Error Bound Of Mode-Based Additive Models
ENTROPY(2021)
摘要
Due to their flexibility and interpretability, additive models are powerful tools for high-dimensional mean regression and variable selection. However, the least-squares loss-based mean regression models suffer from sensitivity to non-Gaussian noises, and there is also a need to improve the model's robustness. This paper considers the estimation and variable selection via modal regression in reproducing kernel Hilbert spaces (RKHSs). Based on the mode-induced metric and two-fold Lasso-type regularizer, we proposed a sparse modal regression algorithm and gave the excess generalization error. The experimental results demonstrated the effectiveness of the proposed model.
更多查看译文
关键词
modal regression, additive models, reproducing kernel Hilbert spaces, error bound
AI 理解论文
溯源树
样例
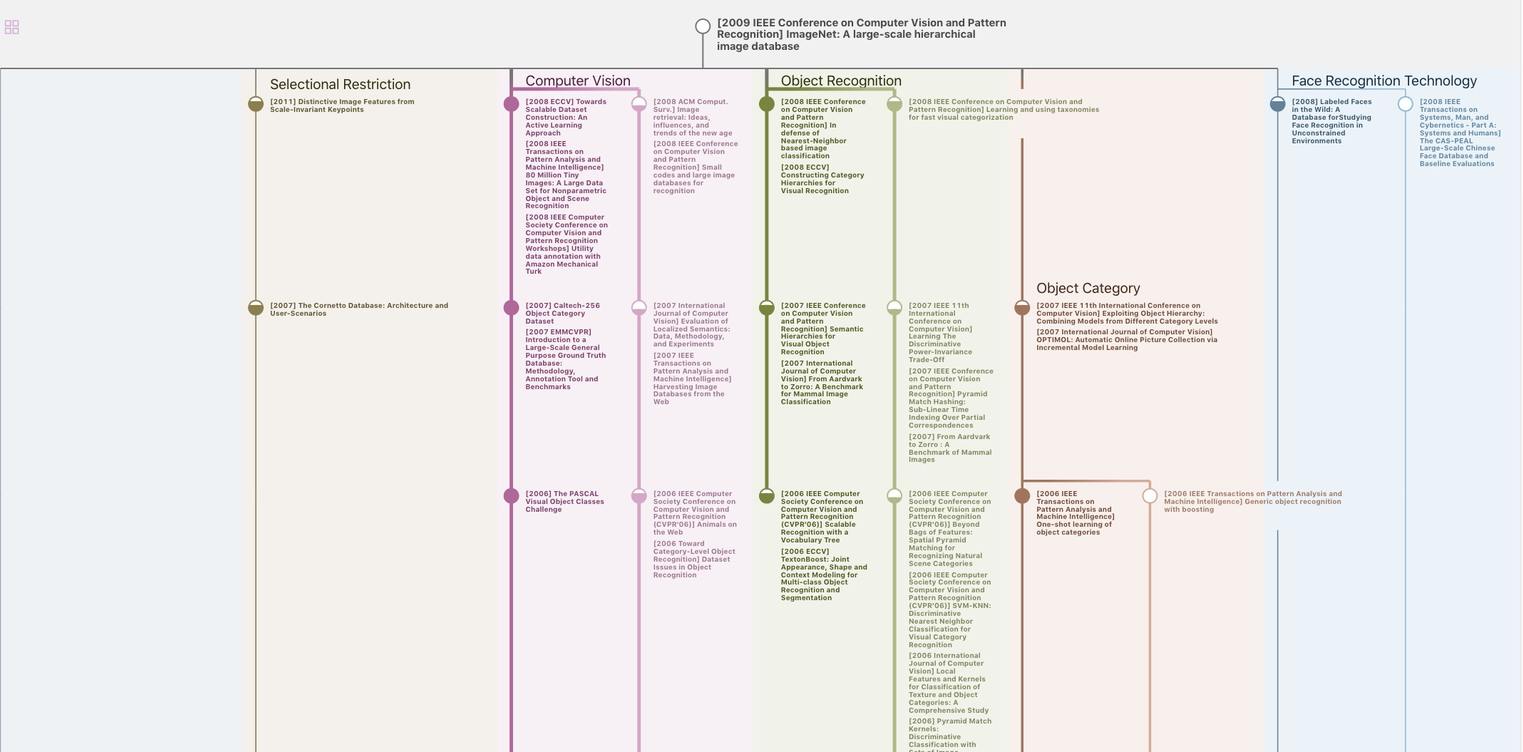
生成溯源树,研究论文发展脉络
Chat Paper
正在生成论文摘要