Dynamic Functional Connectivity For The Classification Of Multiple Sclerosis Phenotype: A Hidden Markov Model Approach
2021 IEEE 18TH INTERNATIONAL SYMPOSIUM ON BIOMEDICAL IMAGING (ISBI)(2021)
摘要
We present a pipeline for the classification of subjects according to multiple sclerosis phenotype. The approach is based on a hidden Markov model built on dynamic functional connectivity. More in detail, a sequence of correlation matrices is built from the fMRI time slices, then projected on the tangent Euclidean space. The number of considered dimensions is reduced through PCA, then dominant set clustering is applied to group similar correlation matrices in a limited number of ”mental” states, which are then used as the hidden states of the Markov model. Subjects in the test set are then classified according to the likelihood of their sequence of observations with the obtained class-wise Markov models. We demonstrate that our approach is capable of discriminating multiple sclerosis phenotypes and that the persistence of some of the identified states is a possible neurohpyisiological marker of disease severity.
更多查看译文
关键词
Multiple sclerosis, fMRI, dominant set clustering, dynamic functional connectivity, hidden Markov model
AI 理解论文
溯源树
样例
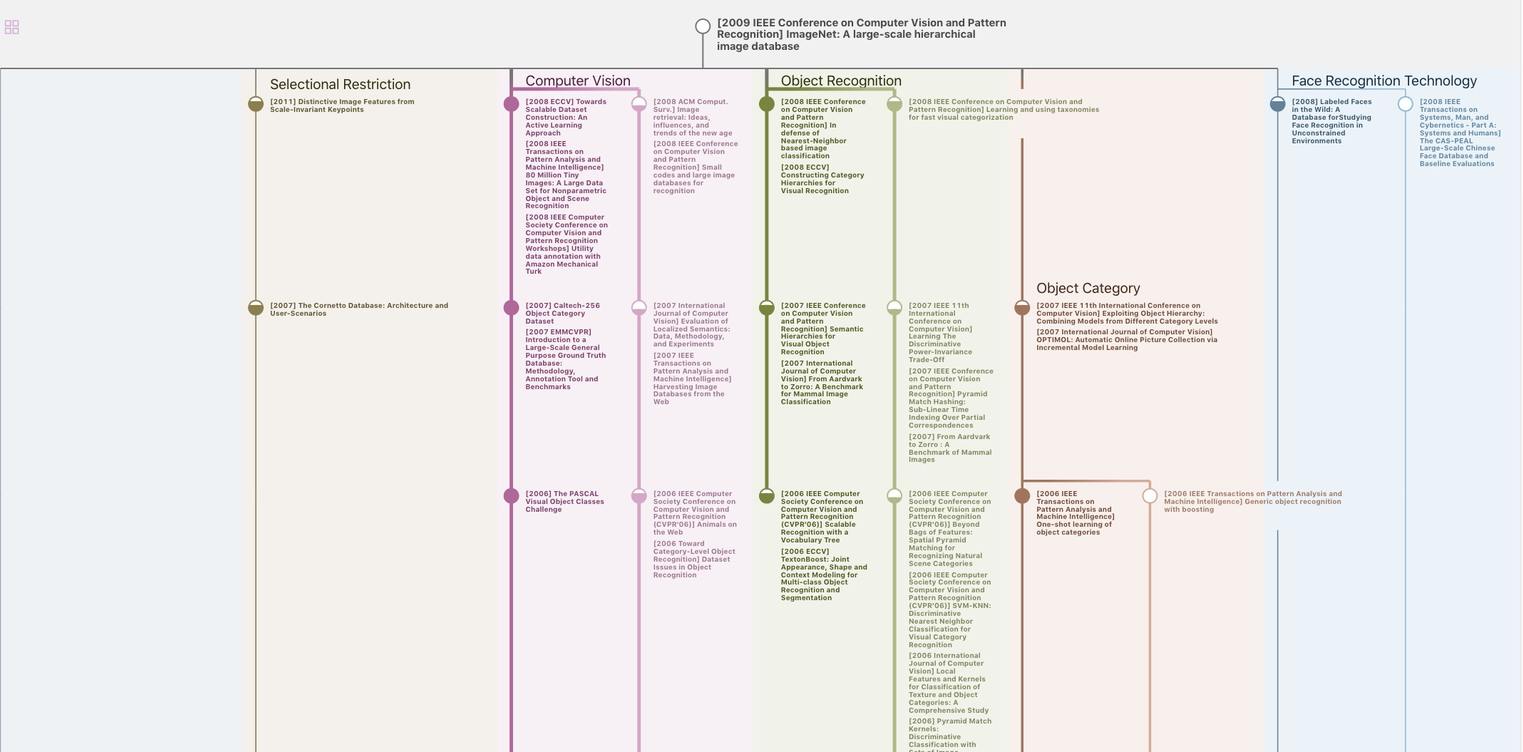
生成溯源树,研究论文发展脉络
Chat Paper
正在生成论文摘要