Coastal Wetland Classification with GF-3 Polarimetric SAR Imagery by Using Object-Oriented Random Forest Algorithm.
Sensors (Basel, Switzerland)(2021)
摘要
When the use of optical images is not practical due to cloud cover, Synthetic Aperture Radar (SAR) imagery is a preferred alternative for monitoring coastal wetlands because it is unaffected by weather conditions. Polarimetric SAR (PolSAR) enables the detection of different backscattering mechanisms and thus has potential applications in land cover classification. Gaofen-3 (GF-3) is the first Chinese civilian satellite with multi-polarized C-band SAR imaging capability. Coastal wetland classification with GF-3 polarimetric SAR imagery has attracted increased attention in recent years, but it remains challenging. The aim of this study was to classify land cover in coastal wetlands using an object-oriented random forest algorithm on the basis of GF-3 polarimetric SAR imagery. First, a set of 16 commonly used SAR features was extracted. Second, the importance of each SAR feature was calculated, and the optimal polarimetric features were selected for wetland classification by combining random forest (RF) with sequential backward selection (SBS). Finally, the proposed algorithm was utilized to classify different land cover types in the Yancheng Coastal Wetlands. The results show that the most important parameters for wetland classification in this study were Shannon entropy, Span and orientation randomness, combined with features derived from Yamaguchi decomposition, namely, volume scattering, double scattering, surface scattering and helix scattering. When the object-oriented RF classification approach was used with the optimal feature combination, different land cover types in the study area were classified, with an overall accuracy of up to 92%.
更多查看译文
AI 理解论文
溯源树
样例
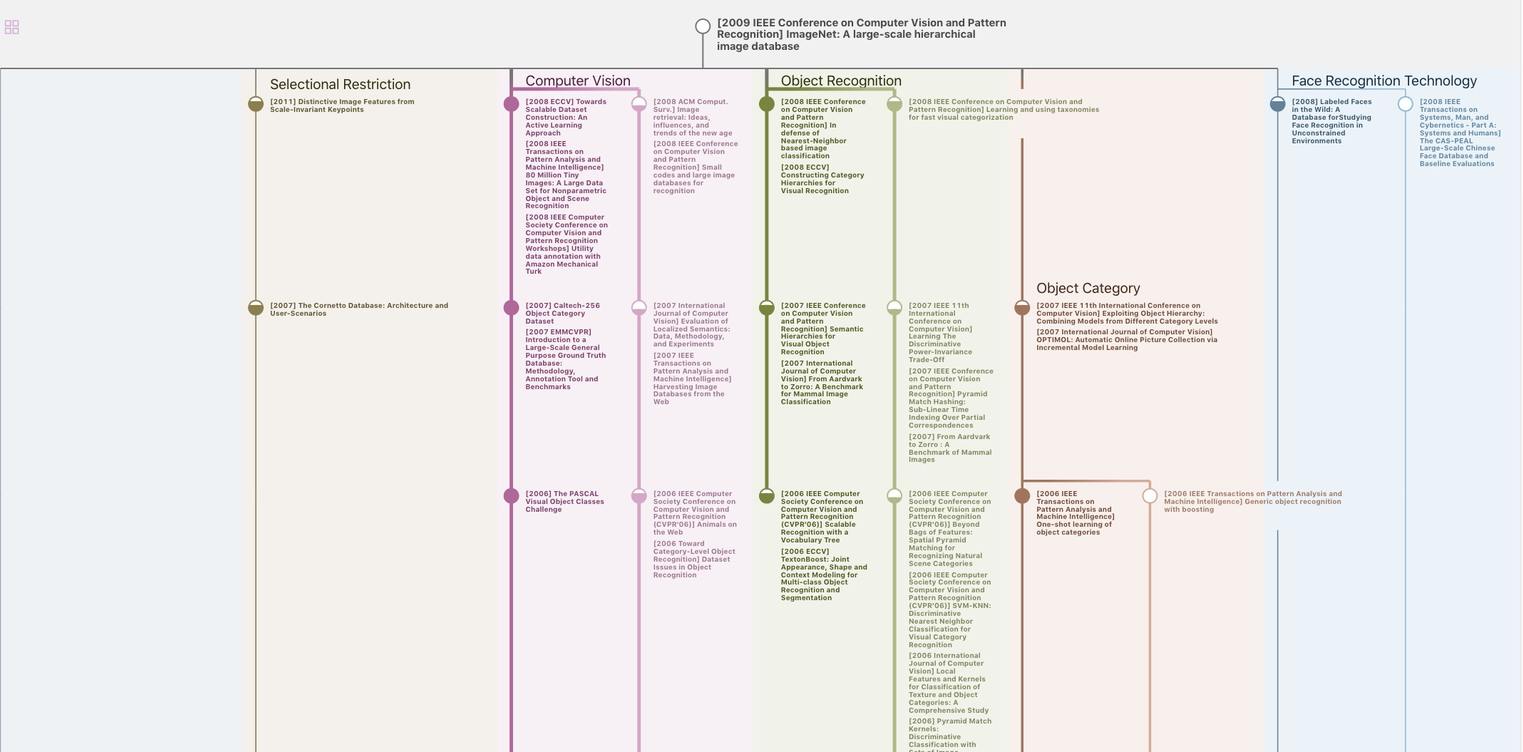
生成溯源树,研究论文发展脉络
Chat Paper
正在生成论文摘要