A COVID-19 PATIENT SEVERITY STRATIFICATION USING A 3D CONVOLUTIONAL STRATEGY ON CT-SCANS
2021 IEEE 18TH INTERNATIONAL SYMPOSIUM ON BIOMEDICAL IMAGING (ISBI)(2021)
摘要
This work introduces a 3D deep learning methodology to stratify patients according to the severity of lung infection caused by COVID-19 disease on computerized tomography images (CT). A set of volumetric attention maps were also obtained to explain the results and support the diagnostic tasks. The validation of the approach was carried out on a dataset composed of 350 patients, diagnosed by the RT-PCR assay either as negative (control - 175) or positive (COVID-19 - 175). Additionally, the patients were graded (0-25) by two expert radiologists according to the extent of lobar involvement. These gradings were used to define 5 COVID-19 severity categories. The model yields an average 60% accuracy for the multi-severity classification task. Additionally, a set of Mann Whitney U significance tests were conducted to compare the severity groups. Results show that patients in different severity groups have significantly different severity scores (p < 0.01) for all the compared severity groups.
更多查看译文
关键词
COVID-19, severity, 3D-CNN networks, deep learning, tomography
AI 理解论文
溯源树
样例
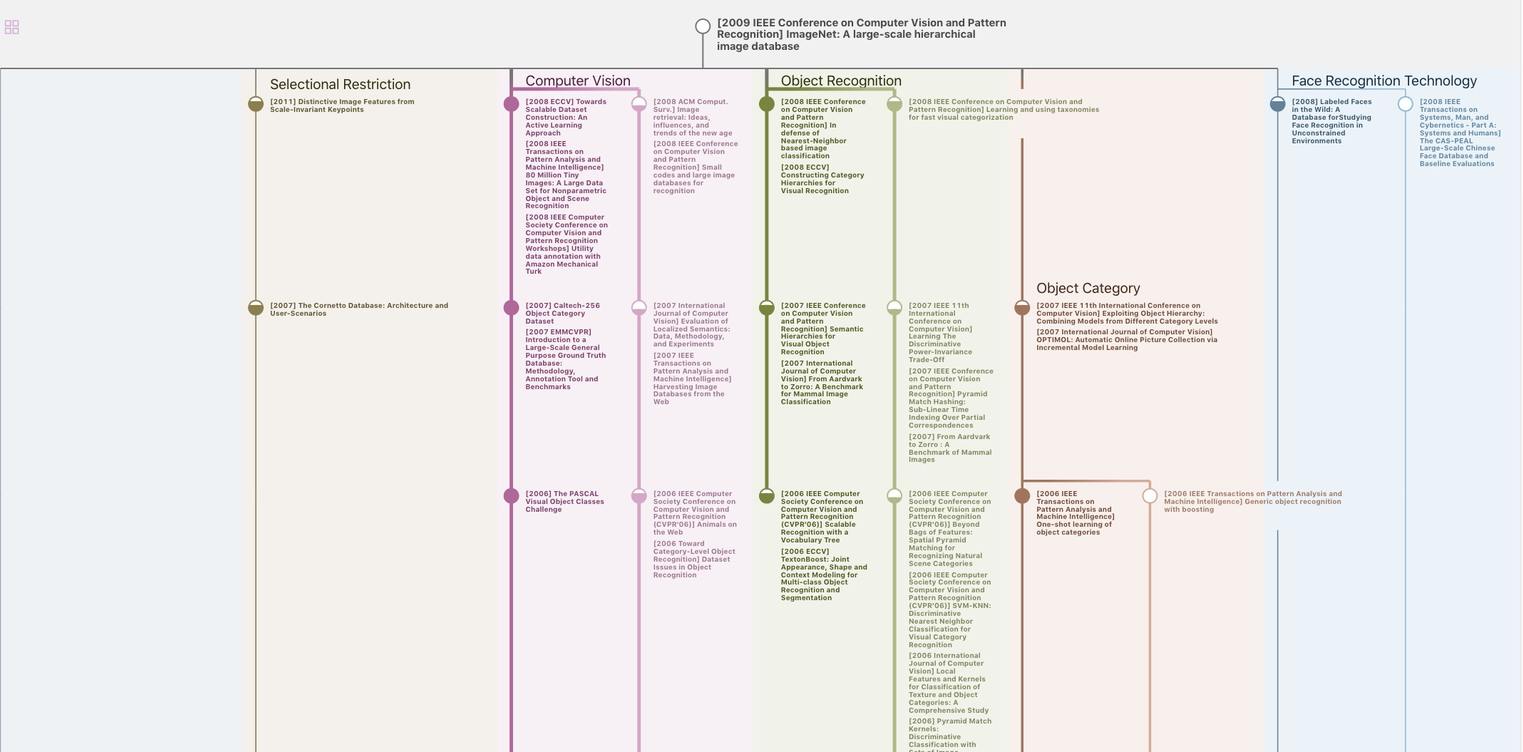
生成溯源树,研究论文发展脉络
Chat Paper
正在生成论文摘要