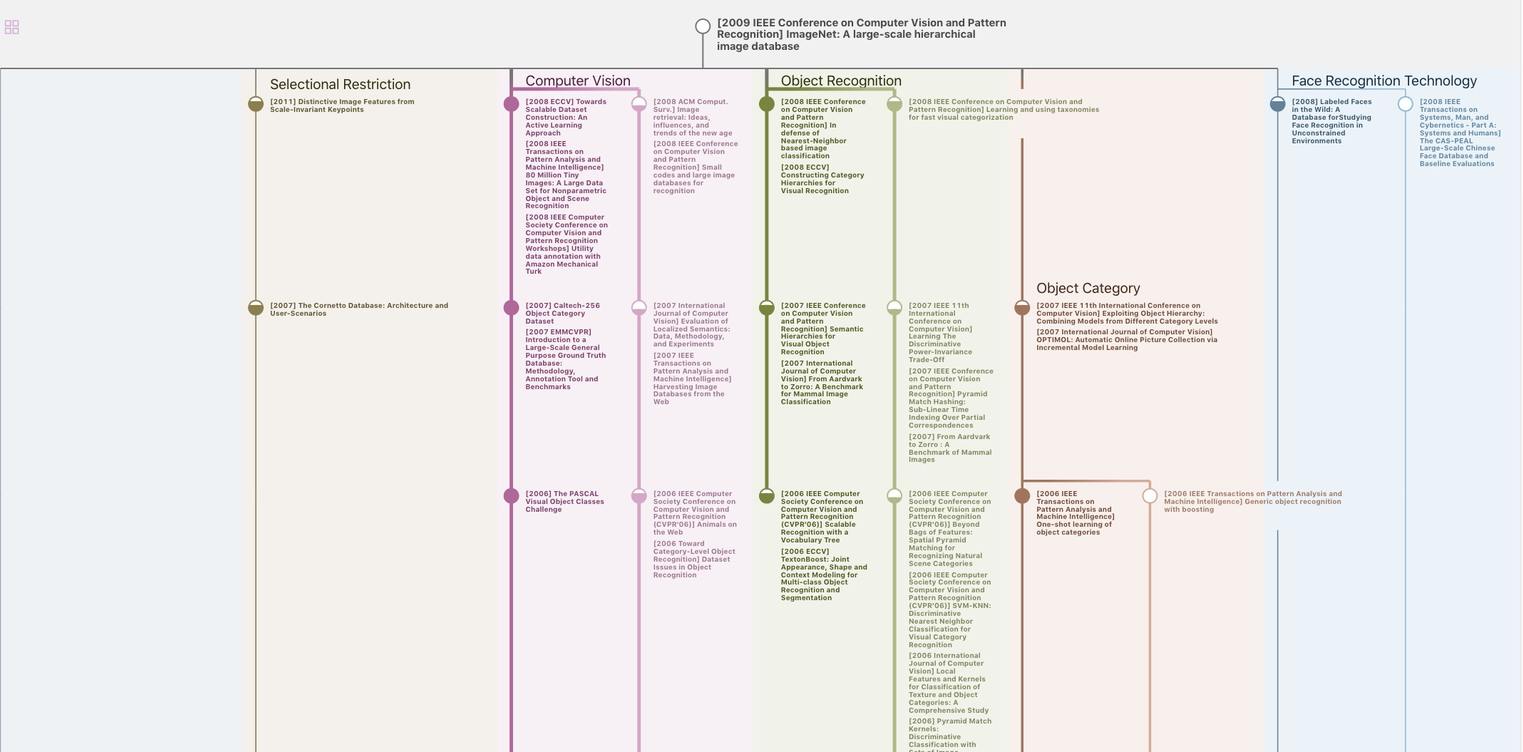
生成溯源树,研究论文发展脉络
Chat Paper
正在生成论文摘要